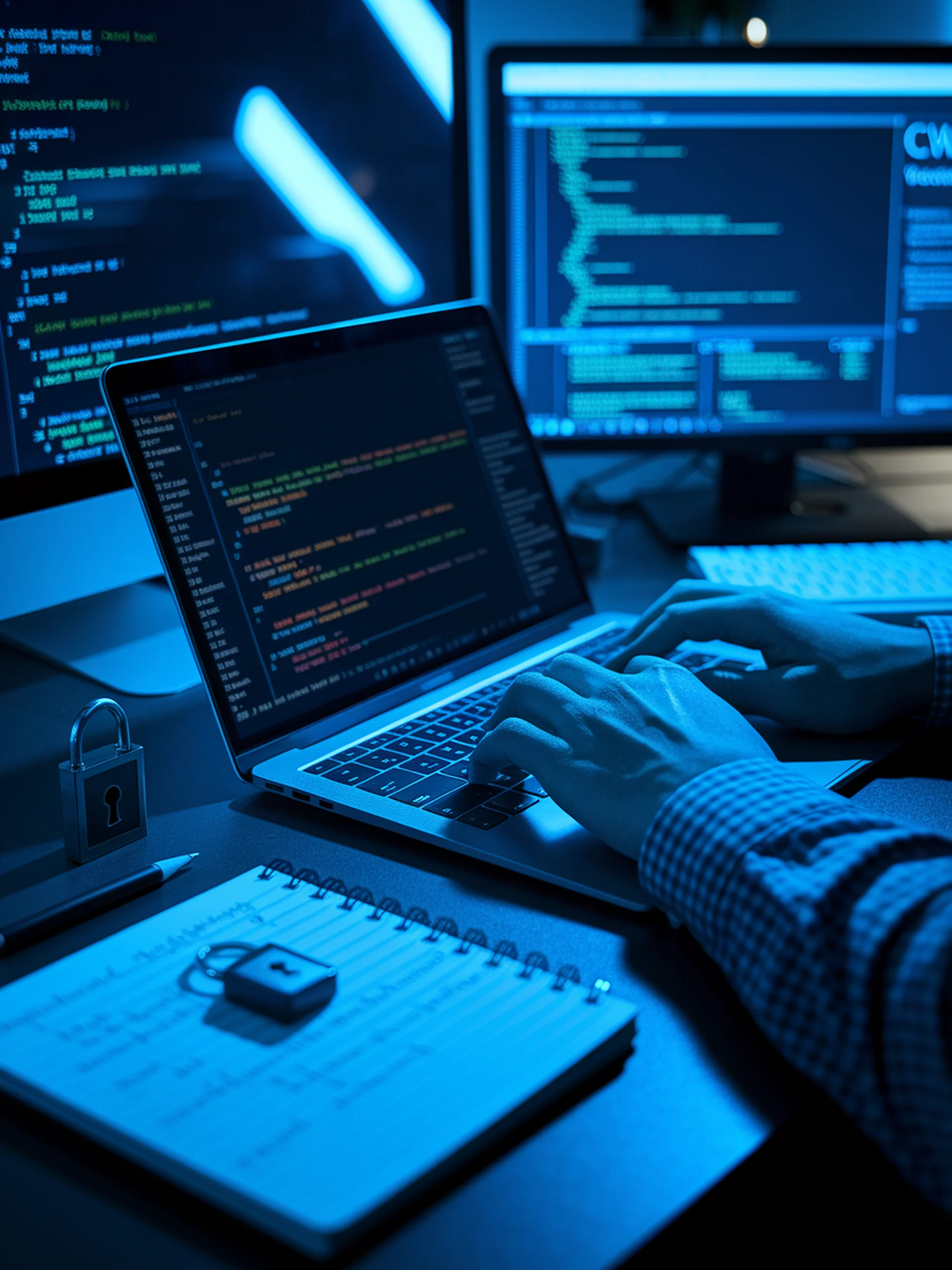
Securing Code Generation in LLMs
Proactive security alignment to prevent vulnerabilities in AI-generated code
This research introduces a novel approach to fortify code-generating LLMs with security awareness, addressing critical vulnerabilities before they enter production systems.
- Uses Common Weakness Enumerations (CWEs) as a framework to identify and mitigate security risks
- Implements a proactive security alignment methodology rather than relying solely on sparse vulnerable code examples
- Creates targeted training data to teach models to recognize and avoid generating insecure code patterns
- Demonstrates significant improvements in the security posture of code-generating LLMs
This research is crucial as insecure AI-generated code could introduce widespread vulnerabilities into software systems, potentially creating security risks at unprecedented scale. The proactive approach ensures security is embedded during model development rather than addressed reactively.
ProSec: Fortifying Code LLMs with Proactive Security Alignment