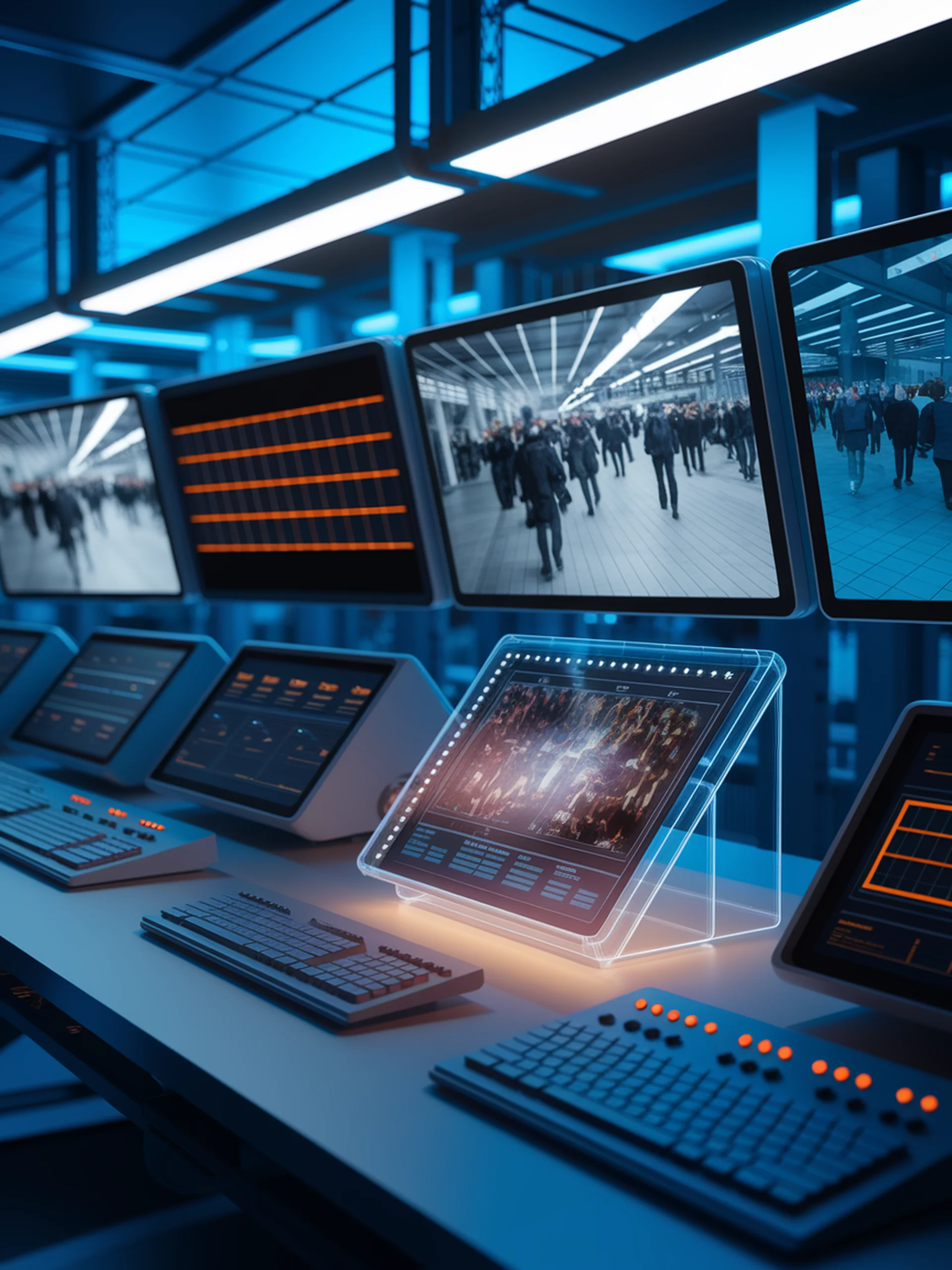
Spotting the Unusual: AI-Powered Anomaly Detection
A breakthrough benchmark for text-based detection of abnormal pedestrian behaviors
This research addresses critical gaps in security surveillance by developing the first large-scale benchmark focused on identifying abnormal human behaviors through natural language queries.
- Created a novel Pedestrian Anomaly Behavior (PAB) dataset with 148K images across 10K identities and 29 anomaly categories
- Developed a new Fine-Grained Representation Learning (FGRL) approach that improves anomaly detection accuracy by 5.5%
- Demonstrated superior performance in real-world scenarios where traditional person search fails to detect unusual activities
- Established a foundation for more effective security monitoring systems that can identify potential threats based on behavioral descriptions
This innovation enables security systems to move beyond simple person identification to detect specific anomalous behaviors that may indicate security threats, significantly enhancing surveillance capabilities in crowded public spaces.
Beyond Walking: A Large-Scale Image-Text Benchmark for Text-based Person Anomaly Search