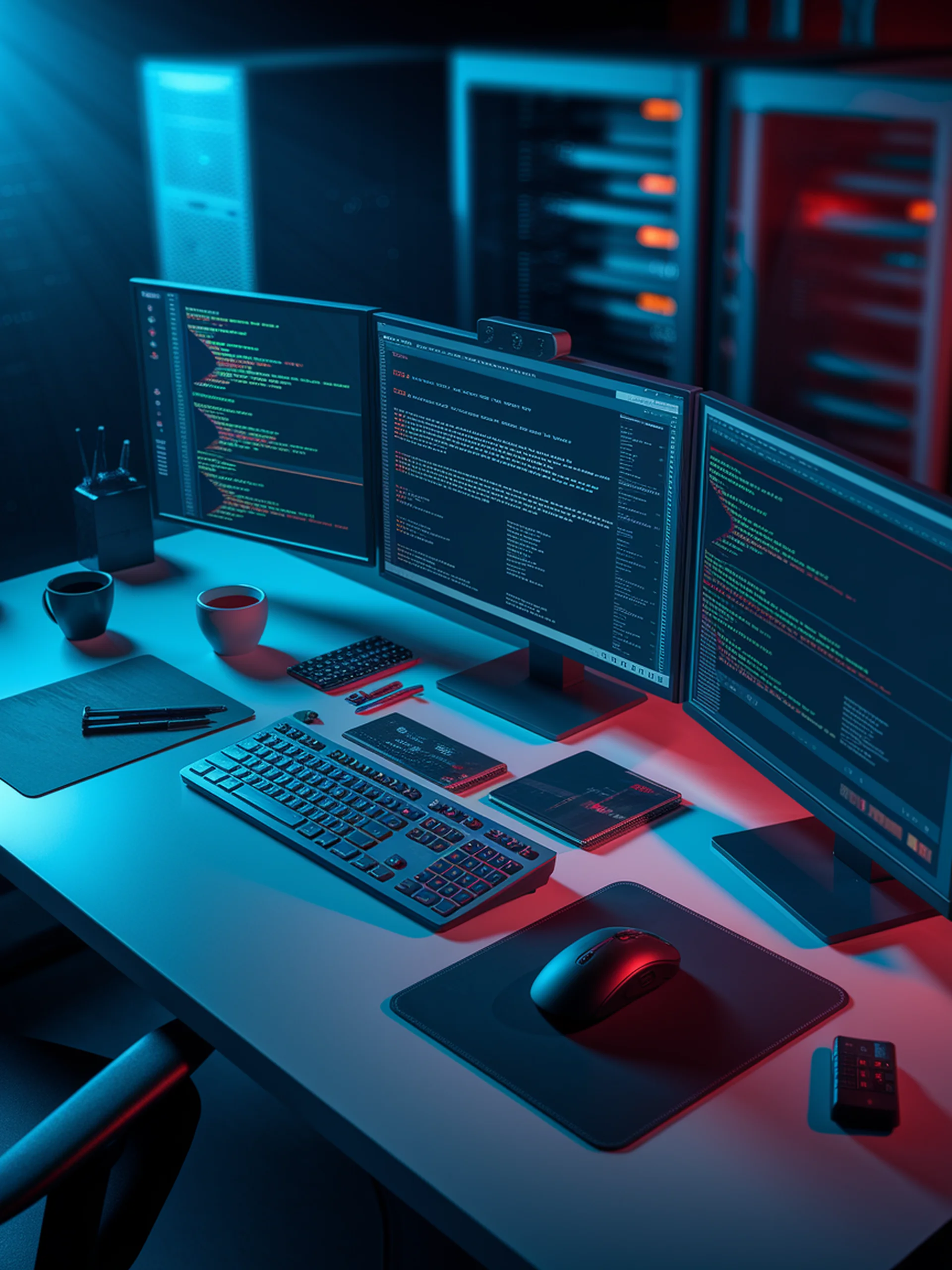
LLMs as Code Obfuscation Weapons?
Evaluating LLMs' ability to create malware-evading code obfuscations
This research systematically evaluates whether Large Language Models can generate obfuscated assembly code that could potentially evade malware detection systems.
Key findings:
- LLMs show concerning capability to generate various assembly code obfuscations without requiring source code
- Advanced LLMs (like GPT-4) outperform others in producing syntactically valid and semantically equivalent obfuscated code
- LLM-generated obfuscations demonstrated the ability to bypass some detection systems
- Researchers found prompting techniques that significantly enhanced obfuscation performance
Security Implications: This research highlights emerging cybersecurity threats as LLMs could lower the barrier for creating sophisticated malware that evades detection, requiring security professionals to develop new defensive strategies against AI-assisted attacks.