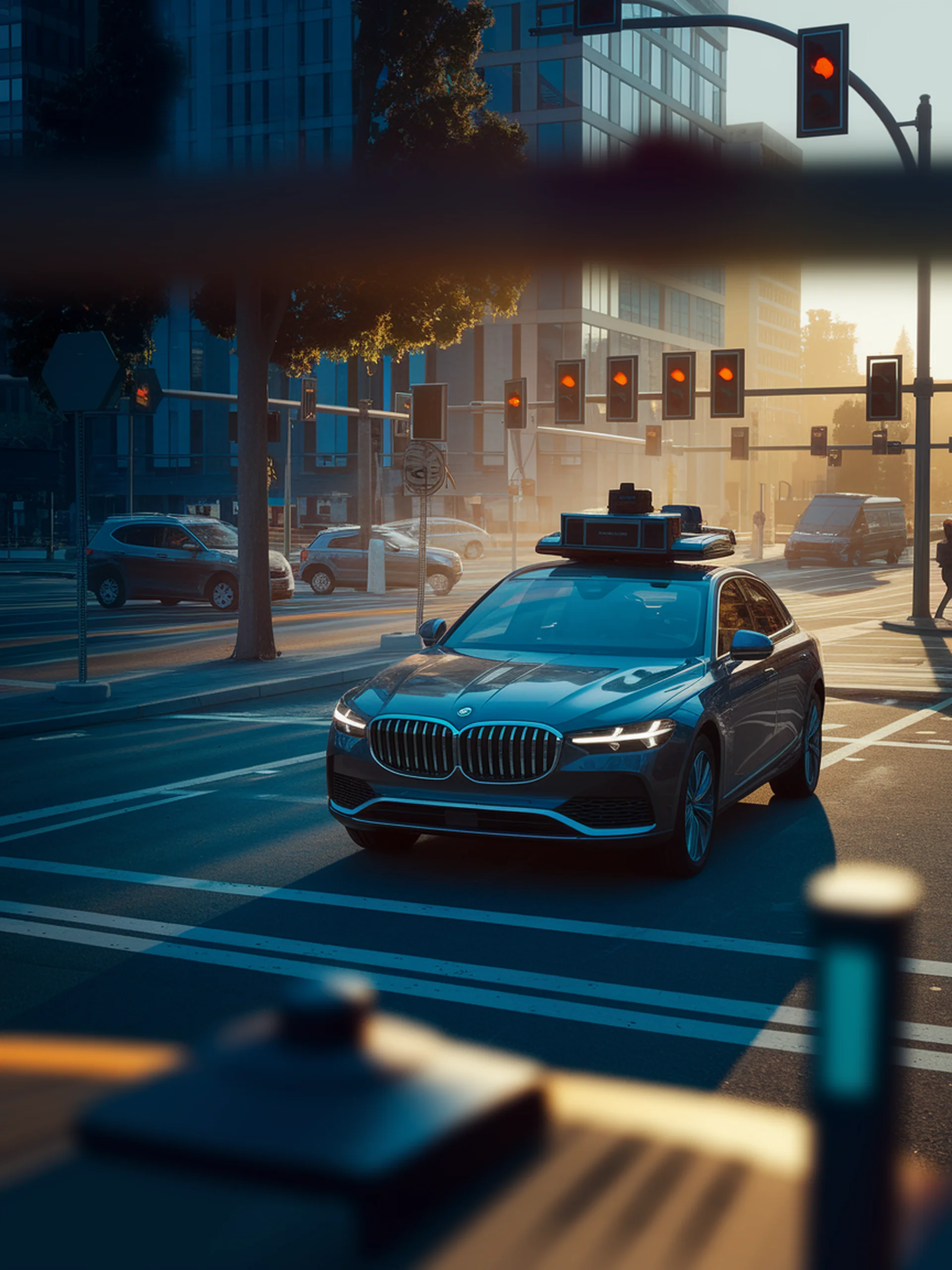
Visual Reasoning for Driving Safety
Enhancing VLMs with Retrieval-Based Interleaved Visual Chain-of-Thought
This research introduces a novel approach to improve visual reasoning in Vision-Language Models (VLMs) for complex driving scenarios, addressing critical gaps in current AI systems.
Key Innovations:
- Developed DrivingVQA, a specialized dataset derived from driving theory exams with expert explanations
- Implemented a retrieval-based interleaved Visual Chain-of-Thought method that significantly enhances reasoning capabilities
- Demonstrated improved performance in real-world driving scenarios through visual reasoning rather than relying solely on memorized knowledge
Security Implications: By improving visual reasoning for driving scenarios, this research directly enhances road safety potential for autonomous vehicles and driver assistance systems, addressing a critical transportation security challenge.
Retrieval-Based Interleaved Visual Chain-of-Thought in Real-World Driving Scenarios