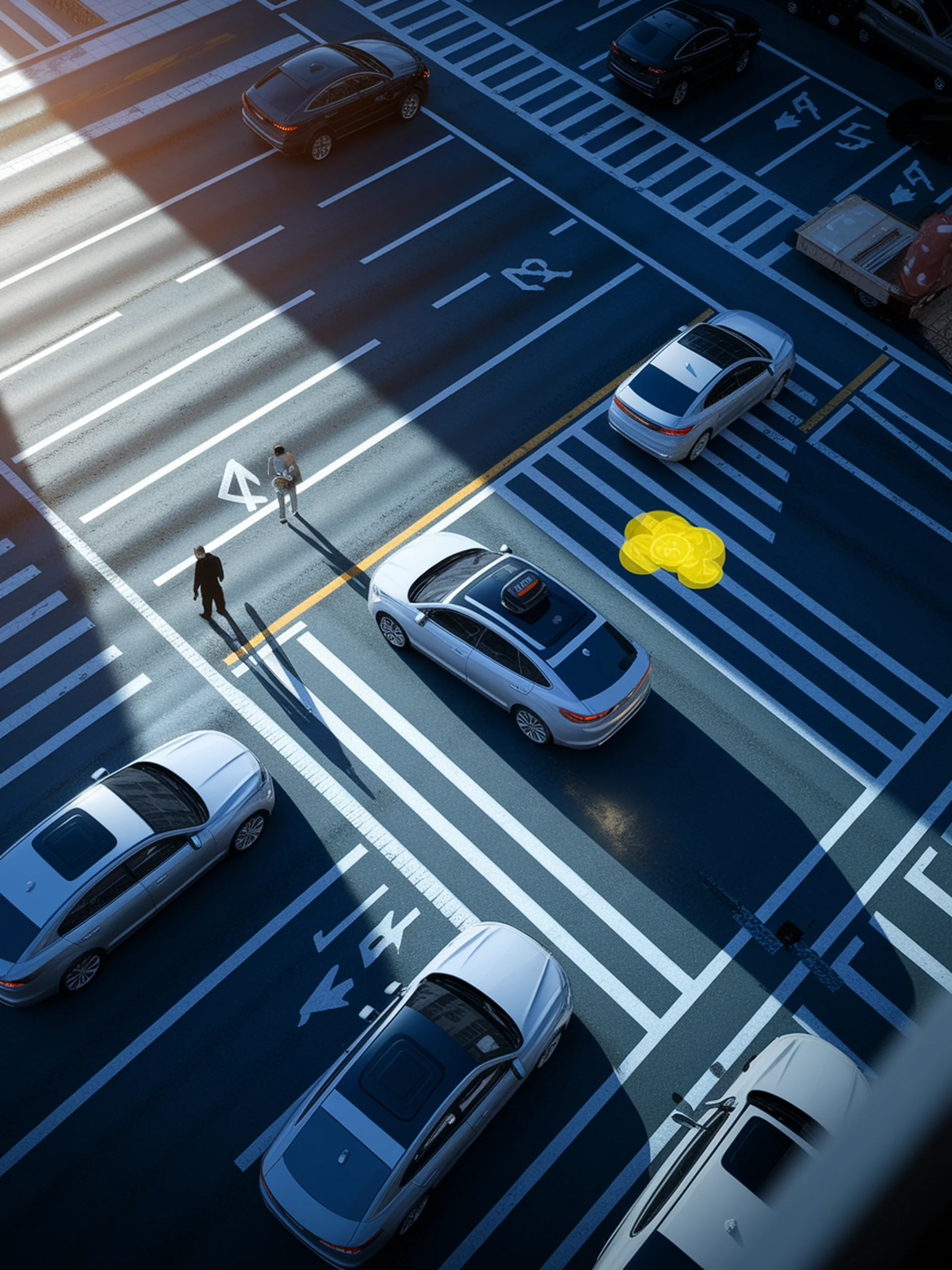
Smarter Adversarial Testing for Self-Driving Cars
Using LLMs to Identify and Generate Critical Safety Scenarios
This research introduces LLM-attacker, a novel framework that leverages large language models to enhance the effectiveness of adversarial testing for autonomous driving systems.
- Addresses the challenge of identifying which traffic participants to manipulate in testing scenarios
- Uses LLMs to prioritize potential adversarial agents based on context awareness
- Demonstrates superior performance in finding safety-critical edge cases compared to traditional methods
- Creates more realistic and diverse testing scenarios that better represent real-world risks
This innovation matters for engineering safer autonomous vehicles by significantly improving how we test their responses to dangerous situations, helping identify vulnerabilities before deployment.