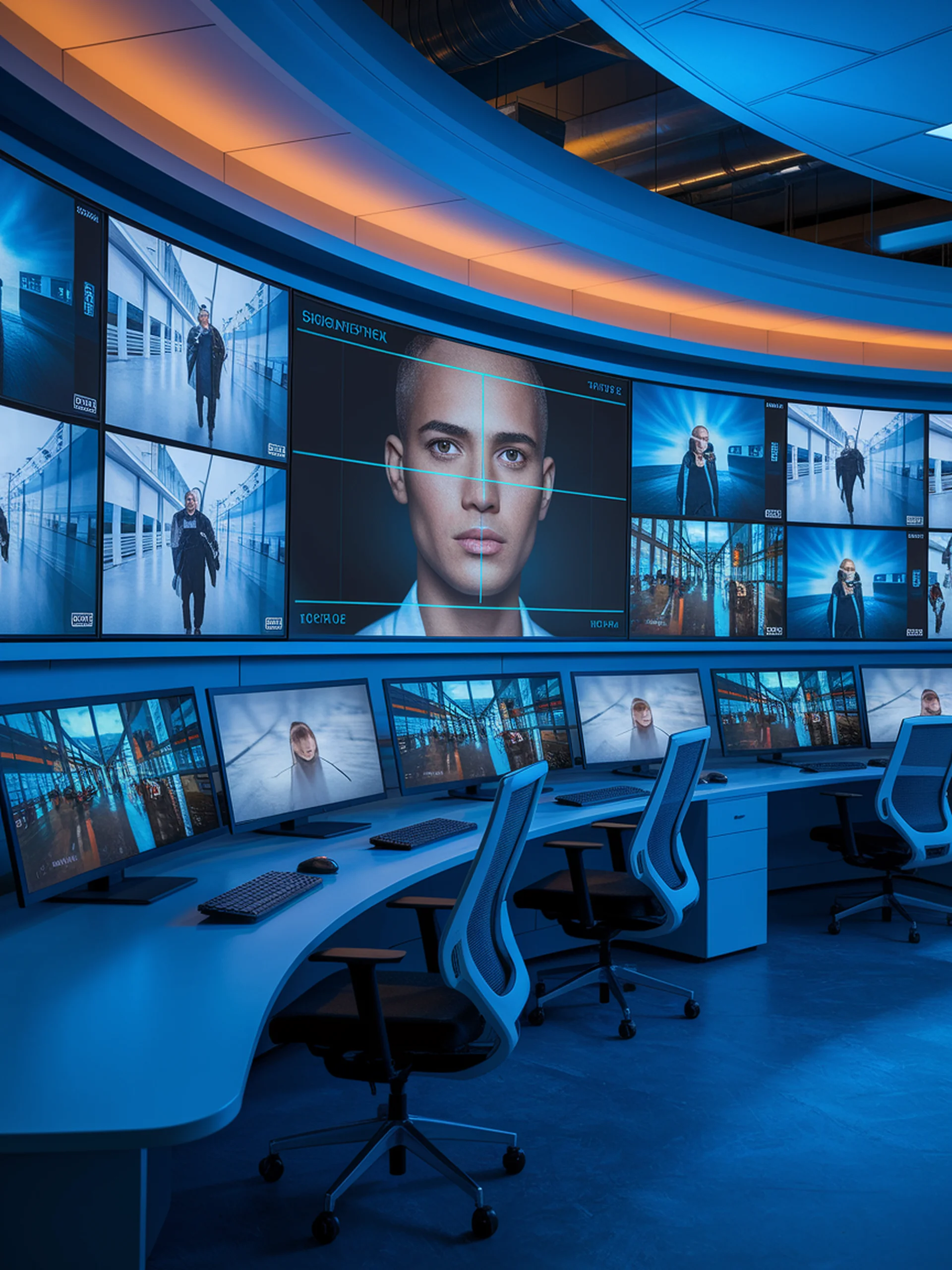
Next-Gen Person Re-Identification
Leveraging CLIP to Enhance Security Systems
This research adapts CLIP (Contrastive Language-Image Pretraining) to create more robust person re-identification systems with improved cross-domain capabilities.
- Harnesses vision-language models to achieve fine-grained and domain-invariant representations
- Addresses key challenges in adapting CLIP for person re-identification tasks
- Enhances discriminative ability while maintaining performance across different environments
- Delivers significant improvements for security and surveillance systems
This advancement enables more reliable tracking of individuals across multiple camera views, directly strengthening security infrastructure while reducing false identifications.
CILP-FGDI: Exploiting Vision-Language Model for Generalizable Person Re-Identification