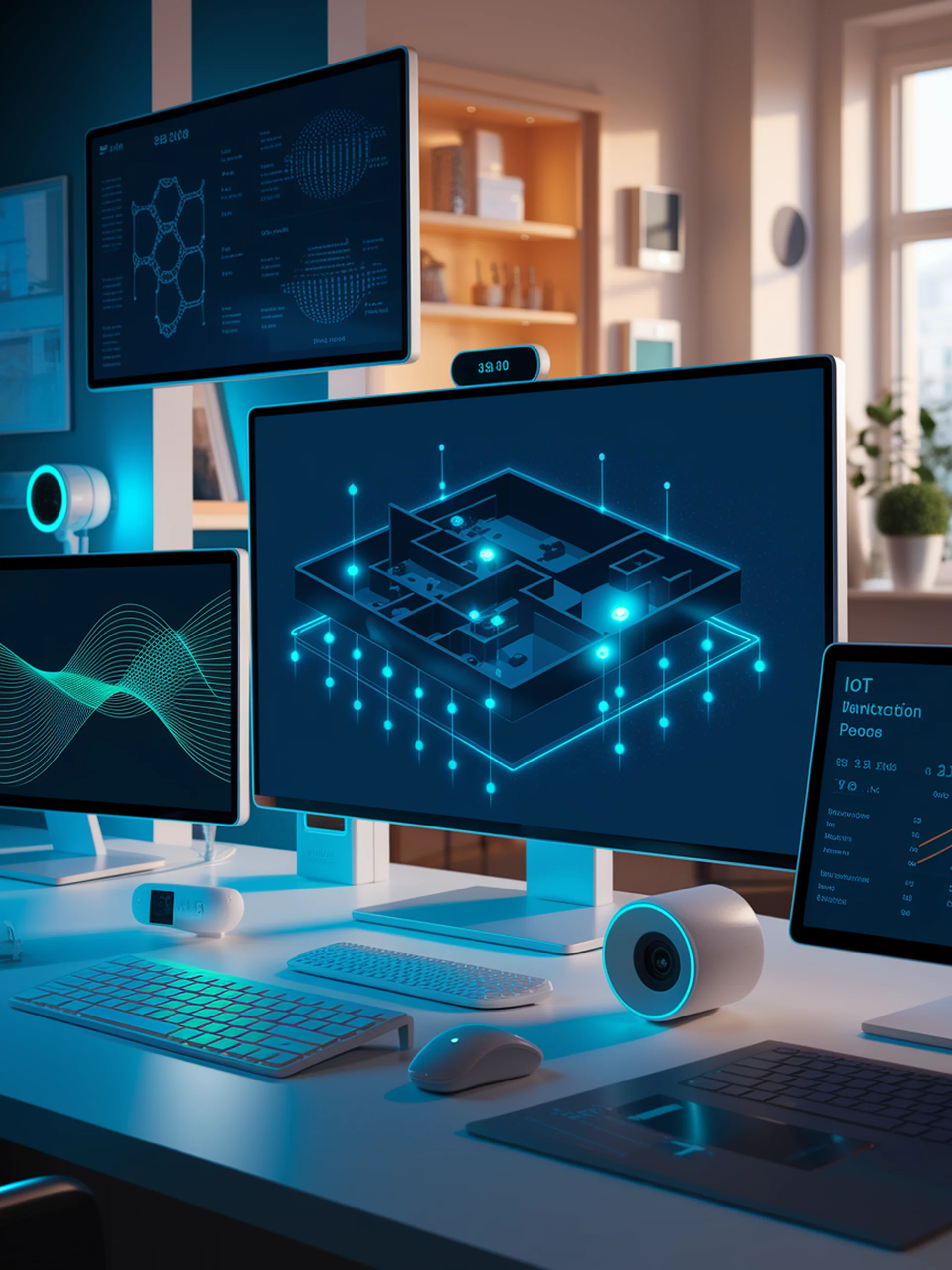
Securing Smart Homes with Synthetic Data
Using LLMs to generate realistic user behavior for security testing
This research introduces IoTGen, an innovative framework that leverages LLMs to generate synthetic smart home user behavior sequences, solving the data scarcity problem in security testing.
- Creates realistic, diverse user behavior patterns without privacy concerns
- Combines Structure Pattern Generator and LLM-based Content Generator for comprehensive data synthesis
- Outperforms existing methods with up to 93.2% realistic behavior generation
- Enables more robust anomaly detection and security testing for smart home environments
Why it matters: Smart home security solutions currently rely on limited, static datasets that fail to adapt to evolving threats and usage patterns. This approach allows continuous generation of high-quality training data without compromising user privacy.
Synthetic User Behavior Sequence Generation with Large Language Models for Smart Homes