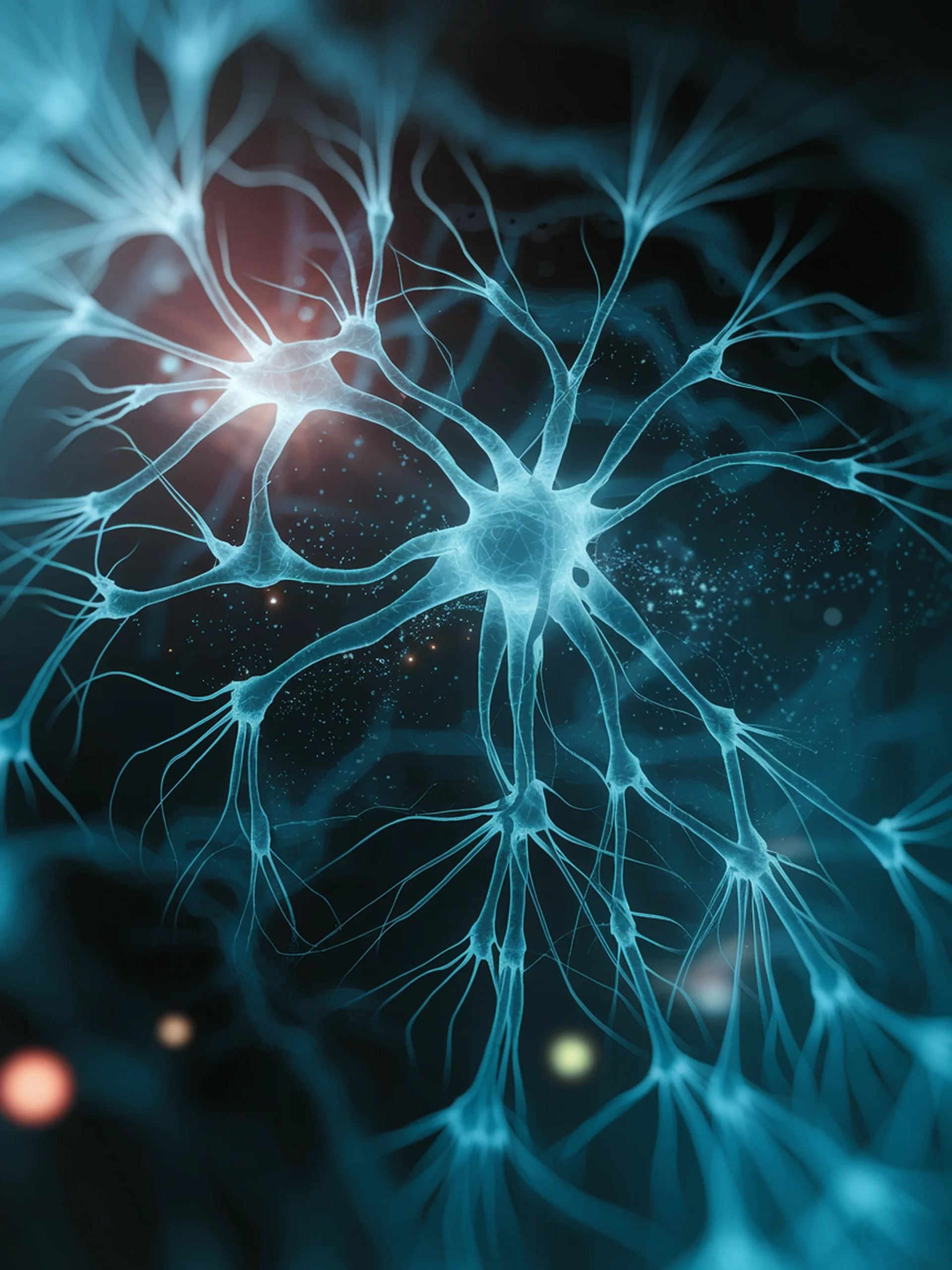
Controlling What LLMs Learn
A novel approach for supervised learning by revealing and controlling latent features
This research introduces a framework that makes LLM embeddings interpretable and controllable for classification tasks, addressing regulatory compliance and model generalization challenges.
- Proposes a self-regularization mechanism that reveals unintended features in LLM embeddings
- Enables selective removal of sensitive or task-irrelevant features from classification models
- Demonstrated improvements in toxic content detection and other security applications
- Achieves better generalization by focusing only on task-relevant features
For security teams, this approach offers a critical advancement in creating more transparent, compliant AI systems where unwanted biases or problematic features can be identified and removed.
Self-Regularization with Latent Space Explanations for Controllable LLM-based Classification