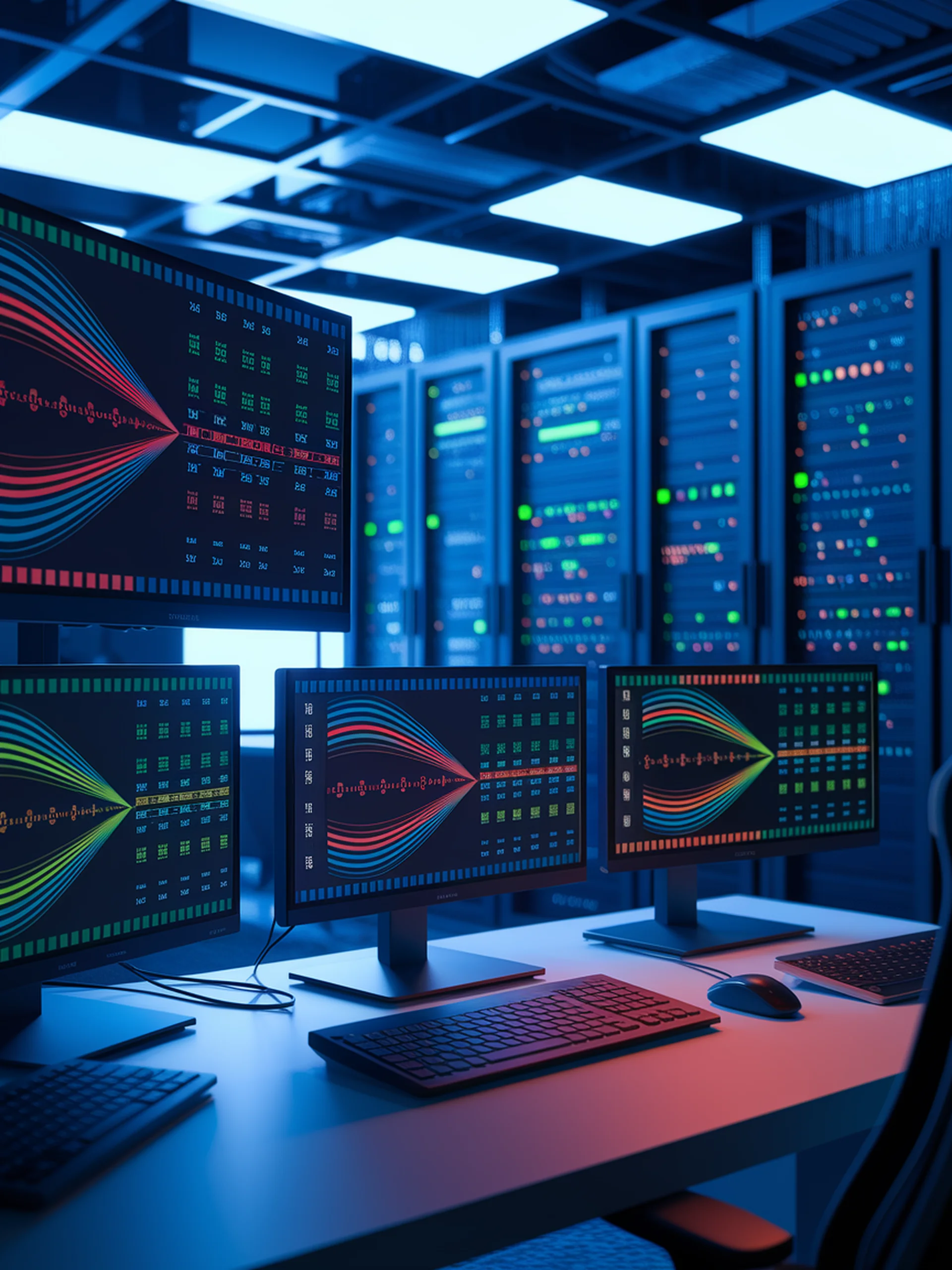
Next-Gen Network Traffic Classification
Comparing Traditional ML, Transformers, and LLMs for Enhanced Security
This research advances network security by comparing various models for classifying network traffic into categories like web browsing, IPSec, and email.
Key Findings:
- Comprehensive analysis using a dataset of 30,959 observations with 19 features collected from Arbor Edge Defender devices
- Evaluation of multiple approaches including traditional ML (Naive Bayes, Random Forest), Deep Neural Networks, Transformers, and Large Language Models (GPT-4o, Gemini)
- LLMs demonstrated promising capabilities for network traffic classification, even with zero-shot approaches
- Results provide valuable insights for improving network security monitoring and threat detection
This research matters for cybersecurity professionals seeking more effective ways to classify and monitor network traffic, potentially enhancing anomaly detection and security response capabilities.
Network Traffic Classification Using Machine Learning, Transformer, and Large Language Models