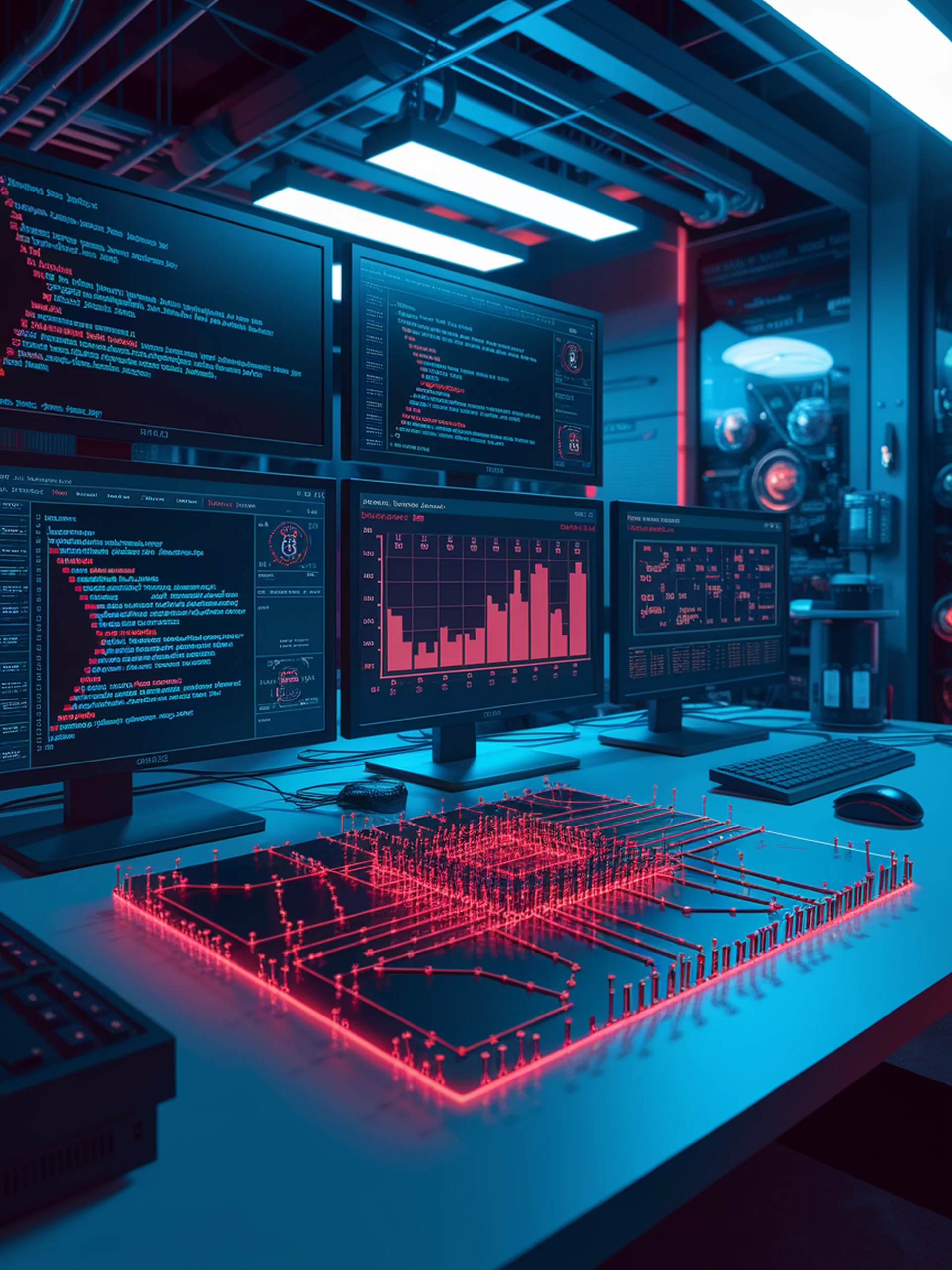
Advanced Malware Detection Through Memory Analysis
Comparing Traditional ML, Transformers, and LLMs for Security Applications
This research evaluates multiple machine learning approaches for malware classification using memory dumps, comparing traditional ML methods, deep learning, and LLMs like Gemini.
- Traditional ML: Six models tested (Logistic Regression, KNN, SVM, Decision Trees, Random Forest, XGB)
- Deep Learning: RNN and Transformer architectures evaluated
- Zero/Few-Shot Learning: Gemini LLM capabilities tested for malware identification
- Comprehensive Comparison: Analysis of accuracy, efficiency, and practical deployment considerations
The findings provide critical insights for security professionals seeking to implement more effective and adaptable malware detection systems that can identify threats from memory analysis alone.