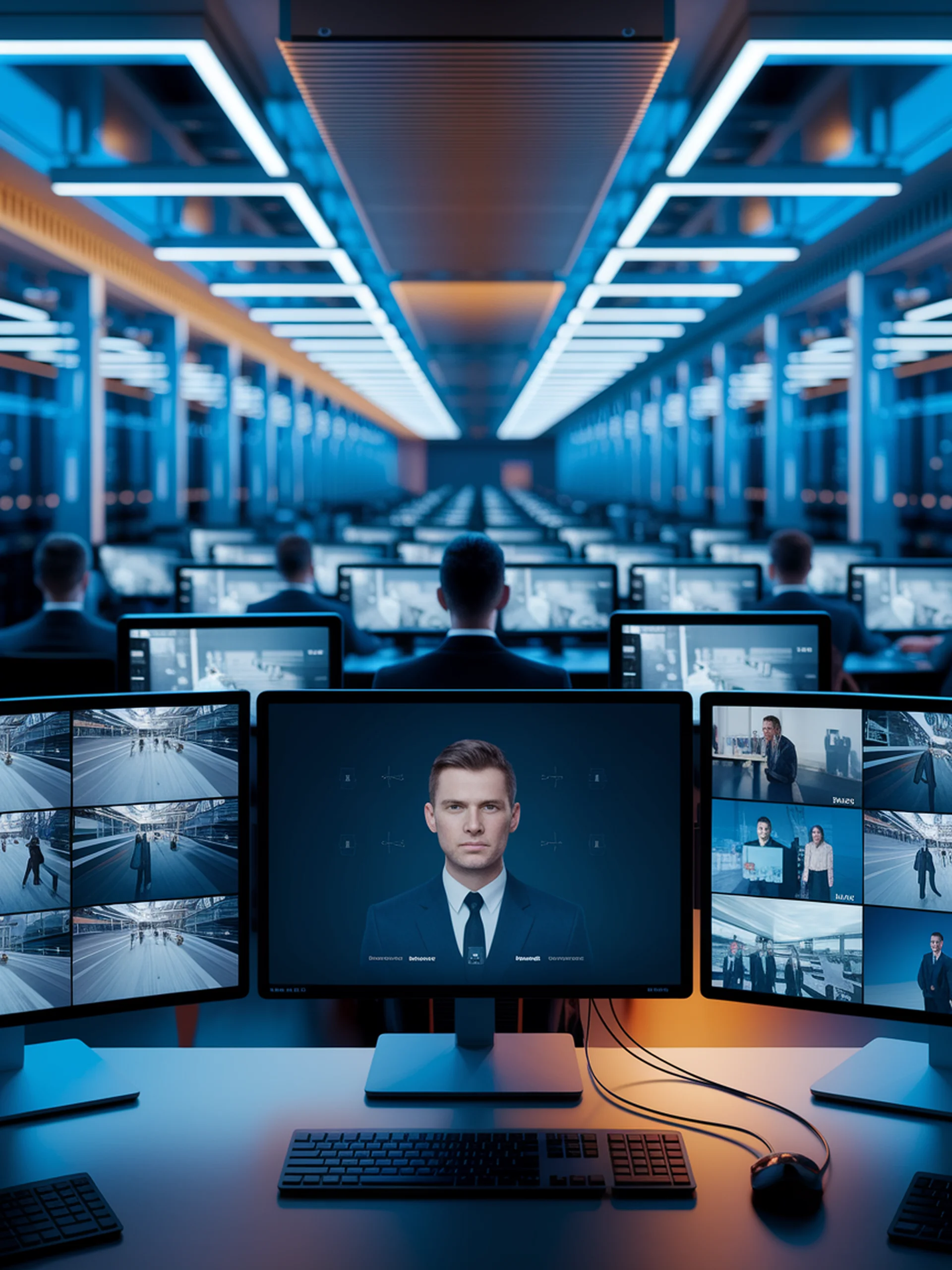
Scaling Person Identification with AI Annotations
Using AI to Model Human-like Diversity in ReID Systems
This research addresses the challenge of building text-to-image person re-identification systems that can generalize across different scenarios without massive human annotation costs.
- Introduces a novel approach modeling thousands of virtual human annotators with diverse description styles
- Achieves superior cross-dataset generalization compared to MLLM-based annotations
- Demonstrates significant performance improvements on CUHK-PEDES, ICFG-PEDES, and RSTPReid datasets
- Provides a cost-effective solution for large-scale security systems needing robust person identification
For security applications, this advancement enables more reliable surveillance systems that can identify persons of interest using natural language descriptions, even in new environments with different visual conditions.
Modeling Thousands of Human Annotators for Generalizable Text-to-Image Person Re-identification