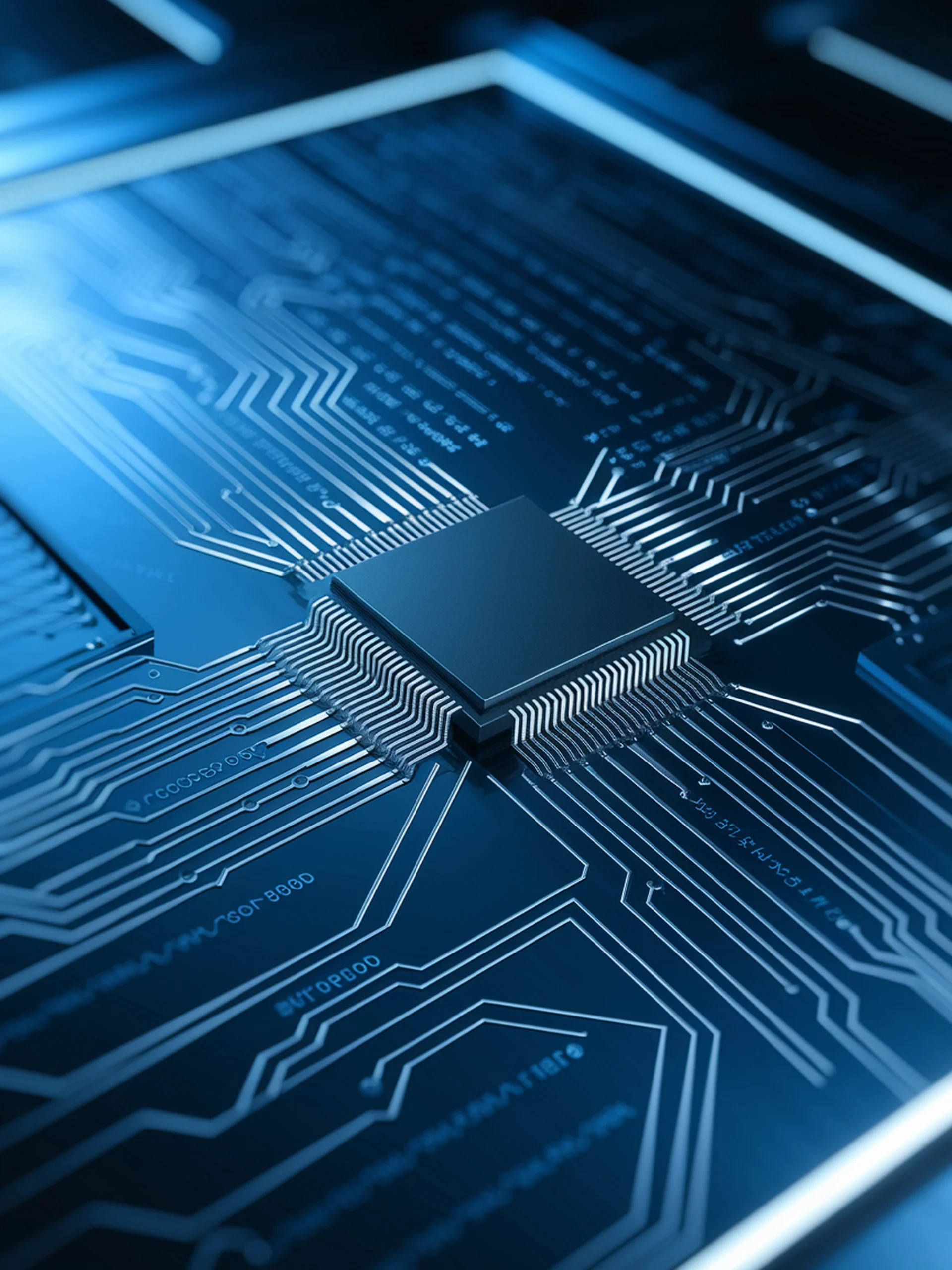
Unlocking Assembly Code Comprehension with AI
A new approach to making assembly code accessible through LLMs
ASMA-Tune represents a breakthrough in enabling large language models to understand and interact with assembly code through a novel structural-semantic instruction tuning framework.
- Combines assembly code's structural properties with semantic understanding to enhance LLM comprehension
- Overcomes the inherent challenges of assembly code's low information density and lack of explicit syntax
- Bridges the gap between MLM-based methods and decoder-focused LLMs for assembly analysis
- Creates new opportunities for security analysts in reverse engineering and vulnerability detection
This innovation has significant implications for the cybersecurity industry, making assembly code analysis more accessible and potentially accelerating vulnerability discovery and malware detection processes.
ASMA-Tune: Unlocking LLMs' Assembly Code Comprehension via Structural-Semantic Instruction Tuning