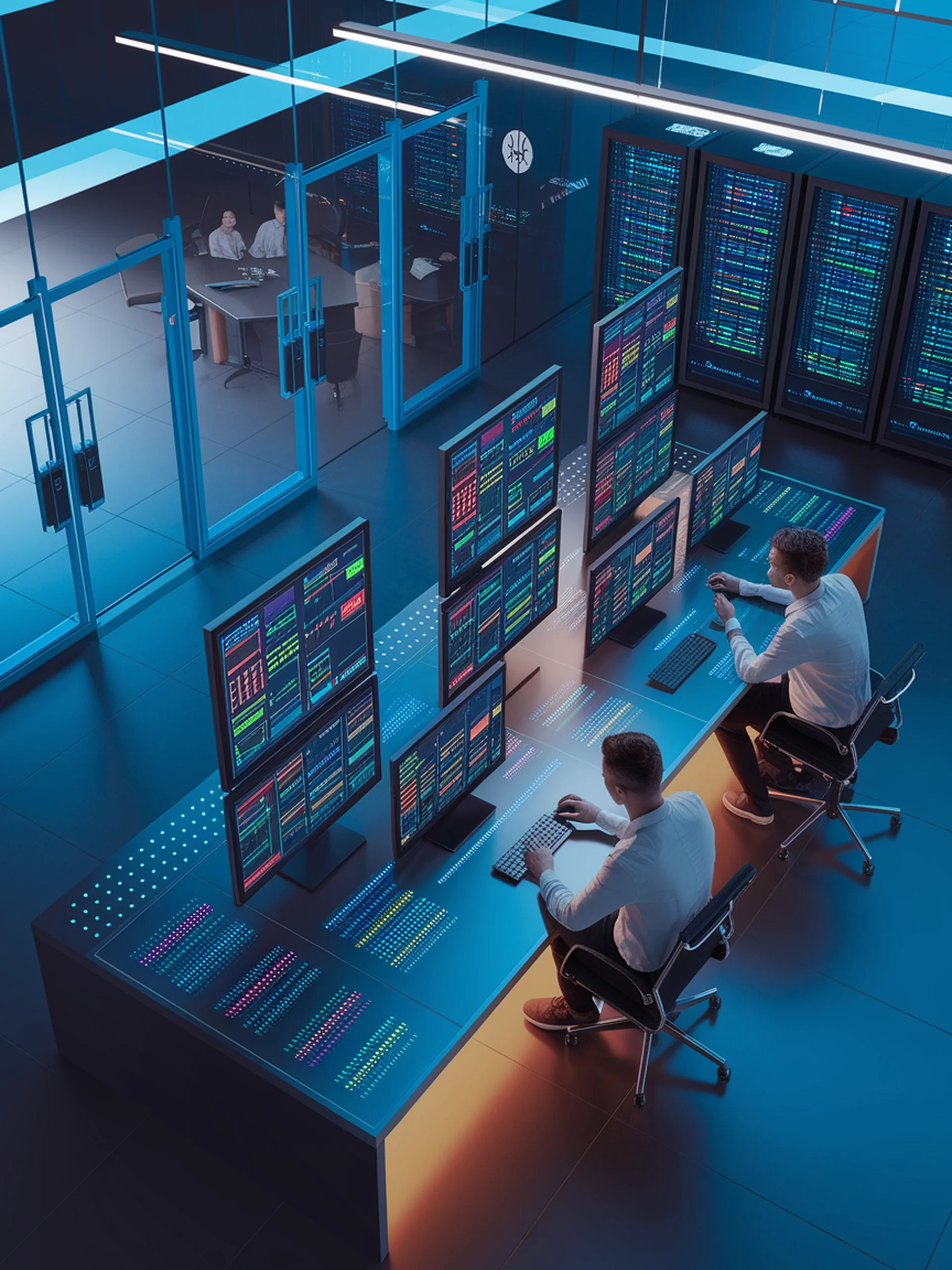
S-Eval: Securing the Future of LLMs
A systematic framework for comprehensive LLM safety evaluation
This research introduces a structured safety evaluation framework for identifying and mitigating harmful content in large language models before deployment.
- Establishes a comprehensive risk taxonomy specifically designed for LLM safety assessment
- Provides automated evaluation techniques to identify potential security threats in LLM outputs
- Enables rigorous pre-deployment testing to prevent harmful content generation
- Addresses critical ethical ramifications of deploying advanced language models
This framework is essential for security professionals as it offers systematic methods to evaluate LLM safety, helping organizations mitigate risks of harmful content generation while maintaining the benefits of these powerful AI systems.
S-Eval: Towards Automated and Comprehensive Safety Evaluation for Large Language Models