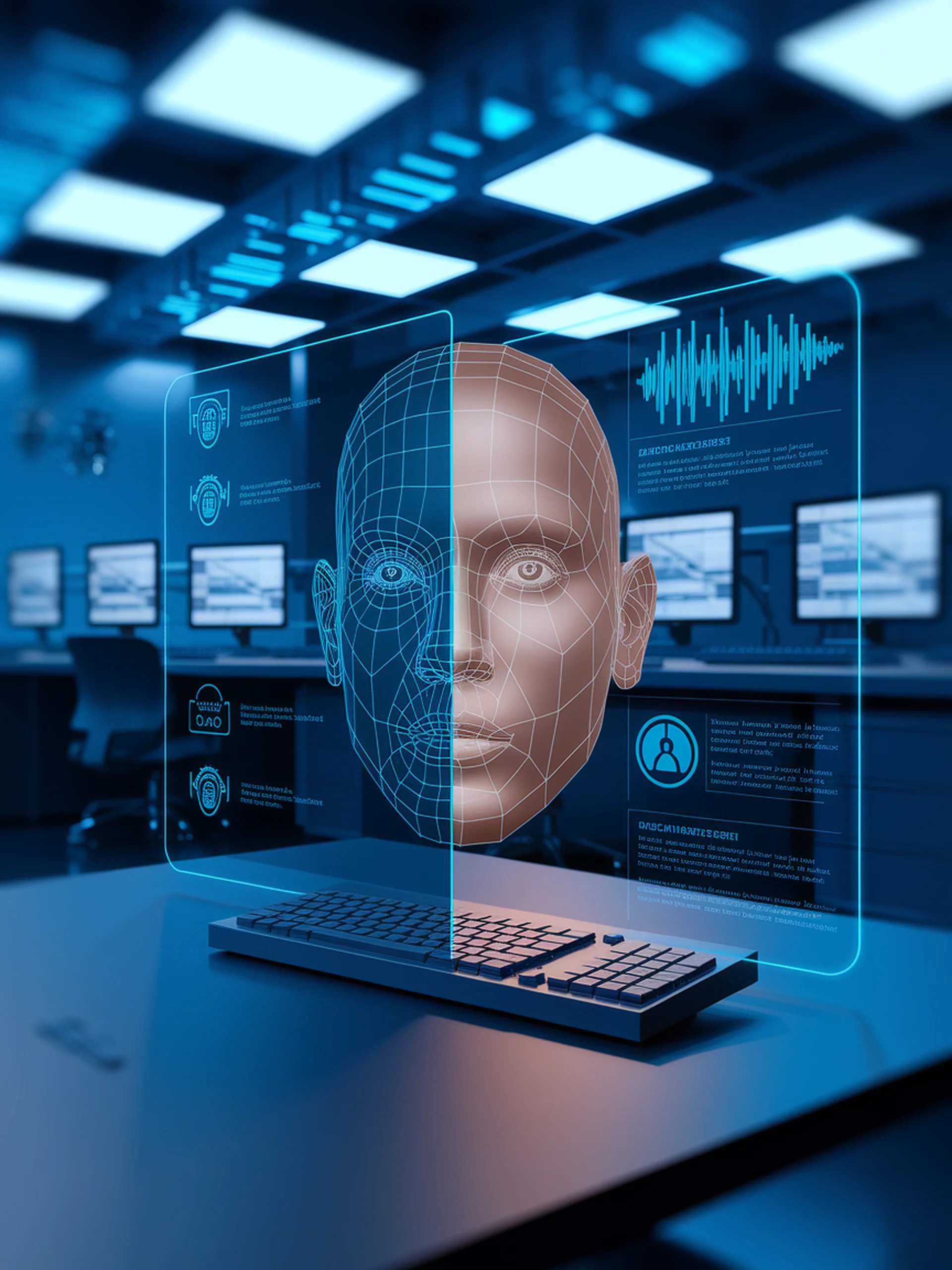
The Evolution of Deepfake Detection
From Single-Modal to Multi-Modal Approaches for Enhanced Security
This research traces how facial deepfake detection has evolved from analyzing single data types to sophisticated multi-modal approaches that integrate audio-visual and text-visual signals.
Key Developments:
- Presents a structured taxonomy of detection techniques across different modalities
- Analyzes the transition from GAN-based to diffusion model-driven deepfake generation and detection
- Examines integration of audio-visual and text-visual cues for more robust detection
- Addresses challenges in combating increasingly realistic synthetic media
Security Implications: As synthetic media becomes virtually indistinguishable from authentic content, this research provides critical frameworks to combat identity fraud, misinformation campaigns, and social manipulation through advanced detection methods.
Evolving from Single-modal to Multi-modal Facial Deepfake Detection: Progress and Challenges