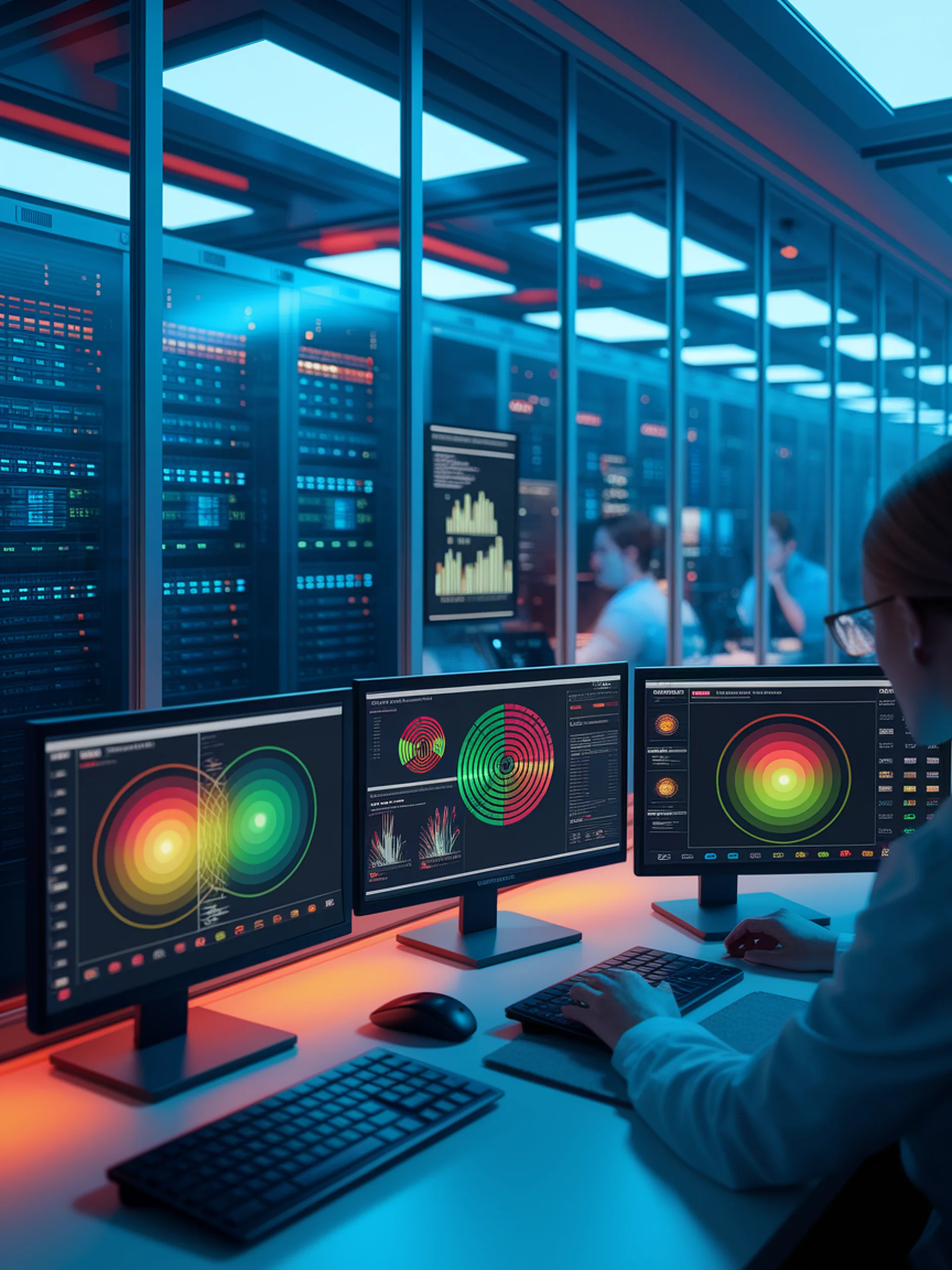
Measuring LLM Safety Through Offensive Content Progression
A new approach to benchmarking model sensitivity to harmful content
The STOP dataset introduces a novel methodology for evaluating biases in Large Language Models by testing how they respond to increasingly offensive content.
- Tests 450 offensive progressions with 2,700 unique sentences of varied severity
- Enables more comprehensive assessment than isolated test cases
- Specifically designed to improve security standards in language models
- Contributes to ethical AI development by identifying and mitigating bias patterns
Security Impact: By systematically evaluating how models handle increasingly harmful content, organizations can build more robust safeguards and reduce potential security vulnerabilities in deployed AI systems.
STOP! Benchmarking Large Language Models with Sensitivity Testing on Offensive Progressions