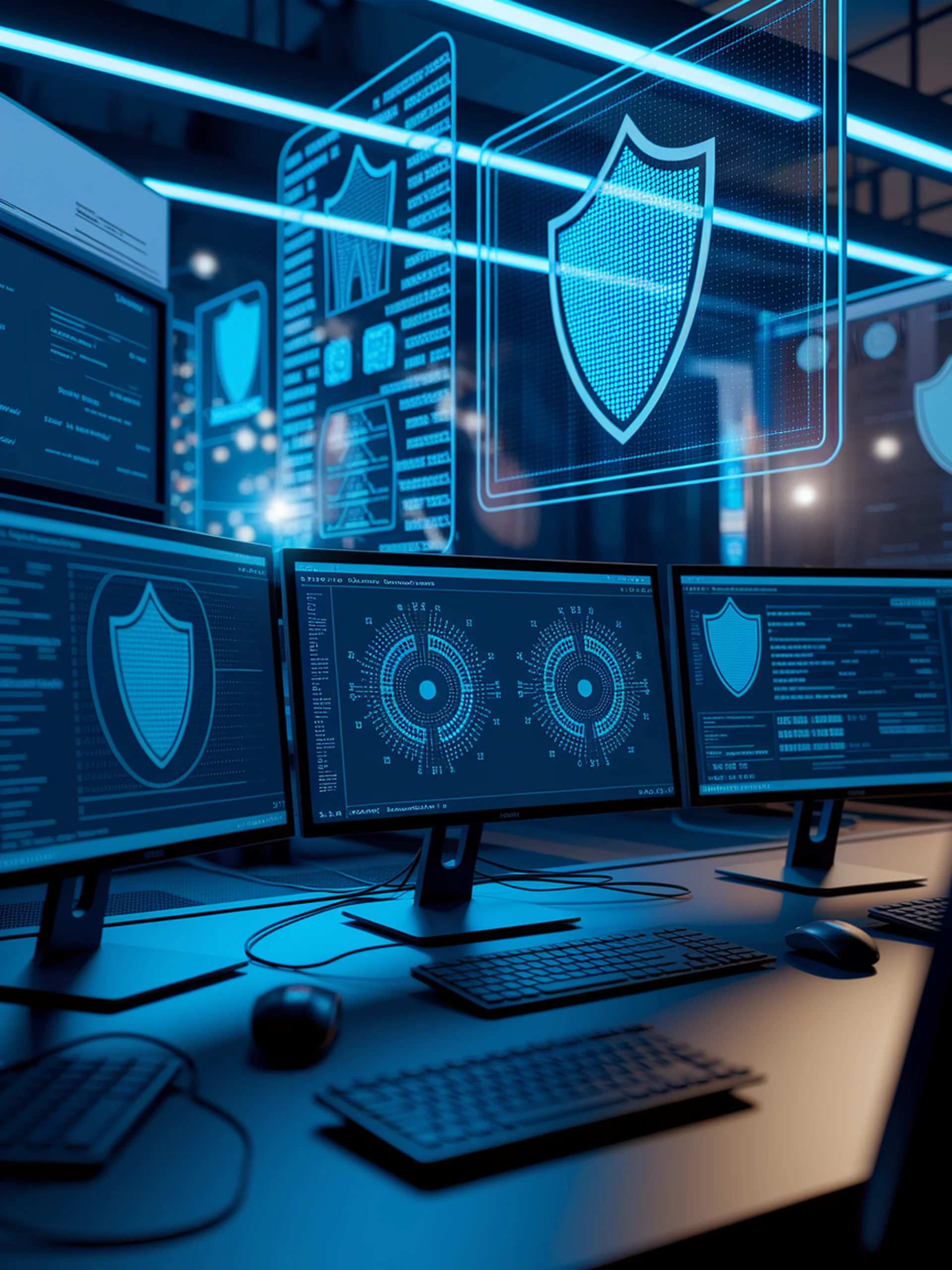
Proactive LLM Safety Auditing
A novel approach for detecting catastrophic AI responses before they cause harm
Output Scouting is a systematic methodology for identifying potentially harmful responses from Large Language Models before they reach production environments.
- Addresses the critical challenge that even well-trained LLMs have non-zero probabilities of generating harmful outputs
- Uses a strategic sampling approach to find outputs with specific harmful characteristics
- Demonstrates effectiveness through real-world testing across multiple safety-critical scenarios
- Provides security professionals with a practical framework for proactive LLM risk assessment
This research is essential for organizations deploying LLMs, offering a structured approach to identify and mitigate security vulnerabilities before they impact users or create legal liability.
Output Scouting: Auditing Large Language Models for Catastrophic Responses