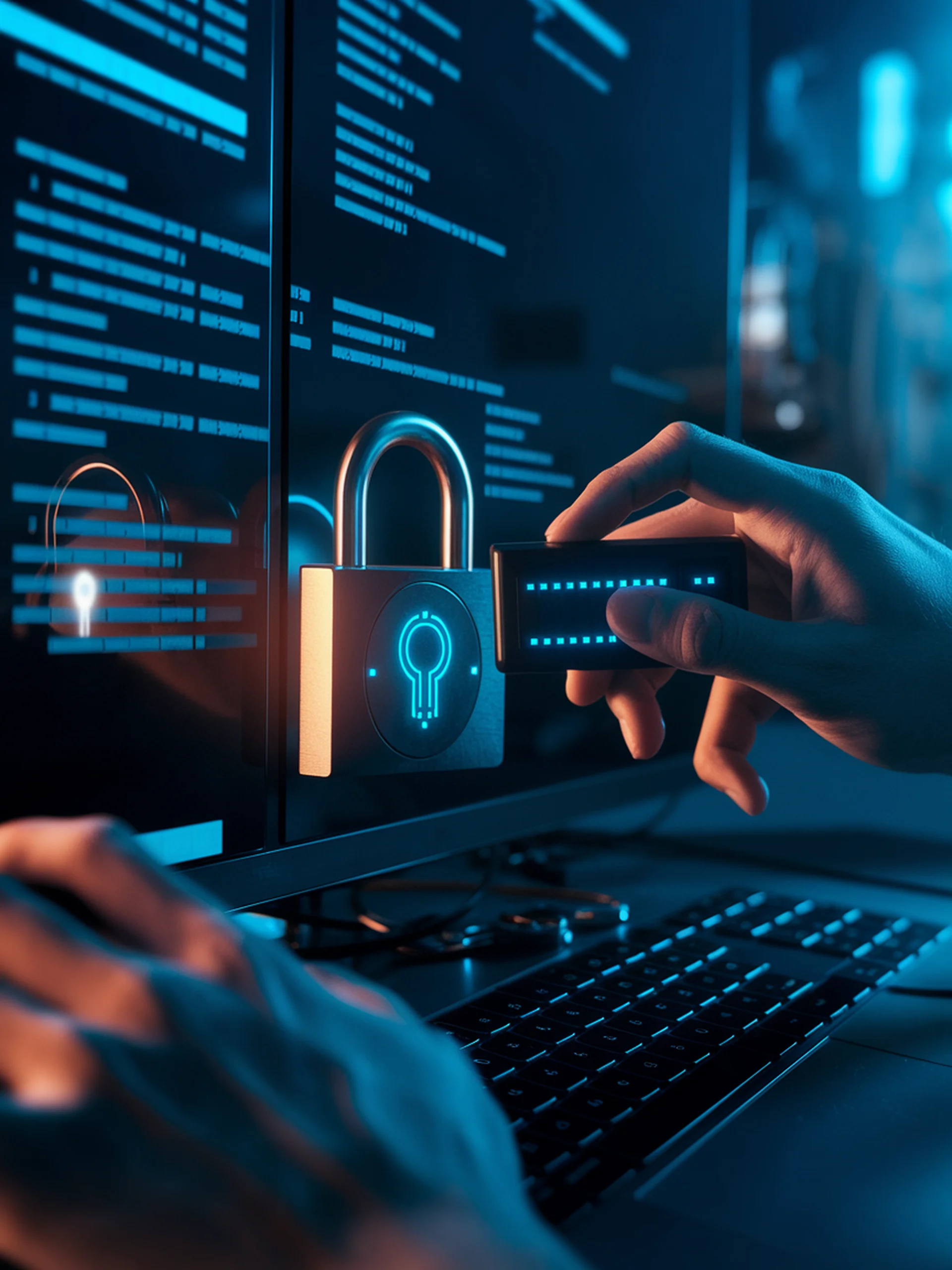
Fooling LLM Detectors: The Proxy Attack Strategy
How simple attacks can make AI-generated text pass as human-written
This research reveals a critical vulnerability in systems designed to identify AI-generated content, demonstrating how easily LLM detectors can be compromised through proxy attacks.
- Introduces a novel proxy-attack strategy that manipulates LLMs to produce text that evades detection
- Achieves up to 83% success rate against leading detectors while maintaining text quality
- Reveals that even state-of-the-art detection systems have significant security flaws
- Works without requiring access to detector architecture or extensive computational resources
For cybersecurity professionals, this research highlights an urgent need to develop more robust detection systems as bad actors could easily exploit these vulnerabilities to spread misinformation or automated content while appearing human-authored.
Humanizing the Machine: Proxy Attacks to Mislead LLM Detectors