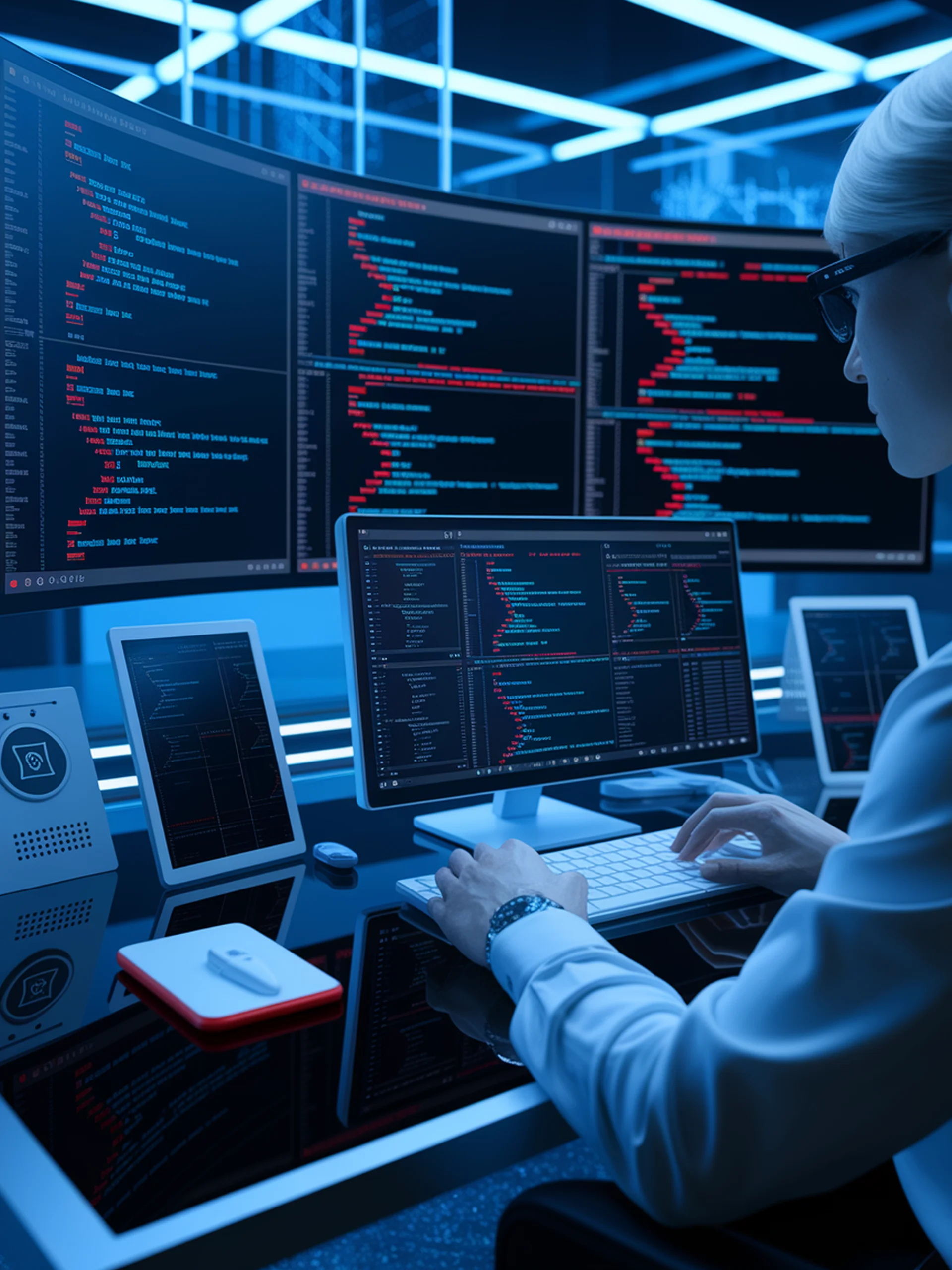
Smarter Security Testing for AI Image Generators
Using LLMs to systematically find vulnerabilities in text-to-image models
ICER is a novel red-teaming framework that uses Large Language Models and bandit optimization to generate interpretable attacks against text-to-image models, systematically probing for safety weaknesses.
- Creates semantic manipulations of prompts to circumvent safety filters
- Employs in-context experience replay to learn from successful attacks
- Provides a systematic evaluation of safety mechanisms in text-to-image systems
- Develops interpretable strategies for identifying vulnerabilities
This research advances AI security by providing essential tools to evaluate safety mechanisms before deployment, helping protect against the generation of harmful content while preserving the creative potential of text-to-image technology.
In-Context Experience Replay Facilitates Safety Red-Teaming of Text-to-Image Diffusion Models