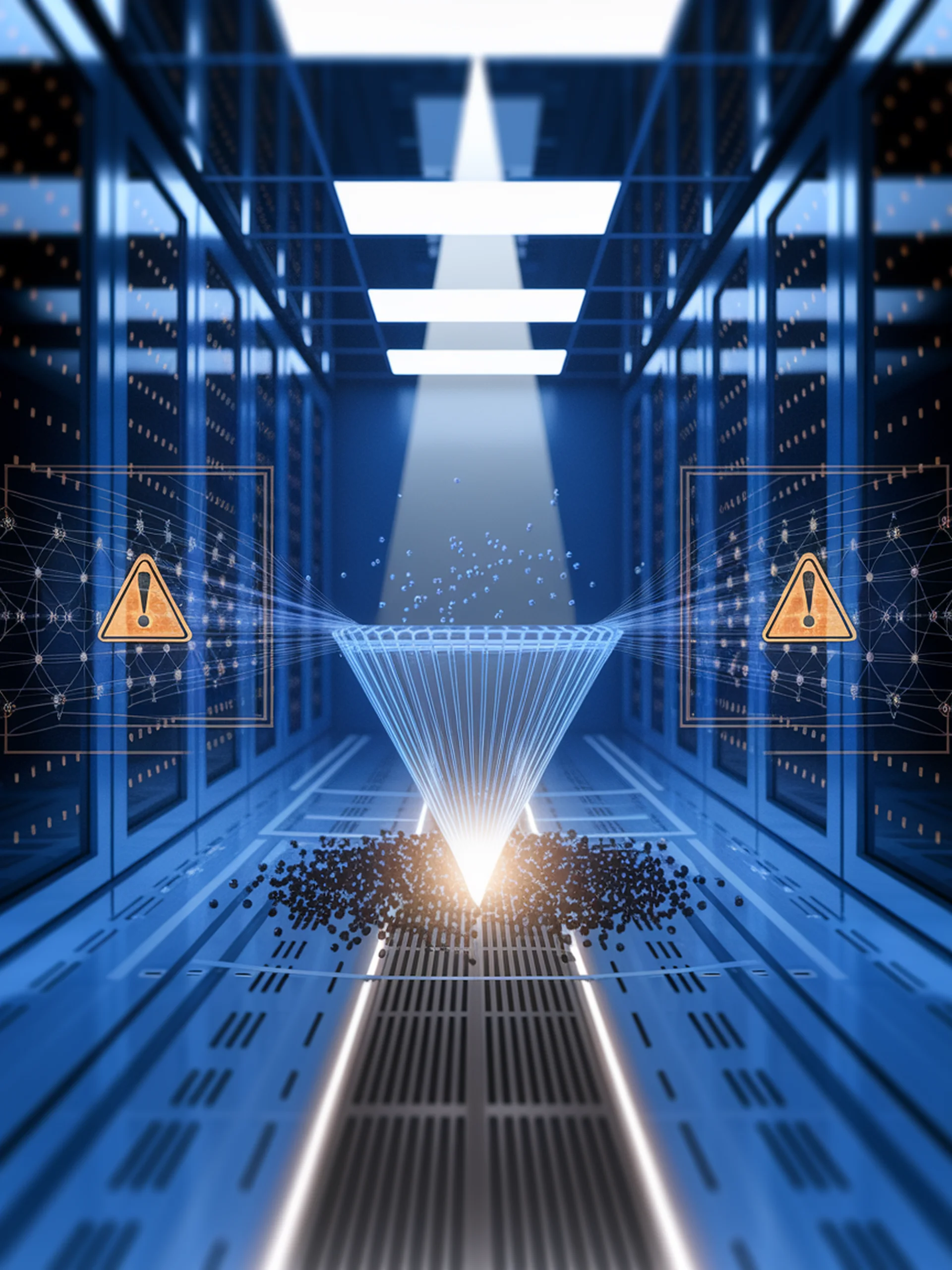
Securing LLMs Against Harmful Content
A Dynamic Filtering Approach Without Retraining
DIESEL introduces a novel semantic guidance mechanism for filtering undesired content from Large Language Models without requiring expensive retraining.
- Uses comparison to reference embeddings to dynamically detect and filter unsafe content
- Achieves high effectiveness against adversarial jailbreaking attacks
- Provides computational efficiency compared to other alignment techniques
- Maintains model performance while enhancing security guardrails
This research addresses critical security concerns in AI deployment by offering a practical approach to prevent LLMs from generating harmful or unaligned responses, essential for responsible AI implementation in business contexts.
DIESEL -- Dynamic Inference-Guidance via Evasion of Semantic Embeddings in LLMs