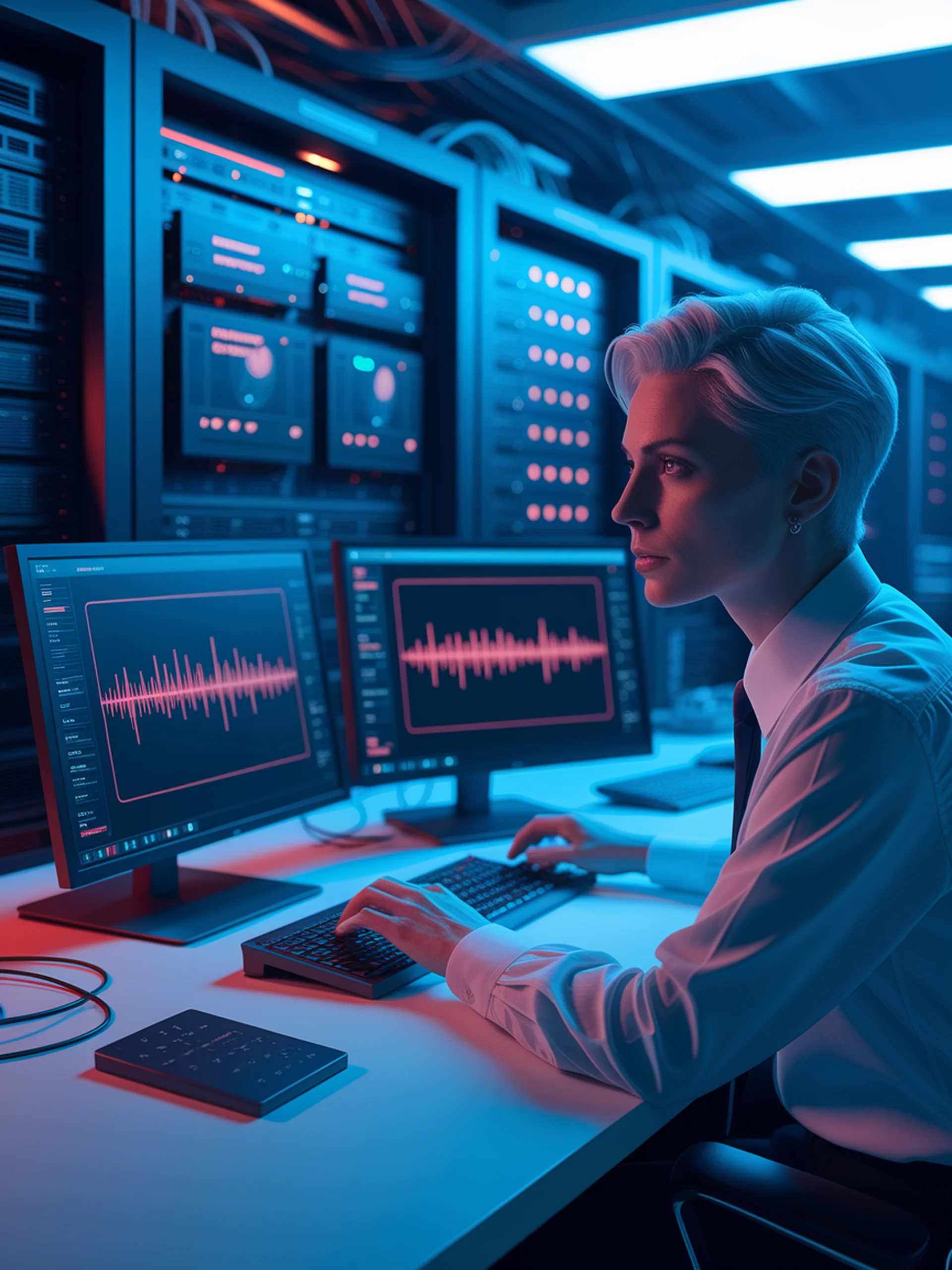
Combating Evolving Toxic Content
Adaptable Detection Systems for Security in LLMs
This research addresses the critical challenge of detecting toxic content that evolves to evade detection systems, particularly in Large Language Models.
- Introduces a novel few-shot learning framework that adapts to new perturbation patterns
- Demonstrates superior performance against jailbreak attempts and evolving attack methods
- Provides a sustainable approach to toxicity detection that requires minimal retraining
- Significantly improves security resilience against malicious users trying to bypass content safeguards
For security professionals, this research offers crucial insights into building more robust content moderation systems that can adapt to the constantly evolving landscape of toxic content disguise methods.
Original Paper: Toxicity Detection towards Adaptability to Changing Perturbations