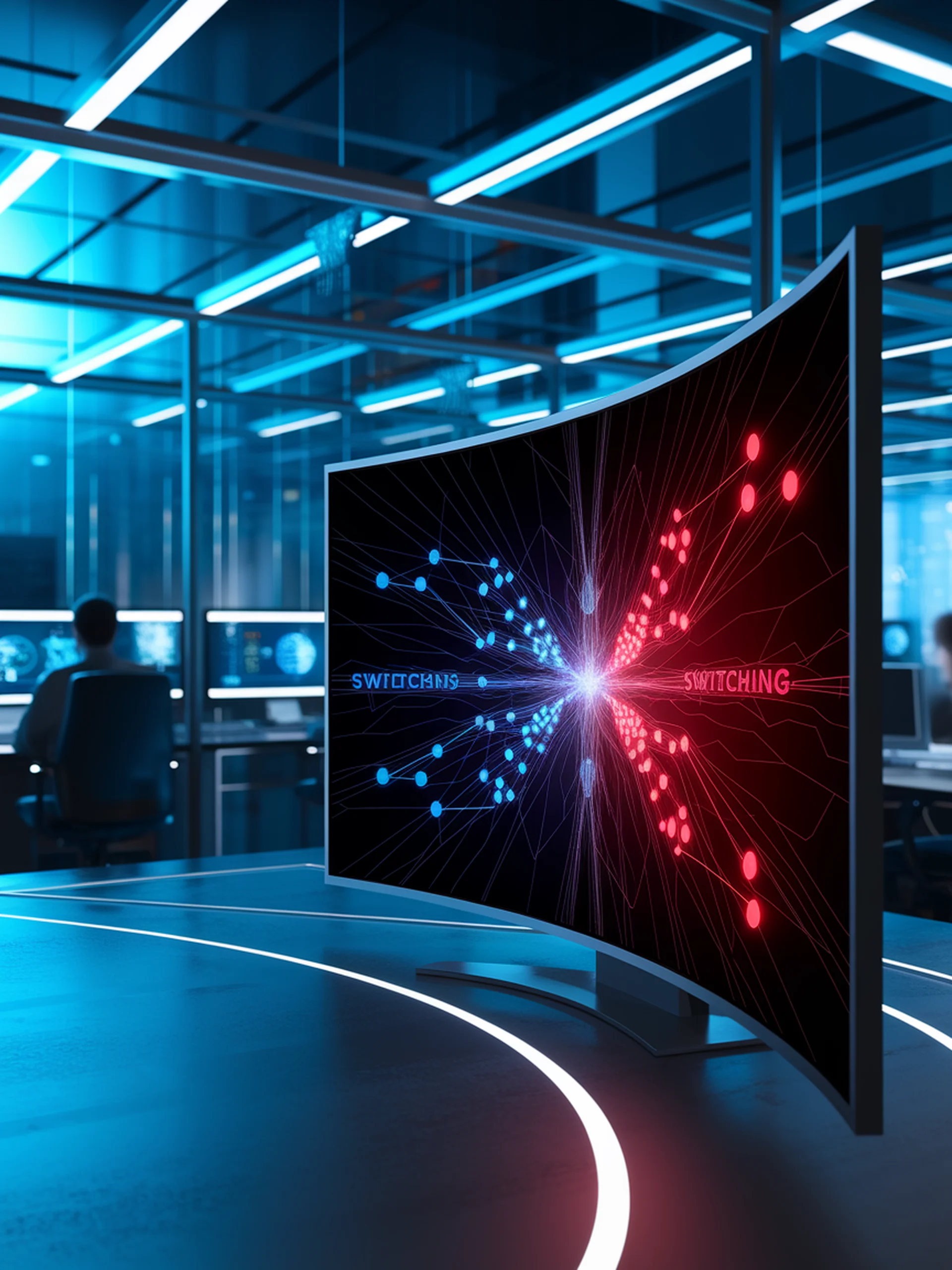
SafeSwitch: Smarter AI Safety Controls
Using internal activation patterns to regulate LLM behavior without sacrificing capability
This research introduces a novel approach to AI safety that monitors internal activations within large language models to detect and prevent harmful outputs while preserving model capabilities.
- Leverages System 2 thinking principles from cognitive science to regulate LLM behavior
- Identifies specific neural activation patterns that correspond to unsafe content generation
- Achieves 95.7% effectiveness in preventing harmful outputs while maintaining model utility
- Demonstrates a more targeted approach than existing safety measures that often cause overcautious behavior
For security teams, this framework offers a promising path to implement more nuanced safety controls that don't compromise LLM performance in legitimate use cases.
Internal Activation as the Polar Star for Steering Unsafe LLM Behavior