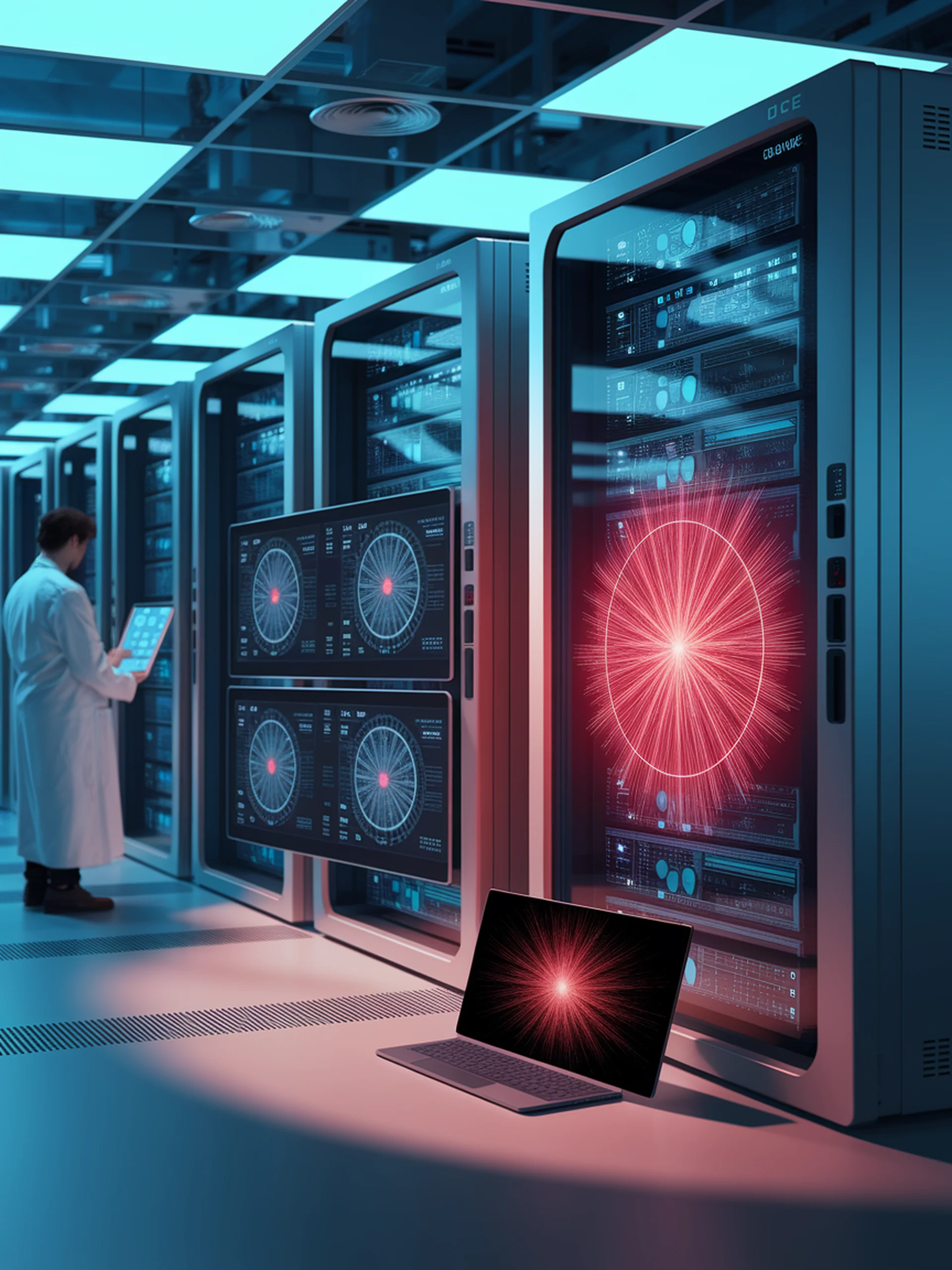
Evaluating the Blind Spots of LLM Safety
Can larger models accurately detect harm in smaller ones?
This research examines whether large language models can reliably evaluate the harmfulness of smaller LLMs - revealing significant limitations in current evaluation approaches.
Key Findings:
- Larger LLMs show inconsistent ability to rank smaller models' harmfulness
- Evaluations suffer from model alignment biases when assessing similar architecture families
- Human annotation remains necessary for reliable harm evaluation
- Edge device deployments of smaller LLMs require specialized safety assessments
Security Implications: As smaller LLMs become more common on resource-constrained devices, understanding their varying tendencies to generate harmful content is critical for responsible deployment. This research highlights that automated safety evaluation through larger models is not yet reliable enough for production environments.
Can LLMs Rank the Harmfulness of Smaller LLMs? We are Not There Yet