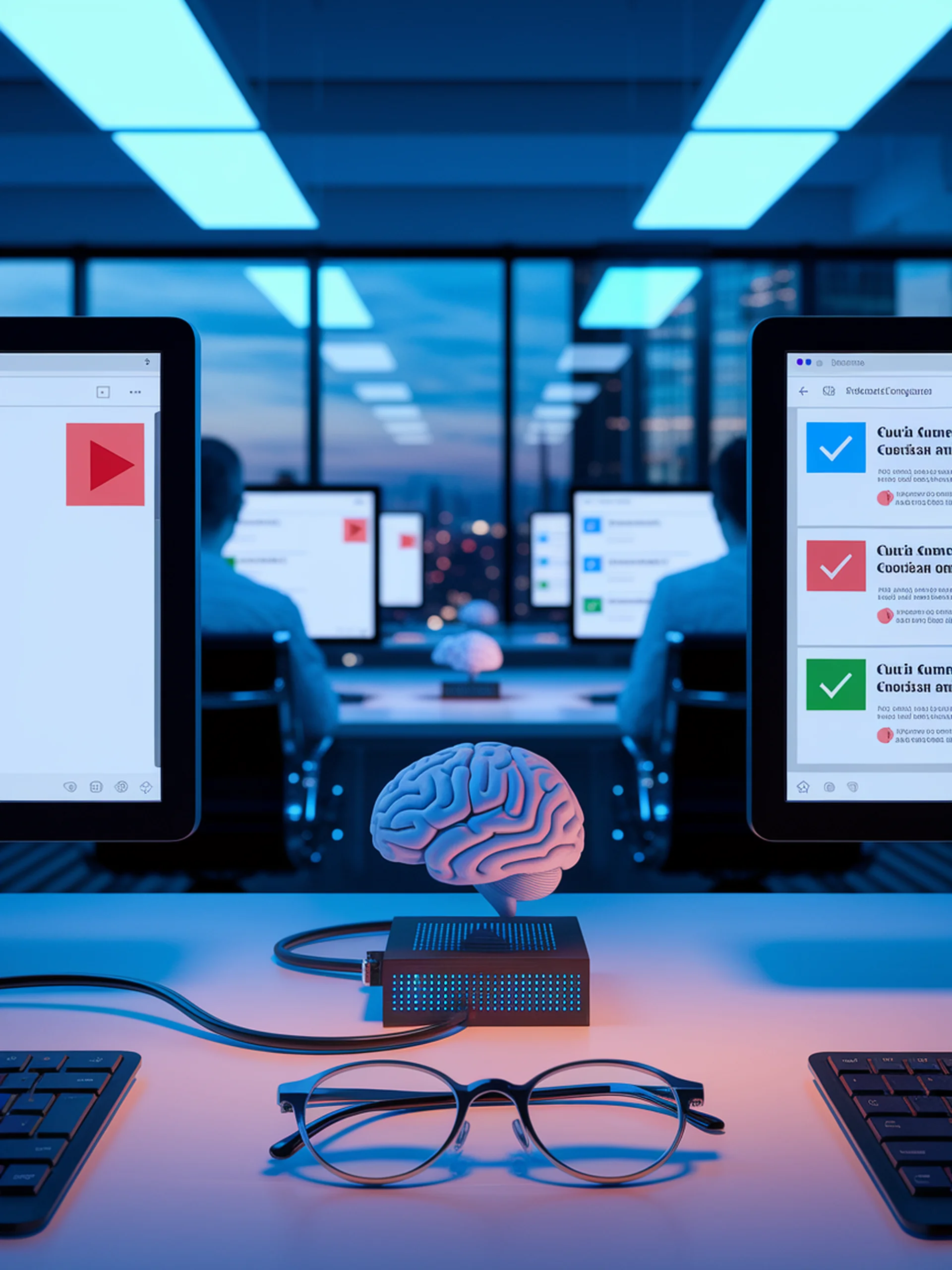
LLMs and Annotation Disagreement
How AI handles ambiguity in offensive language detection
This research explores how Large Language Models perform when faced with human disagreement in offensive content labeling.
- Examines LLM confidence levels when processing ambiguous offensive language cases
- Evaluates multiple LLMs on their alignment with human annotator perspectives
- Reveals insights into AI decision-making for subjective content moderation tasks
- Addresses a critical gap in understanding how AI handles content that humans disagree about
Security Impact: The findings directly enhance content moderation systems by improving how AI handles ambiguous harmful content, creating more nuanced and effective digital safety tools.