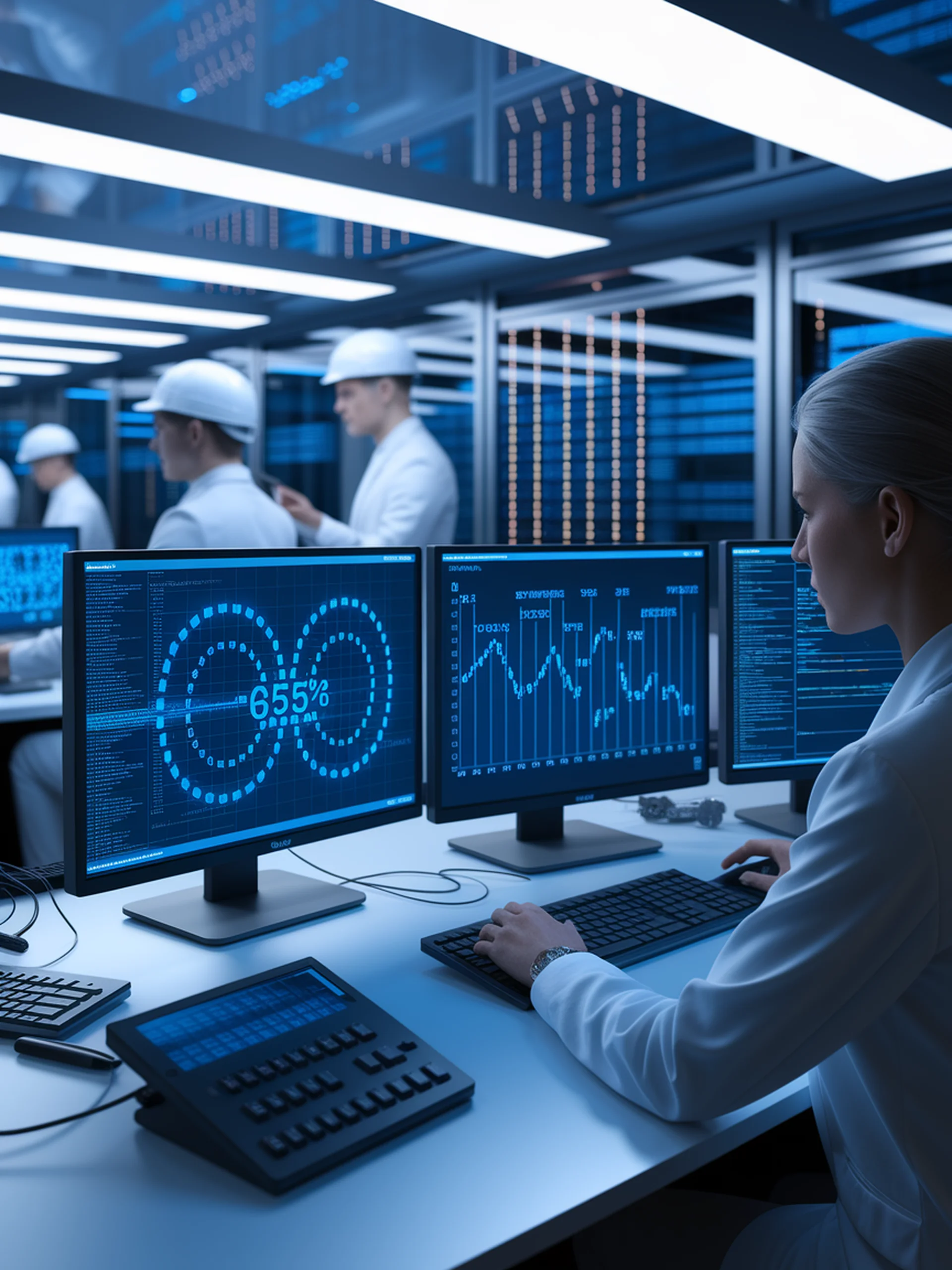
Intent-Aware Repair for Safer LLMs
Precision-targeting toxic behaviors without compromising model capabilities
IRepair introduces a novel approach to fix toxic behaviors in Large Language Models while preserving their general capabilities.
- Uses intent recognition to identify harmful patterns without broad parameter changes
- Achieves 65% reduction in toxicity while maintaining overall performance
- Employs targeted parameter updates rather than indiscriminate fine-tuning
- Demonstrates superior repair quality compared to conventional domain-adaptive training
This research addresses critical security concerns by providing a surgical approach to eliminate harmful outputs that could pose legal and ethical risks when deploying LLMs in commercial applications.
IRepair: An Intent-Aware Approach to Repair Data-Driven Errors in Large Language Models