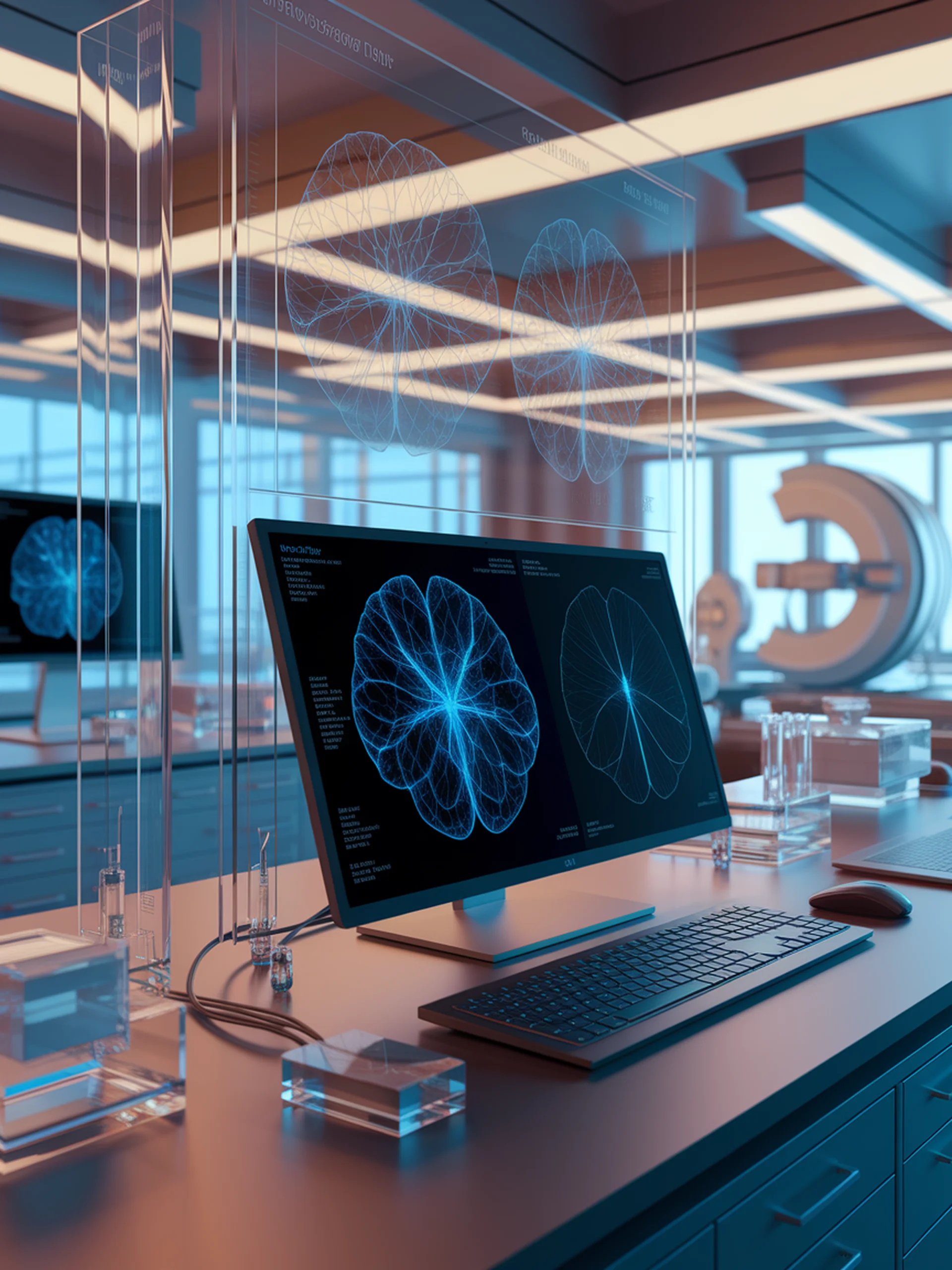
Unlocking LLM Transparency with Sparse Autoencoders
Optimizing interpretable features for critical classifications
This research systematically analyzes Sparse Autoencoders (SAEs) as tools for extracting human-interpretable representations from Large Language Models, essential for applications requiring transparency and control.
- Evaluates optimal model-layer selection and scaling properties for feature extraction
- Investigates different SAE architectural configurations including width and pooling strategies
- Demonstrates SAEs can extract interpretable features useful for classification tasks
- Explores transferability of features across models and tasks
Medical Significance: In healthcare applications, this approach enhances explainability and transparency of AI systems—critical for clinical decision support, medical diagnostics, and ensuring regulatory compliance where interpretability is non-negotiable.
Sparse Autoencoder Features for Classifications and Transferability