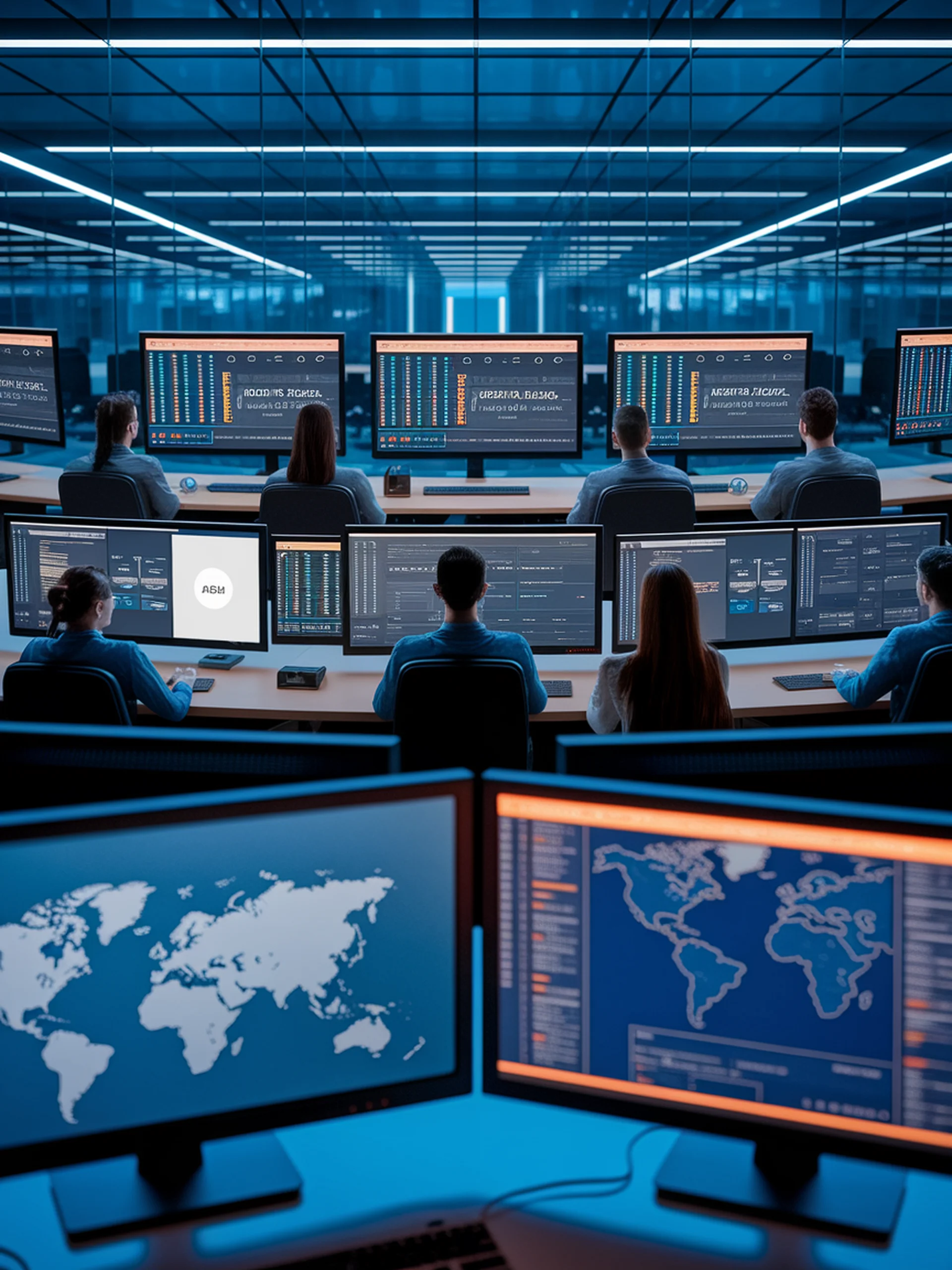
Geographically-Aware Hate Speech Detection
Evaluating LLMs for culturally contextualized content moderation
This research evaluates how effectively large language models can detect hate speech across different languages and cultural contexts, addressing a critical security challenge in content moderation.
- Systematically tests LLM performance across multilingual datasets from diverse geographic regions
- Examines model robustness against adversarial attacks in hate speech detection
- Highlights the importance of cultural and contextual factors in accurate content moderation
- Provides insights for developing more geographically-aware AI systems for security applications
This work offers significant value for security professionals building more effective and culturally-sensitive content moderation systems that can adapt to regional linguistic variations and contextual nuances.
Evaluation of Hate Speech Detection Using Large Language Models and Geographical Contextualization