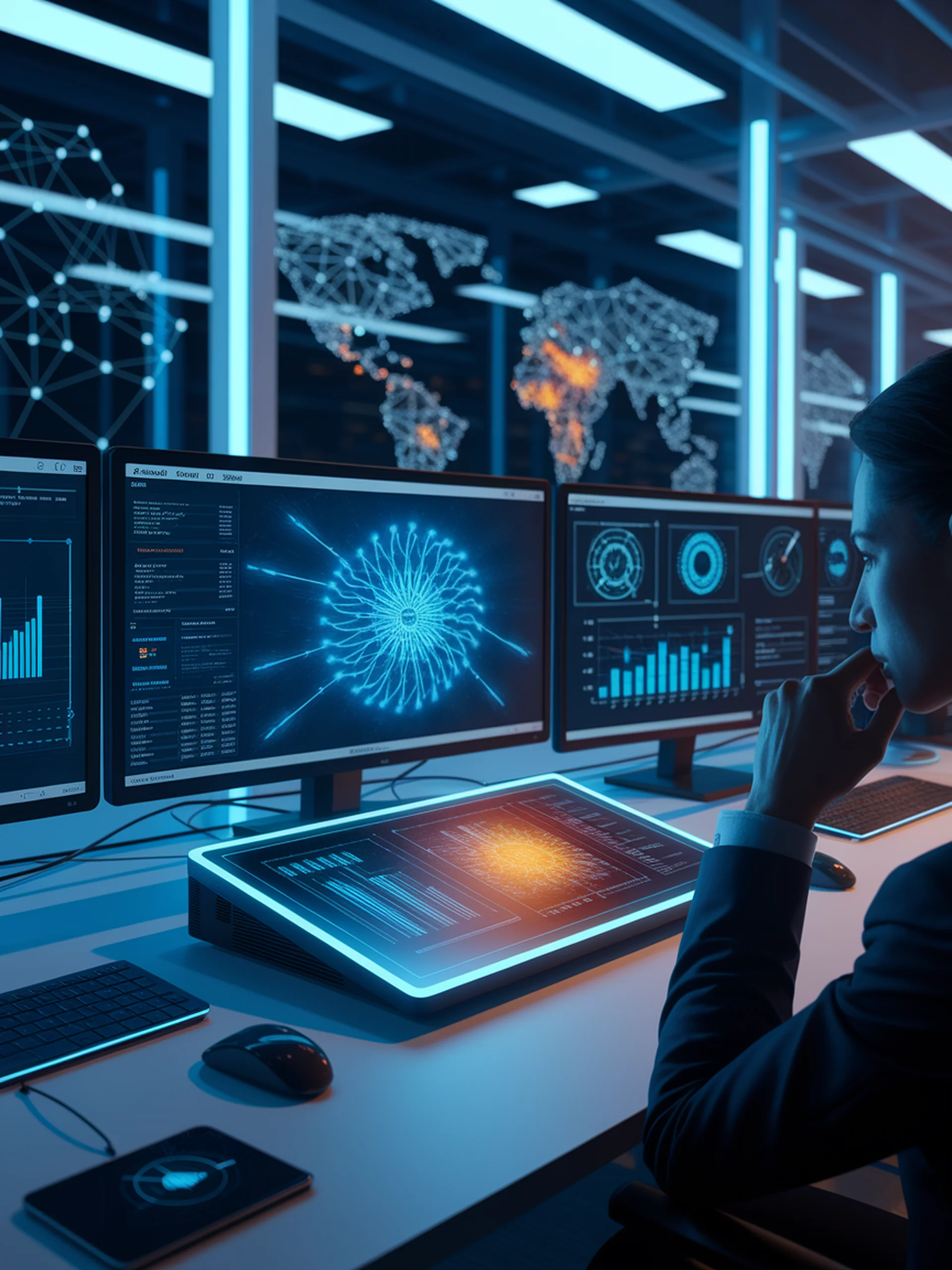
Controlling Toxic AI Outputs
A statistical approach to safer large language models
This research introduces a conformal prediction framework to control harmful outputs from LLMs with statistical guarantees.
- Focuses specifically on tail events - rare but highly problematic outputs like toxic or offensive content
- Provides a cost-efficient method that doesn't rely on expensive human annotations
- Delivers reliable control of LLM outputs with mathematical guarantees
- Enhances security and safety for real-world AI deployments
Why it matters: As LLMs become more widespread, controlling their worst-case behaviors becomes critical for safe deployment in sensitive environments. This approach offers a practical solution for organizations to implement robust security controls.
Conformal Tail Risk Control for Large Language Model Alignment