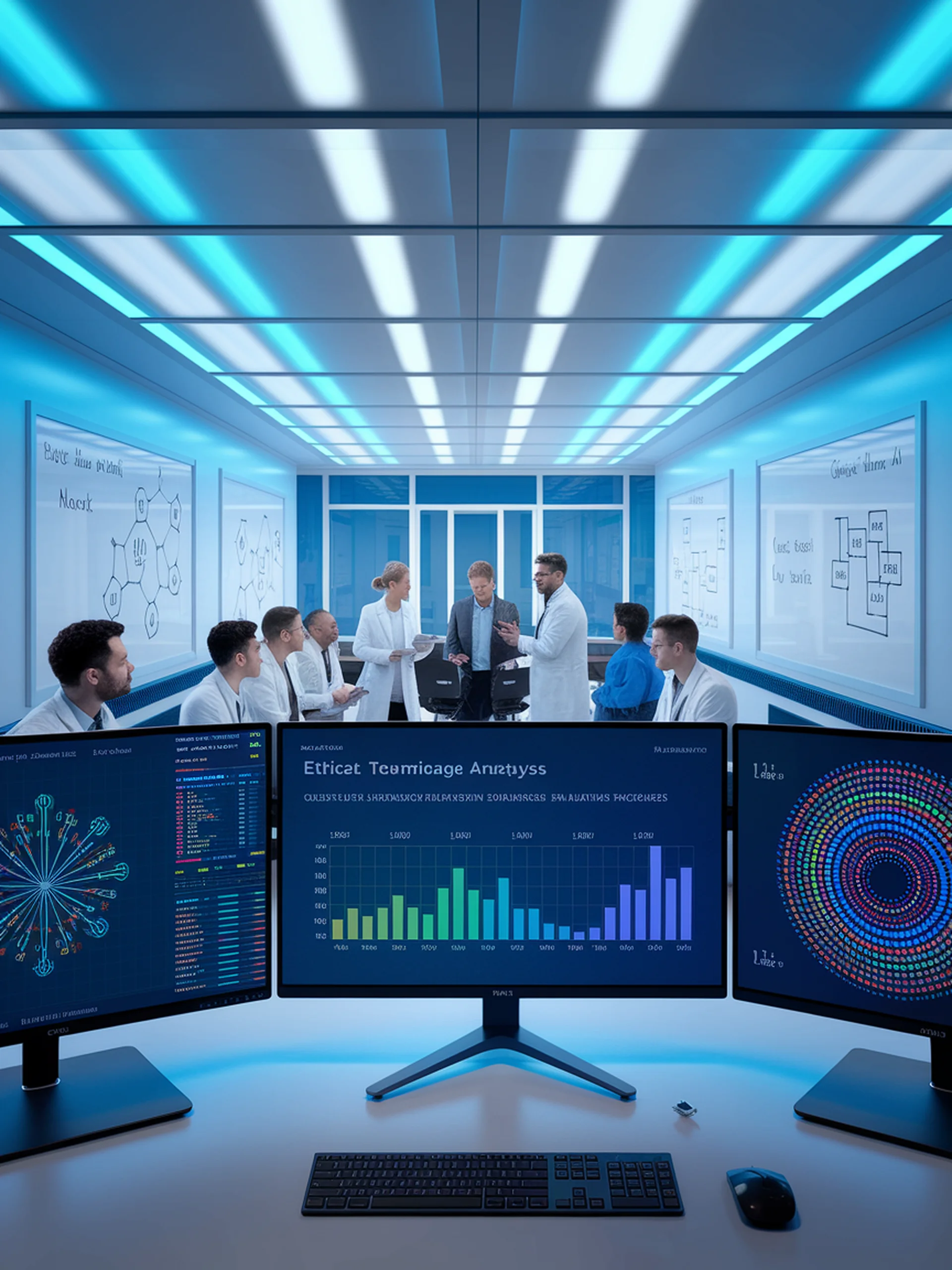
Evaluating AI Models for Inclusive Computing Language
Benchmarking LLMs' ability to detect harmful technical terminology
This research evaluates how effectively different language model architectures (encoder, decoder, and encoder-decoder) can identify harmful and non-inclusive terminology in technical computing contexts.
Key findings:
- Various model architectures (BERT, RoBERTa, GPT-4, Claude, Gemini) were evaluated against a curated database of technical terms
- Researchers assessed each model's effectiveness in detecting harmful language across specific use cases
- The work contributes to understanding how model design impacts harmful language detection capabilities
For security teams, this research provides valuable benchmarks for creating safer digital environments, enhancing content moderation, and supporting psychological safety in technical documentation and communications.