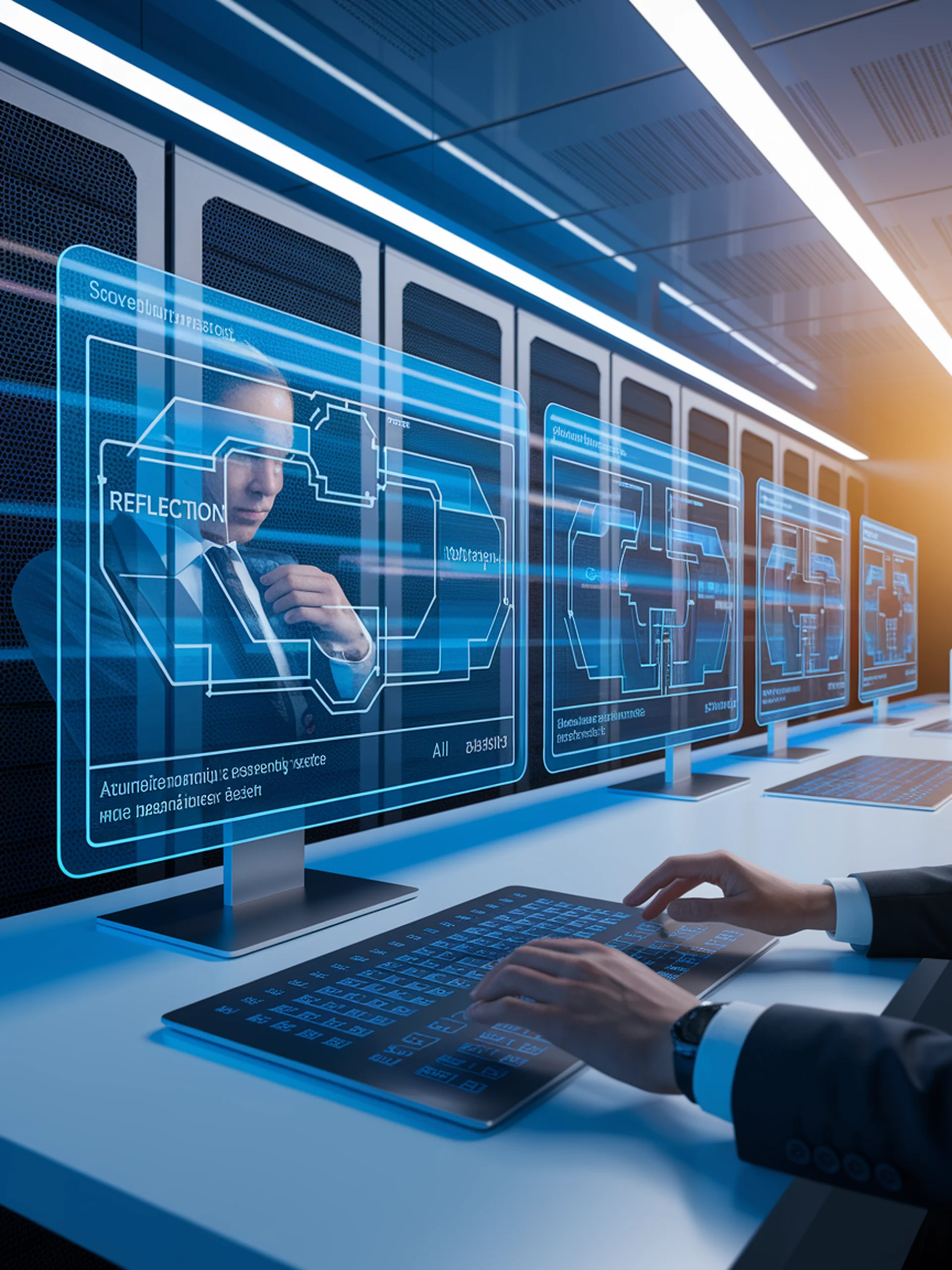
Fixing False Refusals in AI Safety
Making LLMs smarter about when to say 'no'
This research introduces a novel Think-Before-Refusal (TBR) approach that helps large language models distinguish between genuinely harmful requests and benign queries that contain trigger words.
- Reduces false refusal rates while maintaining safety guardrails
- Implements a reflection process before rejection decisions
- Demonstrates significant improvements across multiple LLMs including GPT-4
- Offers a simple but effective solution that doesn't require model retraining
For security professionals, this advancement represents a critical balance between maintaining protective guardrails while improving user experience by reducing frustrating false rejections.
Think Before Refusal: Triggering Safety Reflection in LLMs to Mitigate False Refusal Behavior