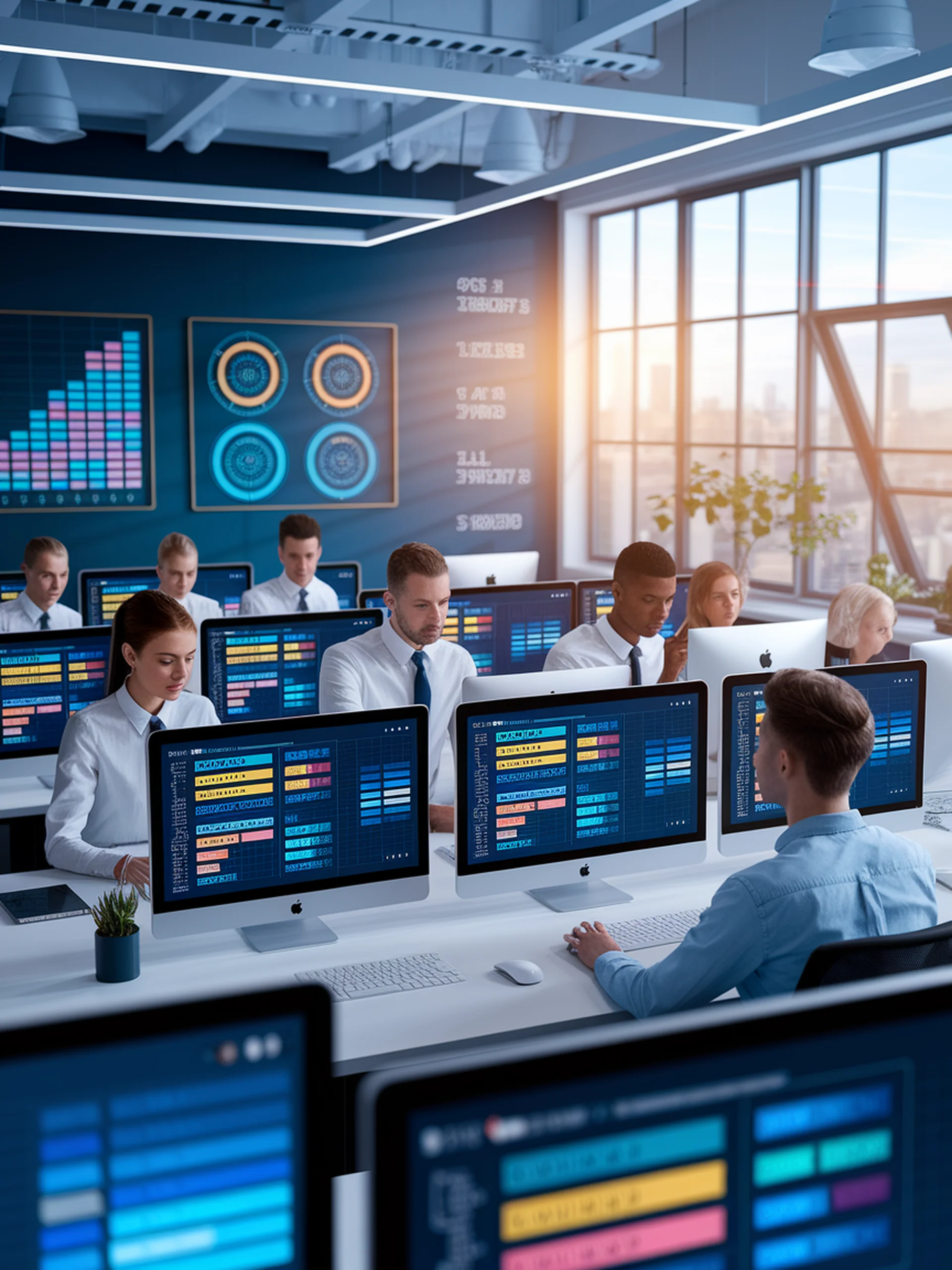
Multi-label Hate Speech Detection
Advancing beyond binary classification for more effective content moderation
This research advances hate speech detection by exploring multi-label classification approaches instead of traditional binary methods, better reflecting the nuanced nature of harmful content.
- Focuses on English-language hate speech requiring differentiated classification
- Surveys existing machine learning models and datasets for multi-label hate speech detection
- Provides a systematic framework for practitioners in content moderation and law enforcement
- Addresses critical security needs for protecting online communities and individuals
The multi-label approach significantly improves content moderation systems by enabling more nuanced responses to different types of harmful content, supporting more effective security measures across digital platforms.