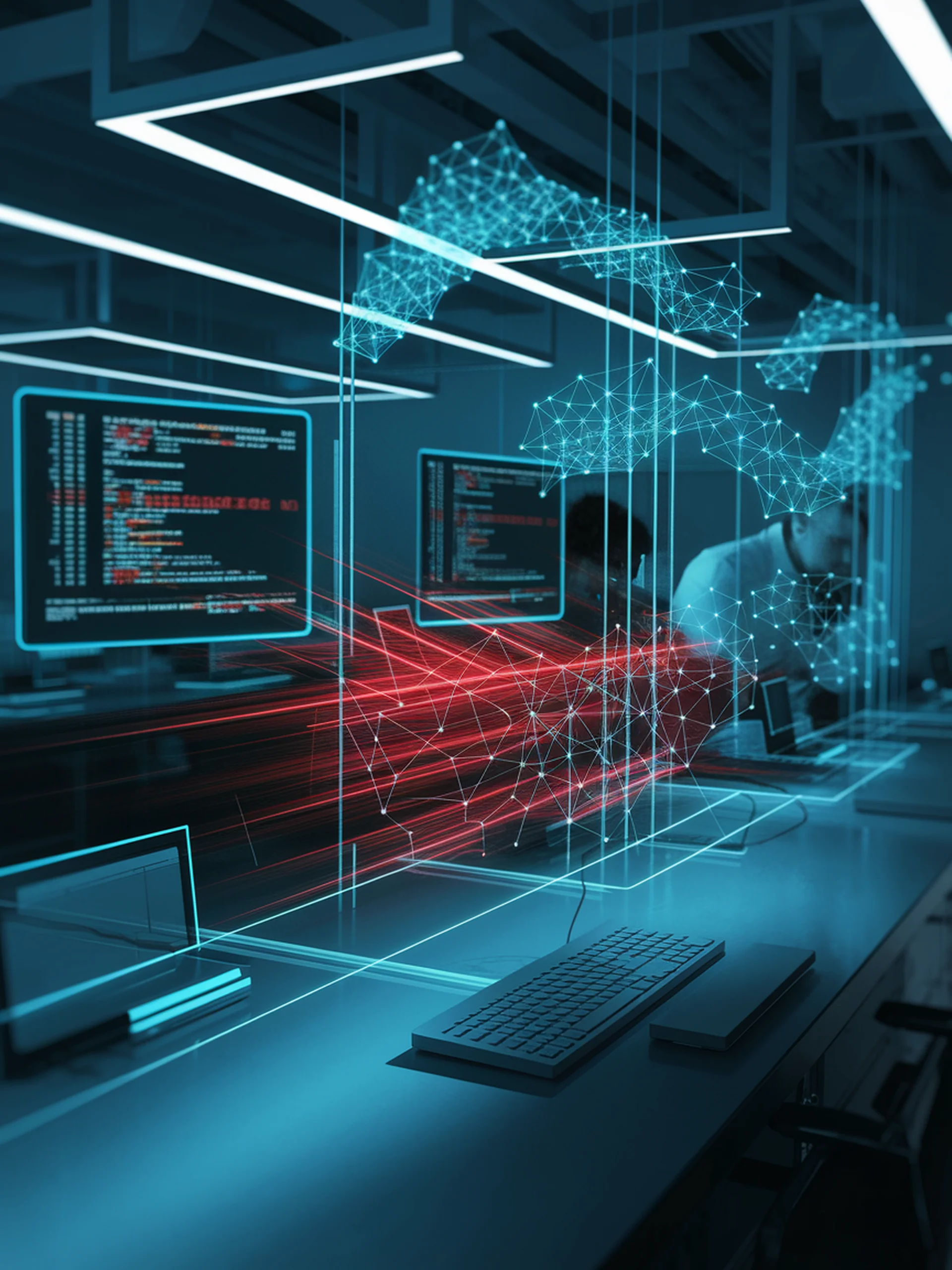
Hidden Attacks: The New Threat to AI Safety
Exploiting embedding spaces to bypass safety measures in open-source LLMs
Researchers discovered a novel attack vector that manipulates embedding spaces in open-source language models to circumvent safety mechanisms, revealing critical security implications.
- Successfully bypasses safety alignment training in popular open-source LLMs
- Embeds harmful instructions directly into the model's token embedding space
- Can override unlearning interventions, restoring previously removed capabilities
- Demonstrates the need for new security measures specifically designed for open-source AI models
This research highlights a concerning security gap as open-source models become more capable and widespread. Unlike traditional text-based attacks, these embedding-space manipulations are harder to detect and defend against, requiring new approaches to AI safety.