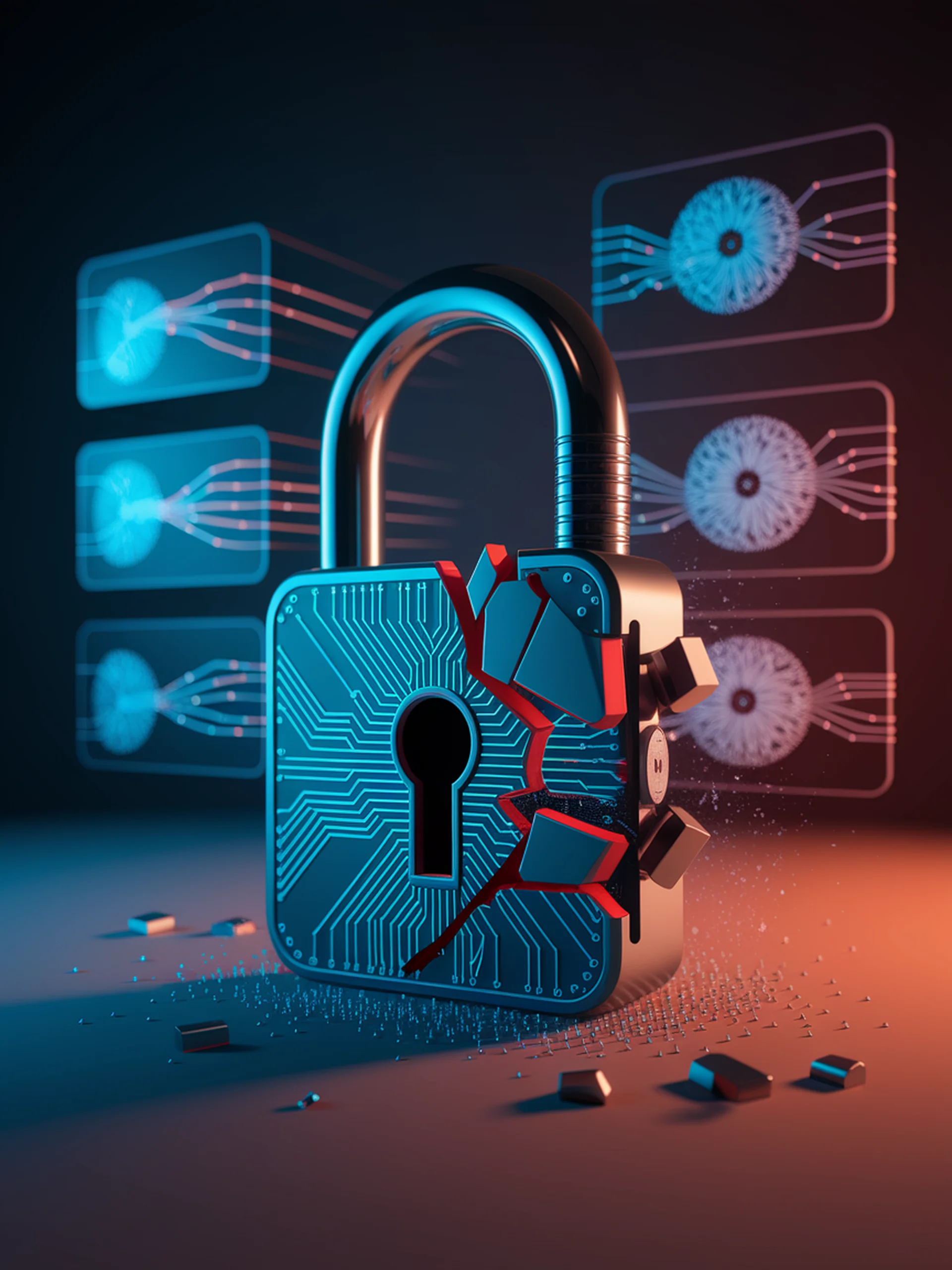
The Myth of Trigger Transferability
Challenging assumptions about adversarial attacks across language models
This research reveals that adversarial triggers optimized to jailbreak one large language model do not reliably transfer to other models, contrary to common belief.
- Extensive evaluation across 13 open-source LLMs shows poor and inconsistent transfer of attack triggers
- Transfer success rates ranged from only 0-30% between most model pairs
- Adversarial triggers appear to be more model-specific than previously assumed
- Even closely related models exhibited limited vulnerability to the same triggers
This work significantly impacts AI security by challenging the assumption that adversarial attacks generalize easily, suggesting that model-specific defenses may be more effective than universal countermeasures.
Investigating Adversarial Trigger Transfer in Large Language Models