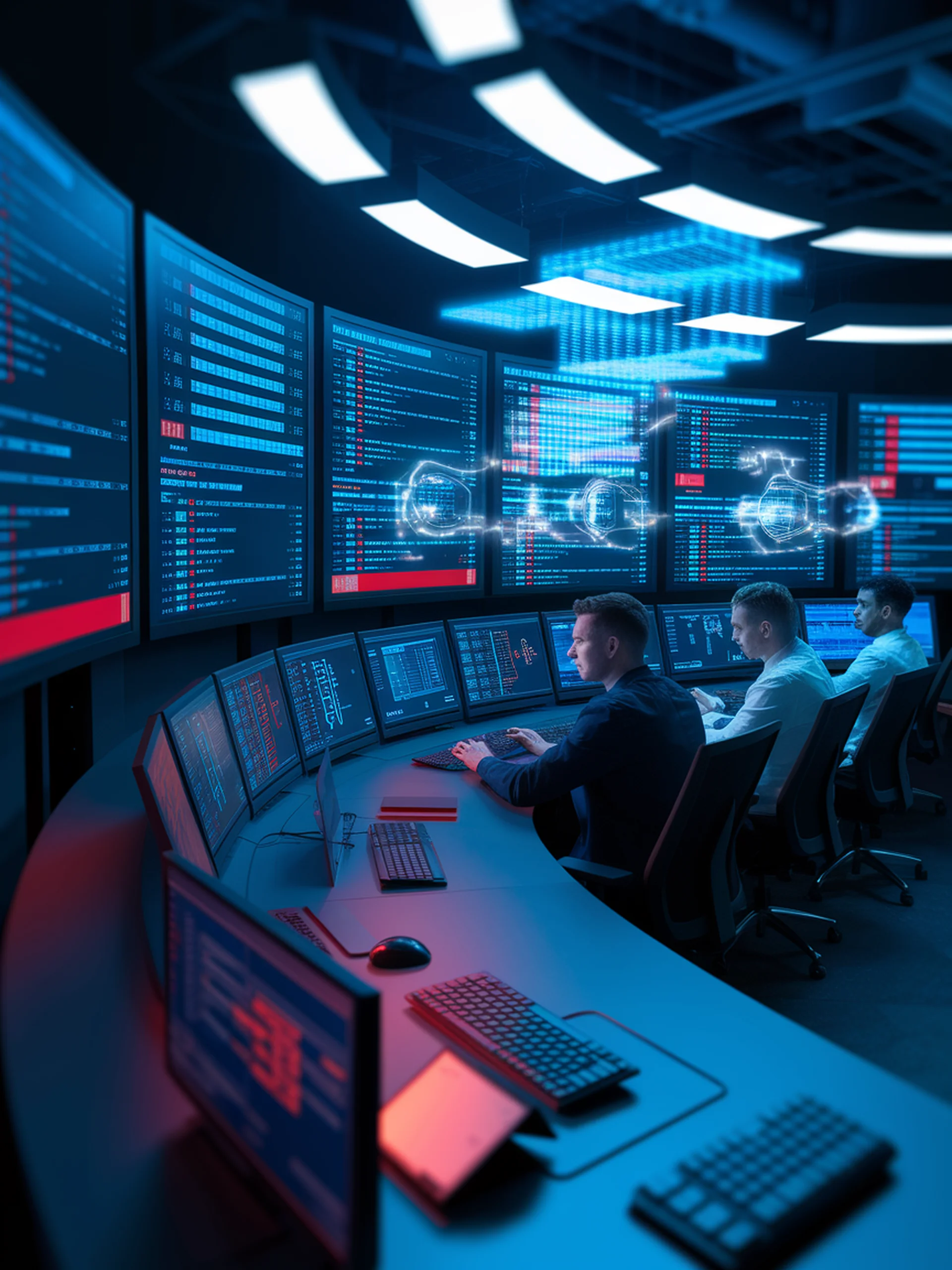
Supercharging LLM Security Testing
A novel approach for discovering diverse attack vectors
This research introduces a new framework for automated red-teaming that generates diverse attack prompts to identify vulnerabilities in large language models.
Key Findings:
- Develops a diversity-enhanced red-teaming technique that discovers a wider range of harmful prompts
- Leverages reinforcement learning to fine-tune attacker models that can expose LLM weaknesses
- Creates more comprehensive safety testing compared to traditional approaches
- Enables more robust protection against numerous attack vectors
This work is critical for AI security as it strengthens defenses against potential misuse, helping organizations build safer AI systems that resist manipulation while maintaining their utility. The research provides practical methods to identify and mitigate risks before deployment.
Learning diverse attacks on large language models for robust red-teaming and safety tuning