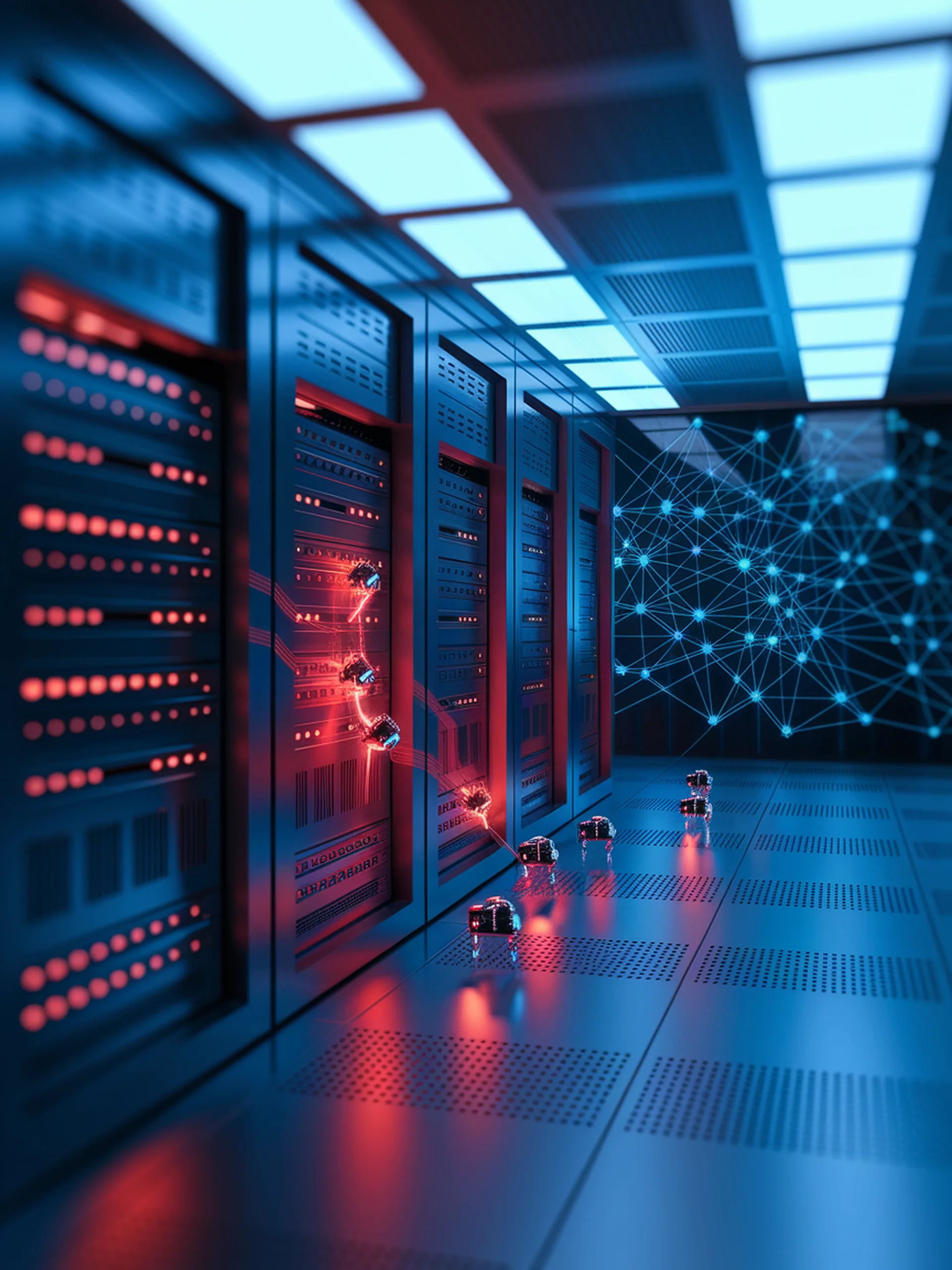
Breaking Through LLM Guardrails with SeqAR
Uncovering security vulnerabilities in large language models through sequential character prompting
This research introduces SeqAR, a novel framework for automatically generating jailbreak prompts that bypass LLM safety mechanisms by employing multiple malicious characters in sequence.
Key Findings:
- SeqAR successfully evades safety guardrails in state-of-the-art LLMs through sequential character deployment
- The approach reveals critical vulnerabilities in current safety alignment techniques
- Researchers adopt a red-teaming strategy to identify and help address these security weaknesses
- Findings demonstrate the need for more robust safety measures in LLM development
Security Implications: This work provides valuable insights for security teams to strengthen LLM defenses against sophisticated attacks, highlighting how sequential prompts can circumvent existing protections and where additional safeguards are needed.
SeqAR: Jailbreak LLMs with Sequential Auto-Generated Characters