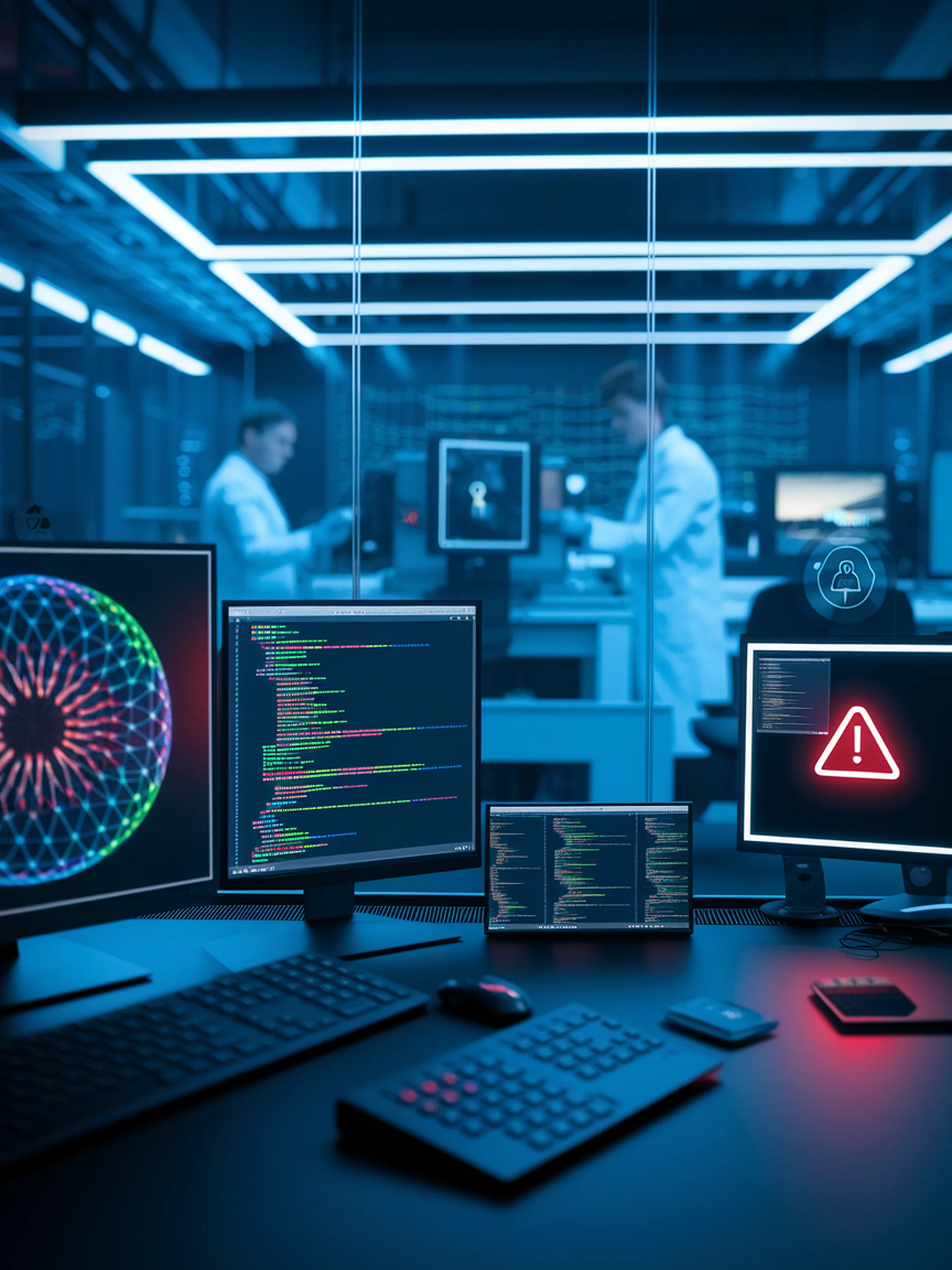
Exposing Biases in LLMs Through Adversarial Testing
How jailbreak prompts reveal hidden biases in seemingly safe models
This research demonstrates that even carefully trained large language models remain vulnerable to bias extraction through specialized adversarial techniques.
Key findings:
- LLMs can be manipulated to reveal underlying biases despite safety guardrails
- Models exhibit various biases including gender, ethnic, religious, and socioeconomic stereotypes
- Jailbreaking techniques successfully bypassed safety mechanisms in multiple commercial LLMs
- Security implications are significant as malicious actors could exploit these vulnerabilities
For security professionals, this work highlights critical weaknesses in current AI safety measures and demonstrates the need for more robust bias mitigation strategies beyond standard training practices.