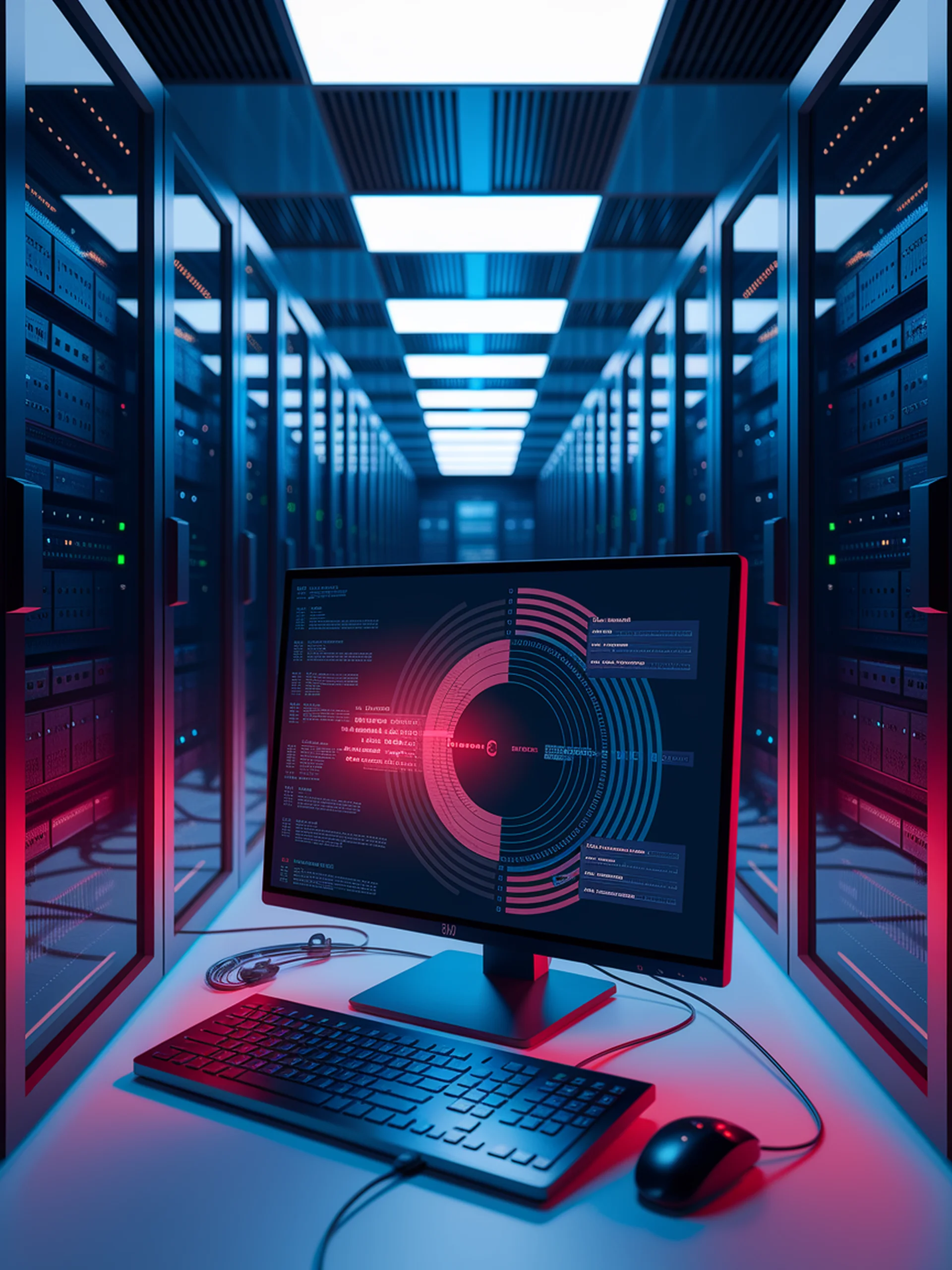
Defending LLMs Against Unsafe Feedback
Securing RLHF systems from harmful manipulation
This research explores a critical but overlooked vulnerability: how reinforcement learning from human feedback (RLHF) can be compromised by unsafe or malicious feedback inputs.
- Identifies novel risks in feedback collection systems widely deployed in production LLMs
- Evaluates effectiveness of different defense mechanisms against unsafe feedback
- Demonstrates that current safety guards can be undermined through manipulated feedback
- Proposes practical safeguards for more robust RLHF implementations
As organizations increasingly rely on user feedback to improve AI systems, this work provides essential guidance for security professionals to protect LLMs from being manipulated into producing harmful content through their training feedback loops.