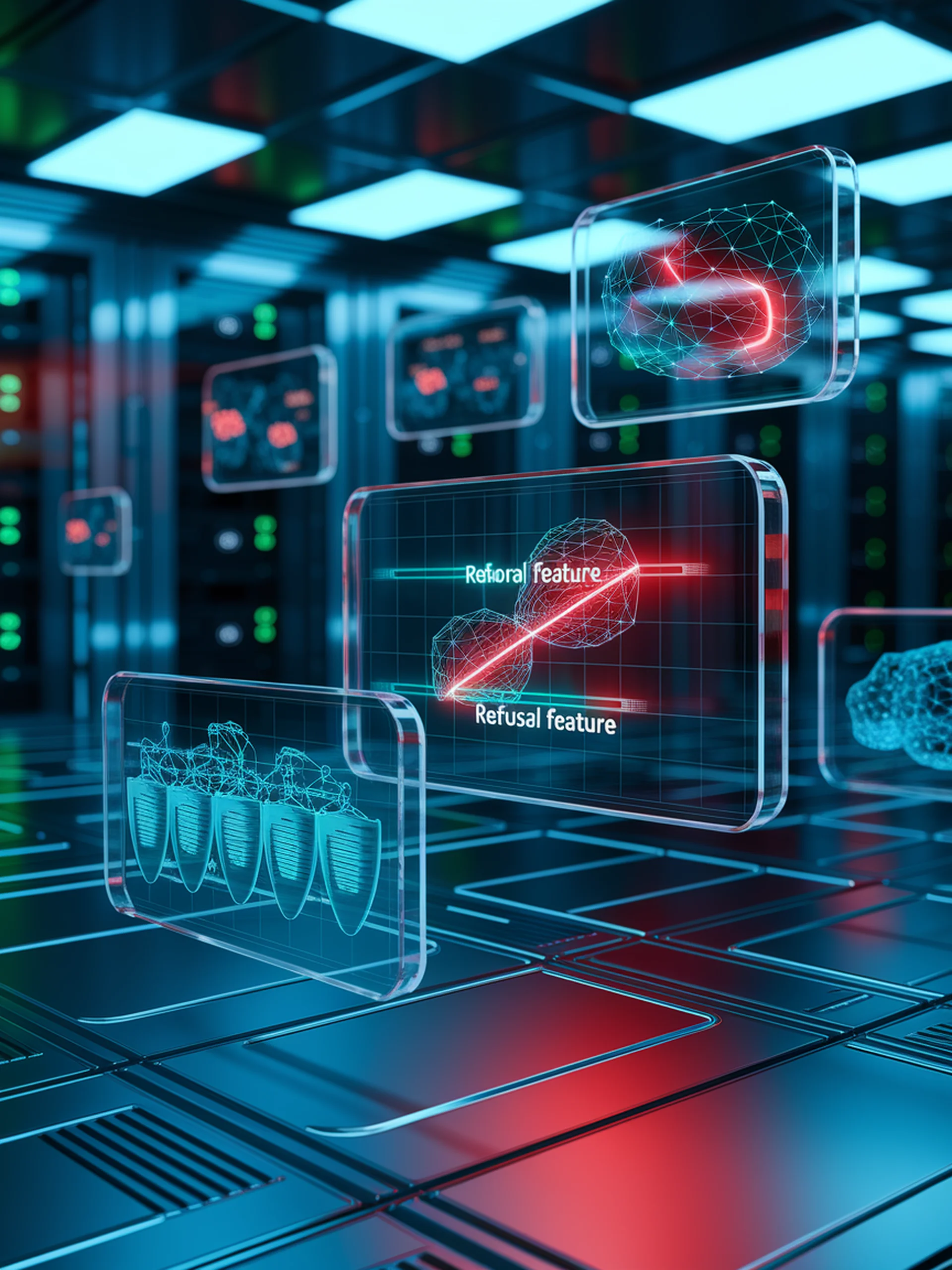
Defending LLMs Against Adversarial Attacks
Refusal Feature Adversarial Training (ReFAT) for Enhanced Safety
This research identifies how adversarial attacks bypass LLM safeguards through a common mechanism, and introduces a novel defensive approach.
- Discovers the universal mechanism behind jailbreaking attacks: the ablation of a specific dimension in embedding space called the refusal feature
- Introduces ReFAT (Refusal Feature Adversarial Training), a lightweight and efficient method to protect LLMs against harmful outputs
- Demonstrates significant improvements in robustness while maintaining model performance and alignment
- Provides a practical solution that addresses the opacity of jailbreaking mechanisms with low computational costs
This research matters for security teams by offering a fundamental understanding of how attacks work and providing an efficient safeguarding method that can be implemented without extensive retraining of large language models.
Robust LLM safeguarding via refusal feature adversarial training