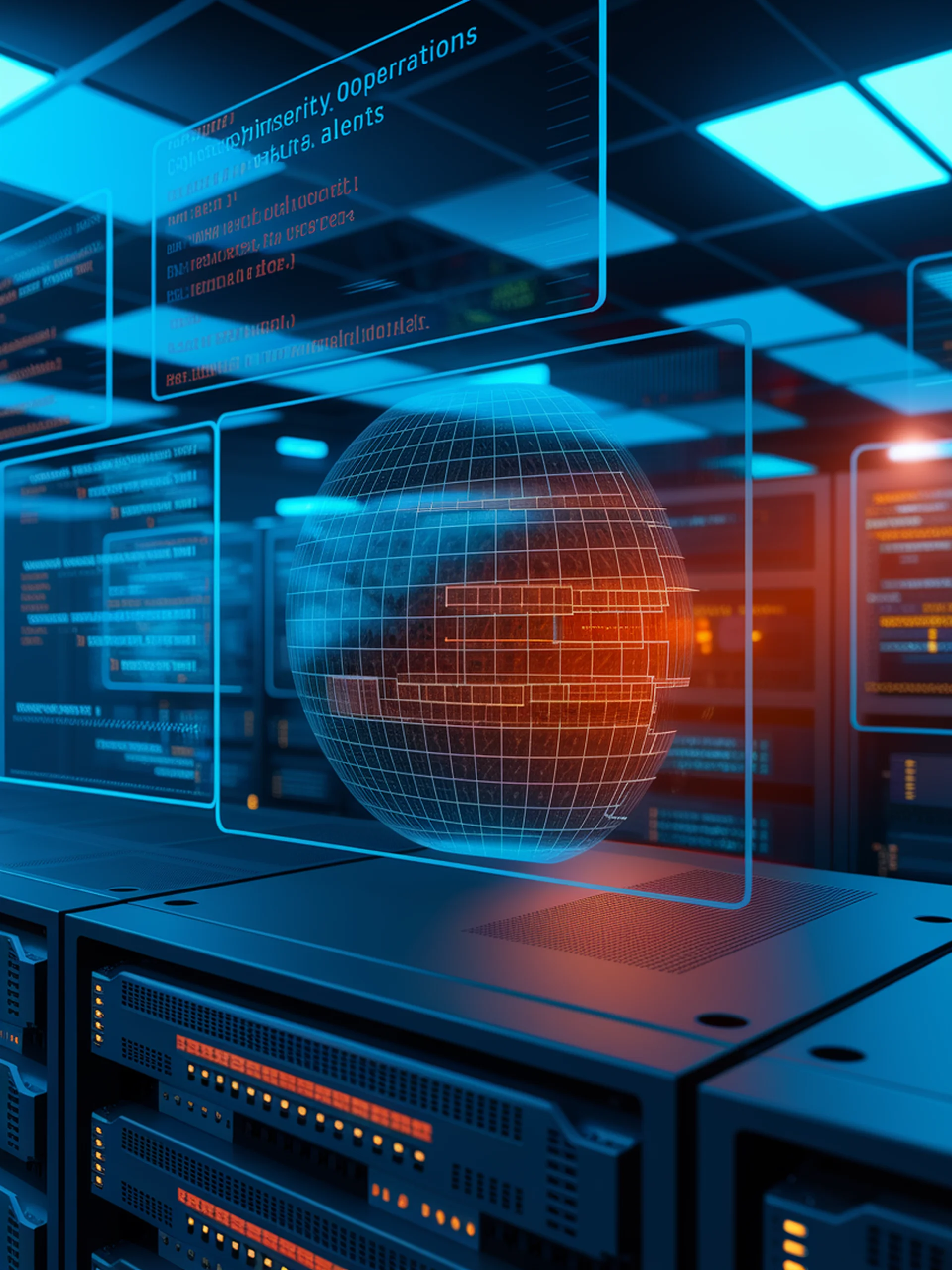
Defending Against Jailbreak Attacks in LLMs
A novel layer-level approach for enhancing AI safety
Layer-AdvPatcher introduces an innovative defense methodology that protects Large Language Models from malicious jailbreak attacks while maintaining normal performance.
- Implements an unlearning strategy at specific layers of the neural network
- Utilizes self-exposure techniques to identify vulnerable components
- Applies targeted patches to neutralize attack vectors without comprehensive retraining
- Achieves significant security improvements with minimal impact on general performance
This research addresses critical security concerns as LLMs are increasingly deployed in business applications, providing a practical approach to safeguarding AI systems against exploitation while preserving their utility.
Layer-Level Self-Exposure and Patch: Affirmative Token Mitigation for Jailbreak Attack Defense