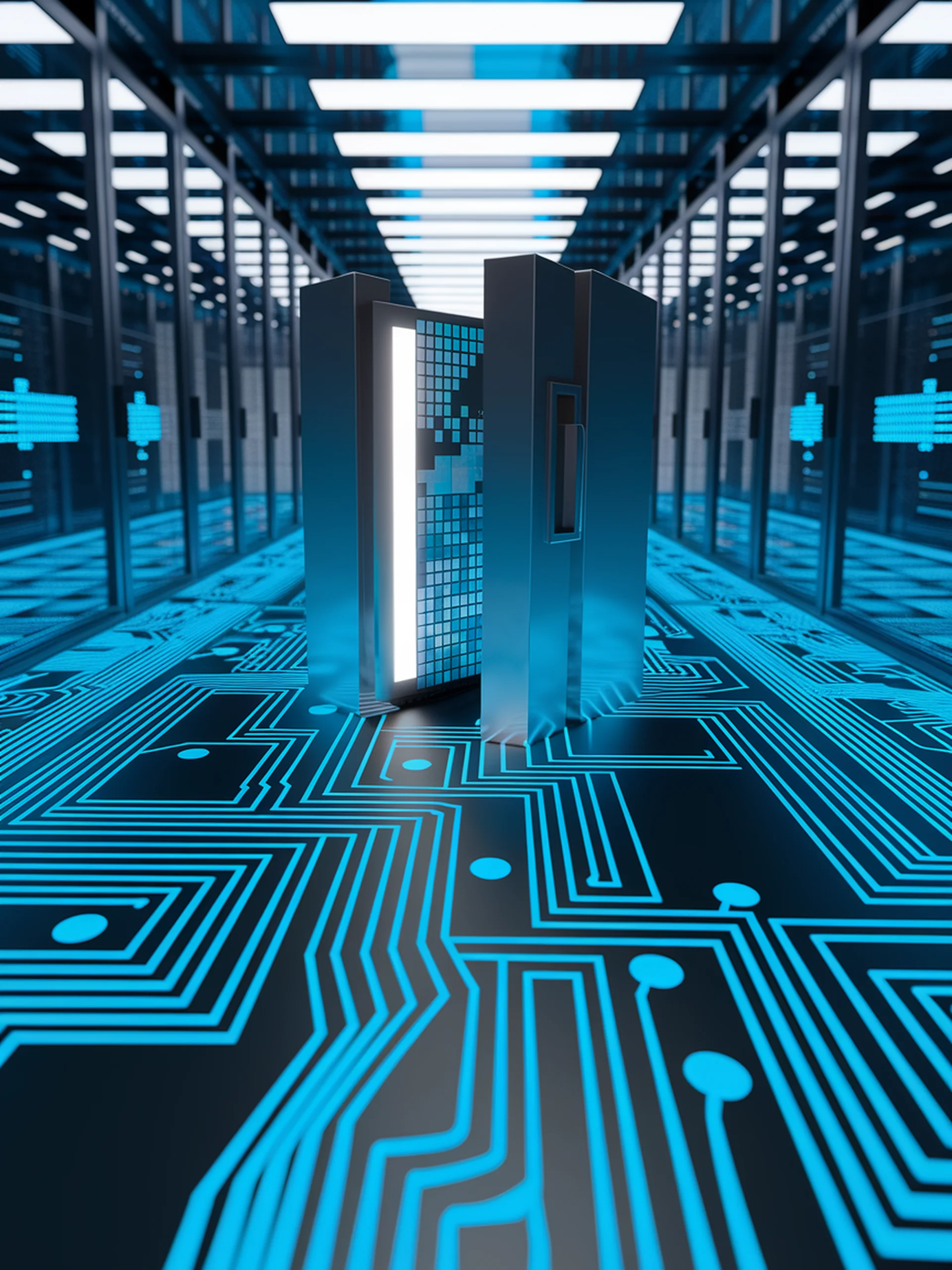
Strengthening LLM Safety Through Backtracking
A novel safety mechanism that intercepts harmful content after generation begins
Backtracking offers a new approach to LLM safety that monitors and corrects harmful content even after generation has started, addressing vulnerabilities in current safety methods.
- Identifies limitations in existing safety techniques that only prevent harmful outputs in initial tokens
- Introduces a monitoring system that detects toxic language during generation and triggers backtracking
- Demonstrates significant reduction in harmful outputs while maintaining model performance
- Works as a complementary layer alongside existing safety alignment methods
This research provides a critical advancement for secure AI deployment by creating more robust safeguards against adversarial attacks and harmful content generation - essential for business applications where trust and safety are paramount.