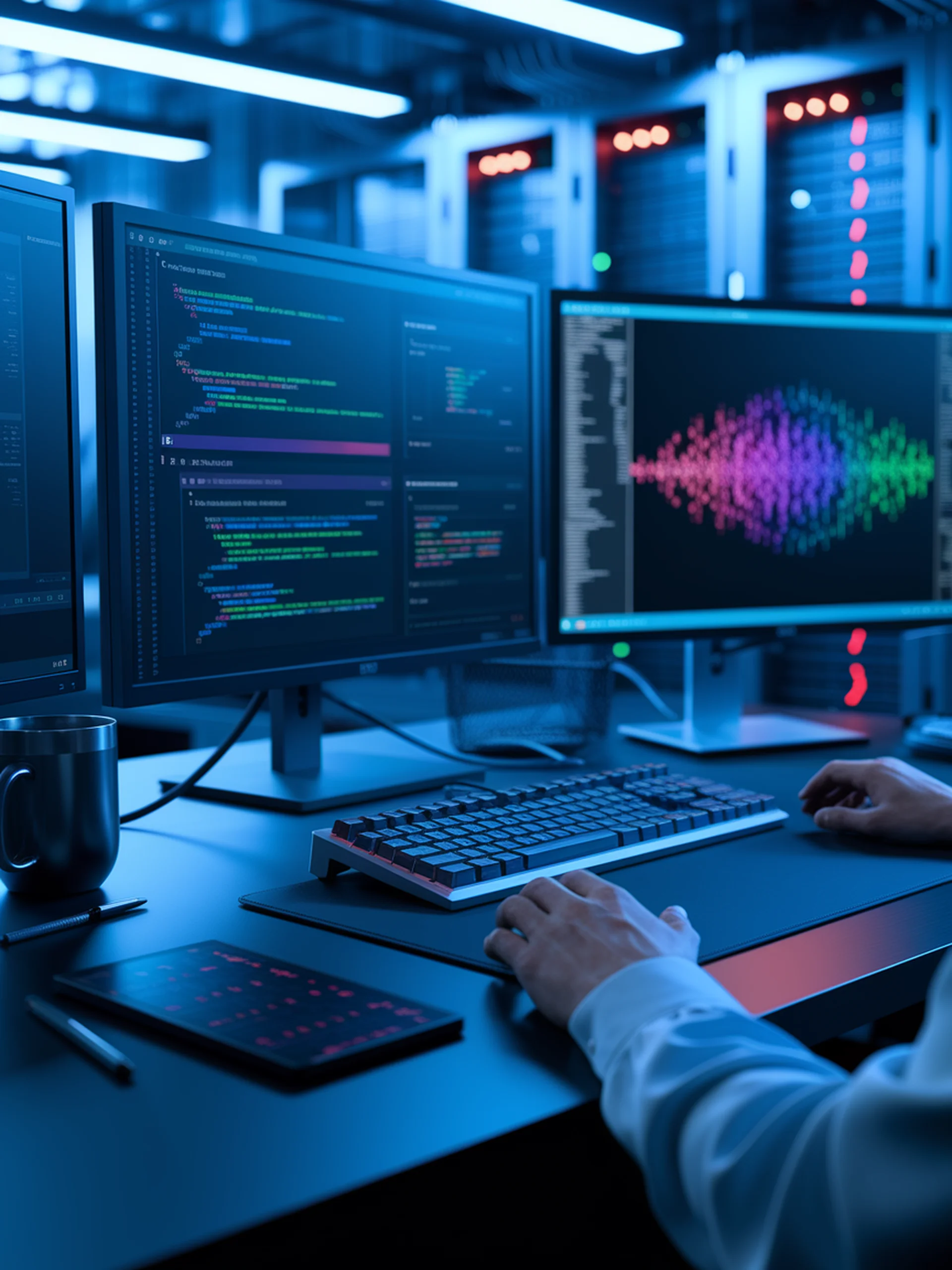
Jailbreaking LLMs Through Fuzzing
A new efficient approach to detecting AI security vulnerabilities
JBFuzz introduces a novel fuzzing-based technique to systematically identify jailbreak vulnerabilities in large language models.
- Uses mutation-based fuzzing to generate and refine jailbreak prompts
- Outperforms existing methods in both effectiveness and efficiency
- Enables proactive security testing by revealing potential safety bypasses
- Demonstrates the need for robust red-teaming tools in LLM deployment
This research highlights critical security concerns as LLMs become more widespread, providing a practical approach for developers to identify and patch vulnerabilities before deployment in production environments.
JBFuzz: Jailbreaking LLMs Efficiently and Effectively Using Fuzzing