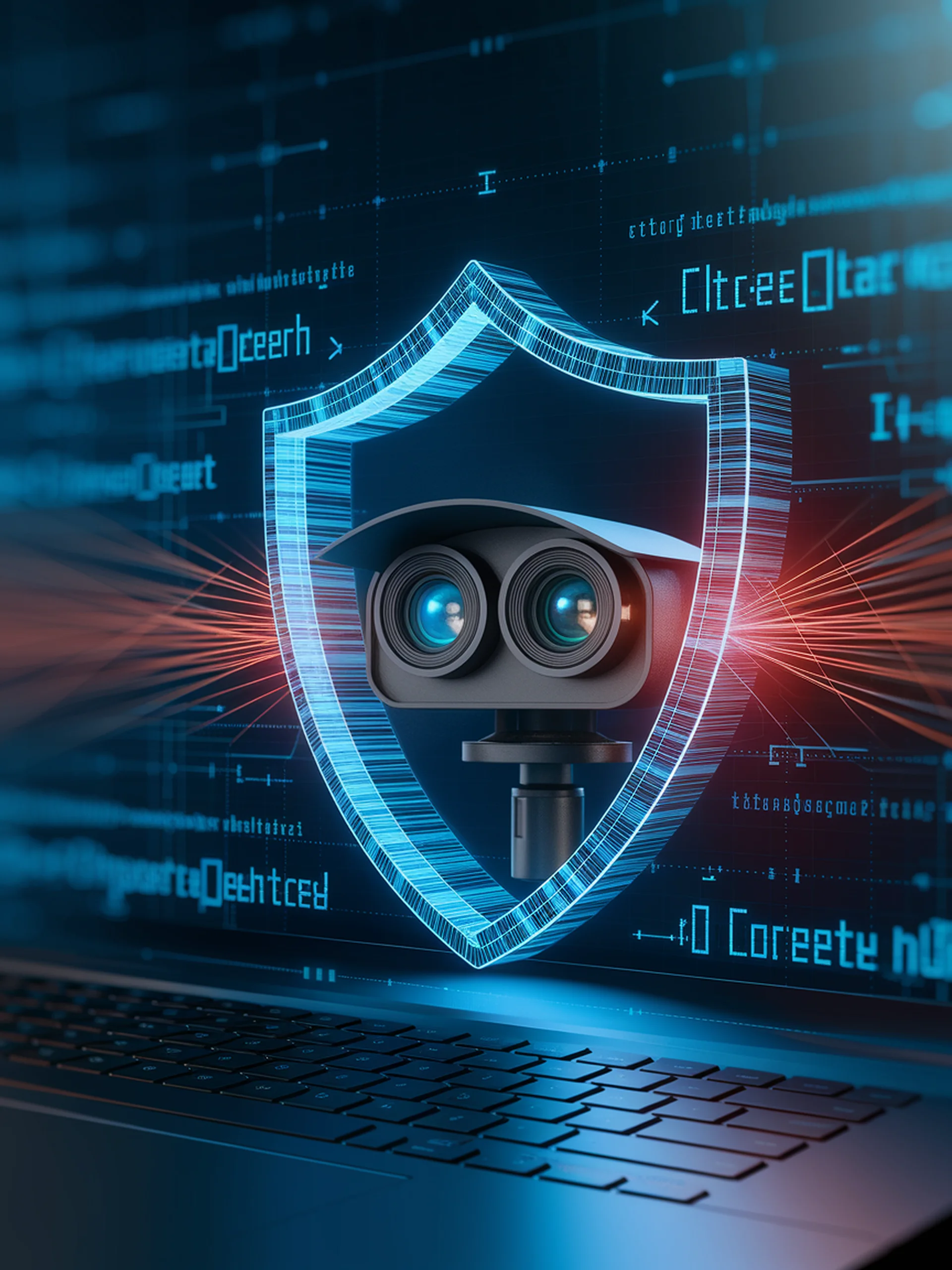
Certified Defense for Vision-Language Models
A new framework to protect AI models from visual jailbreak attacks
This research introduces CeTAD, a novel certified defense framework that provides mathematical guarantees against visual jailbreak attacks on vision-language models.
- Proposes a toxicity-aware distance metric to measure semantic differences between harmful and safe responses
- Develops a certification procedure that ensures robustness against adversarial visual perturbations
- Delivers formal security guarantees rather than just empirical defenses
- Addresses a critical vulnerability in multimodal AI systems as they become more widespread
This work represents a significant advancement for enterprise security teams deploying vision-language models, offering provable protection against emerging attack vectors that could otherwise bypass content moderation systems.
CeTAD: Towards Certified Toxicity-Aware Distance in Vision Language Models