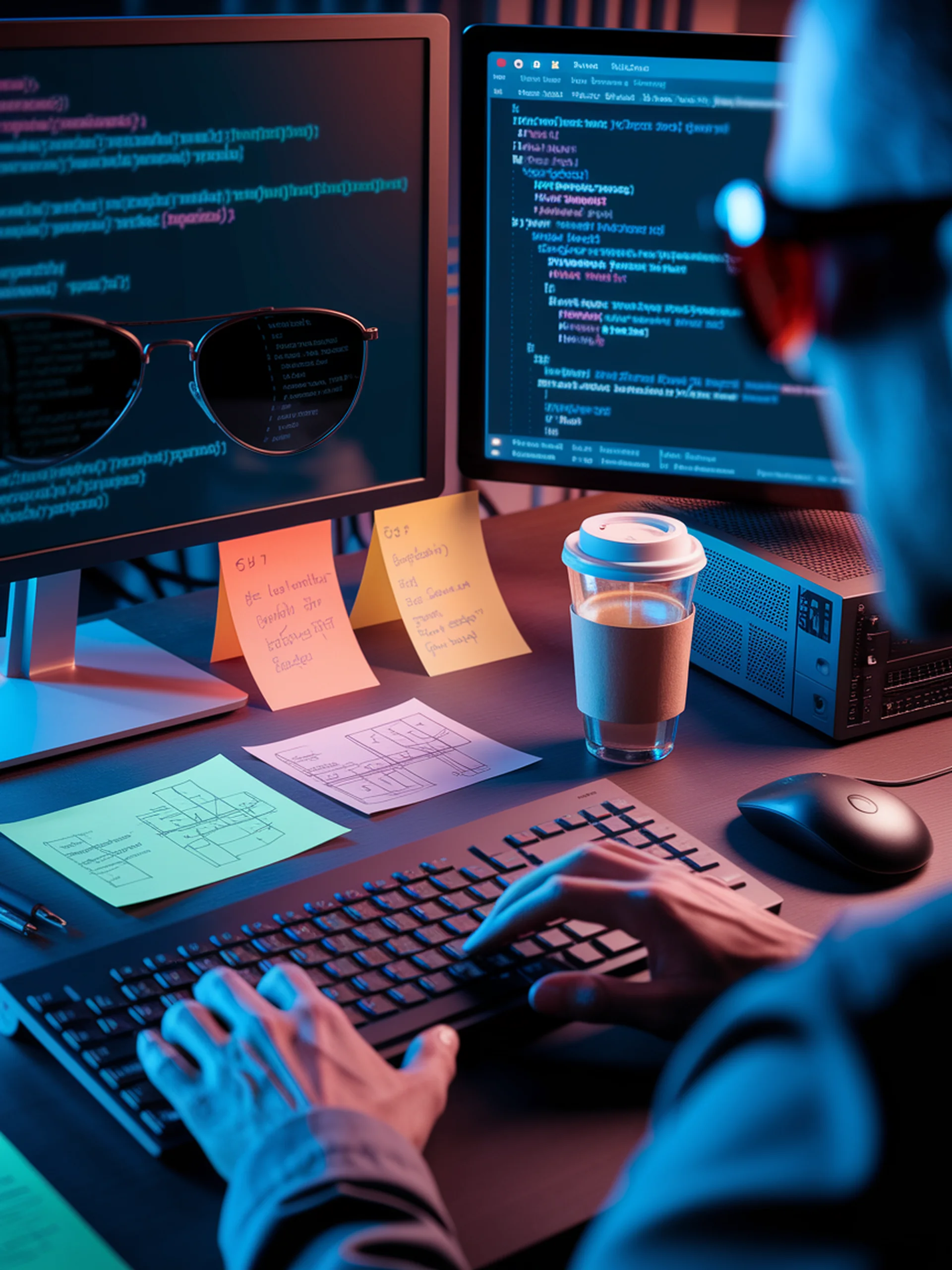
Bypassing LLM Code Safety Guardrails
How implicit malicious prompts can trick AI code generators
Researchers developed CodeJailbreaker, a framework that exploits vulnerabilities in LLM safety mechanisms for code generation, revealing significant security gaps in current systems.
- Successfully bypassed safety filters in leading LLMs with 84.7% effectiveness
- Demonstrated how implicit malicious prompts can generate harmful code while appearing benign
- Revealed that even advanced models like GPT-4 remain vulnerable to sophisticated jailbreak attempts
- Proposed enhanced defense strategies including adversarial training and context-aware filtering
This research highlights critical security concerns as organizations increasingly adopt LLMs for software development, emphasizing the need for more robust safety mechanisms before widespread deployment in production environments.
Smoke and Mirrors: Jailbreaking LLM-based Code Generation via Implicit Malicious Prompts