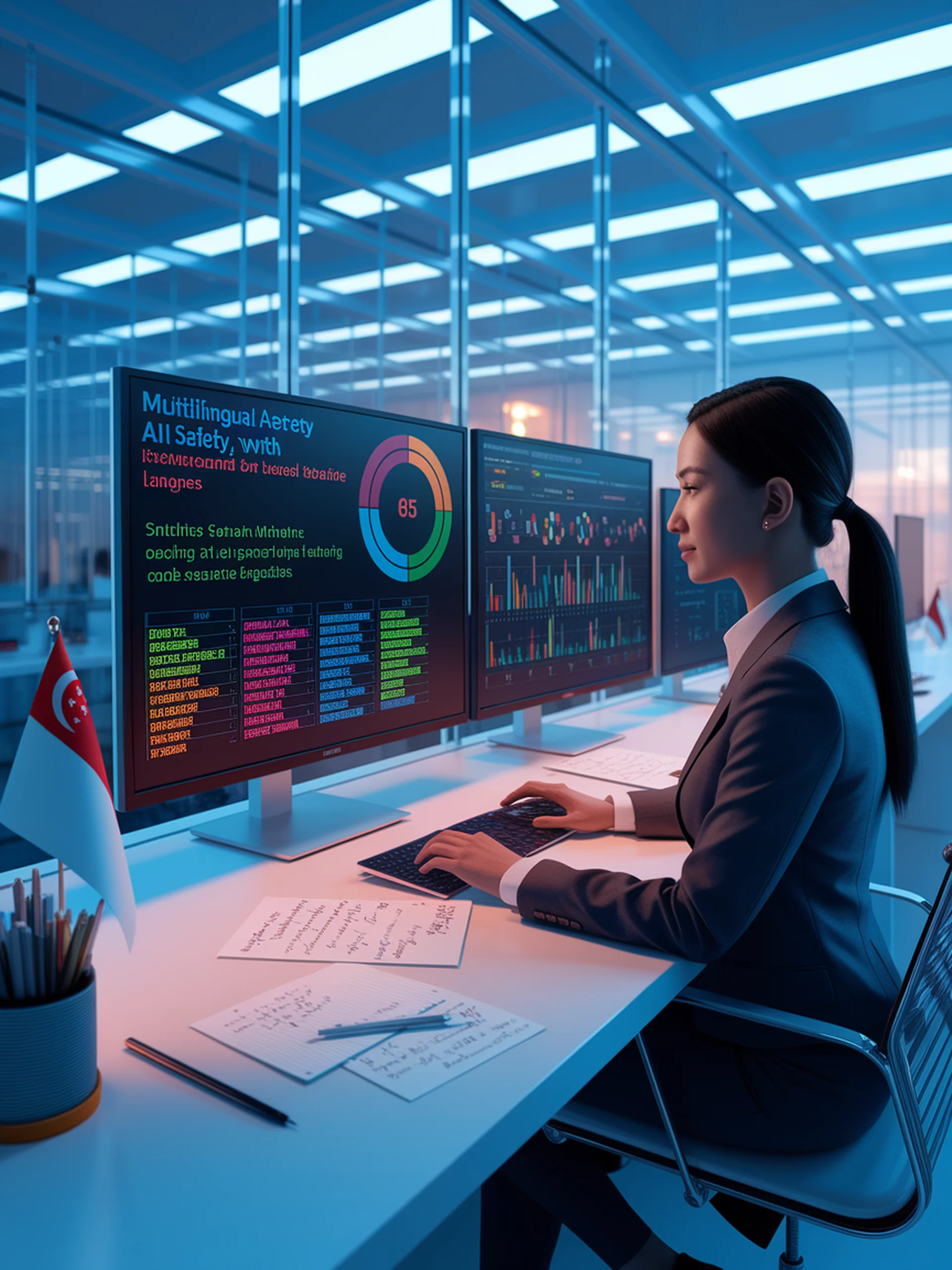
Making LLMs Safe in All Languages
Novel safety alignment for low-resource languages like Singlish
This research addresses the critical gap in safety alignment for non-standard English varieties and low-resource languages, using Singlish as a case study.
- Compared three alignment methods (SFT, DPO, and KTO) for reducing toxic responses
- Found that SFT+KTO combination achieved superior results in reducing harmful outputs
- Demonstrated effectiveness of targeted safety alignment for languages beyond standard English
- Proposed a generalizable framework for other low-resource languages
This work matters for security as it helps ensure AI systems remain safe when deployed in diverse linguistic contexts, reducing potential harm to underrepresented language communities.