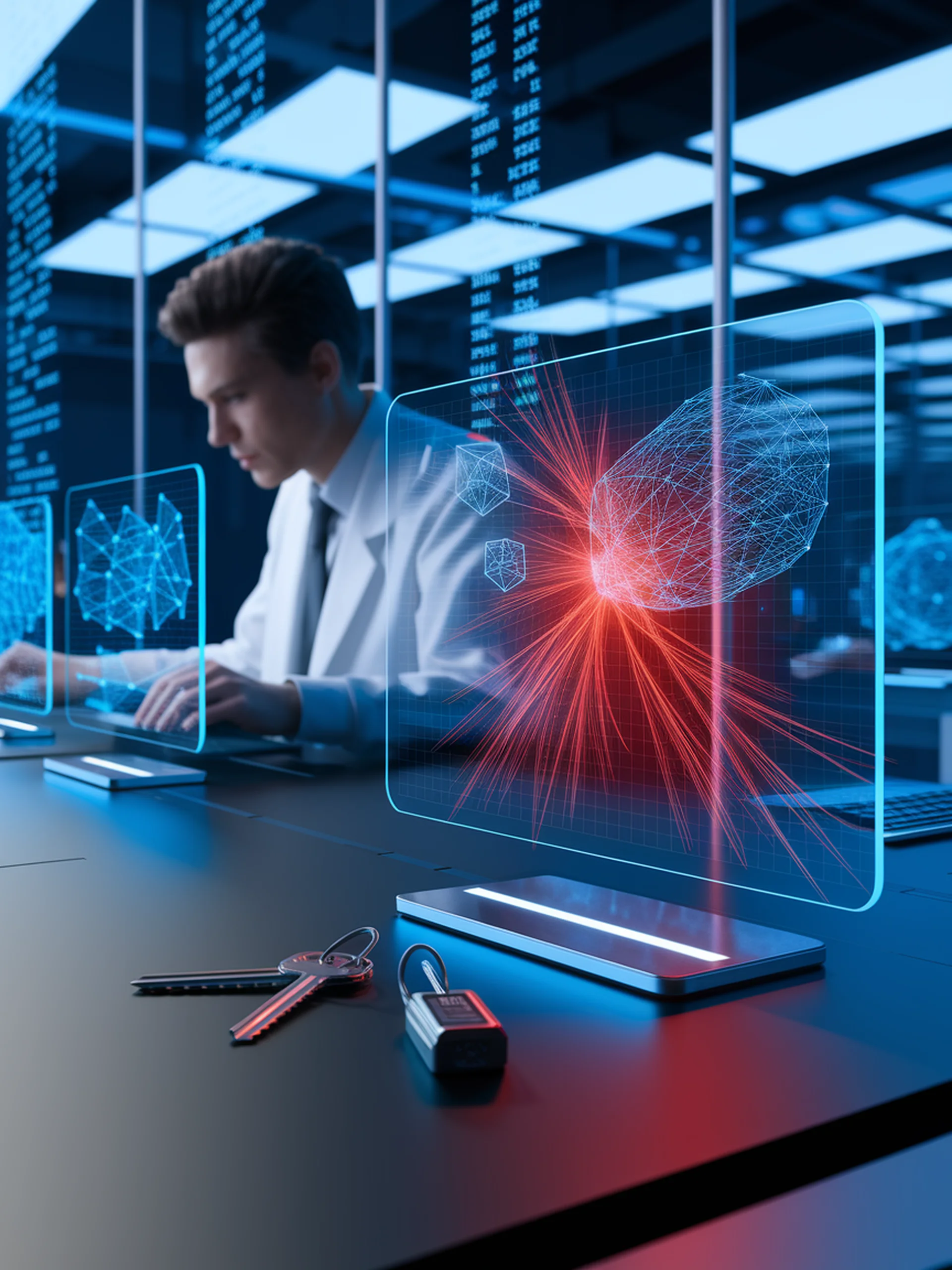
Strengthening LLM Unlearning Security
Making Representation Misdirection methods robust against backdoor-like vulnerabilities
This research identifies and addresses critical vulnerabilities in current LLM unlearning techniques, revealing how even a single forget-token can compromise system security.
- Demonstrates how Representation Misdirection (RM) unlearning methods can be reframed as backdoor attacks, where forget-tokens act as triggers
- Identifies that current approaches significantly reduce model robustness in practical scenarios
- Proposes Random Noise Augmentation to mitigate vulnerabilities while maintaining unlearning effectiveness
- Provides empirical validation across multiple models and datasets
For security professionals, this work offers crucial insights into making LLM unlearning methods more reliable and resistant to manipulation in real-world deployments.
Improving the Robustness of Representation Misdirection for Large Language Model Unlearning