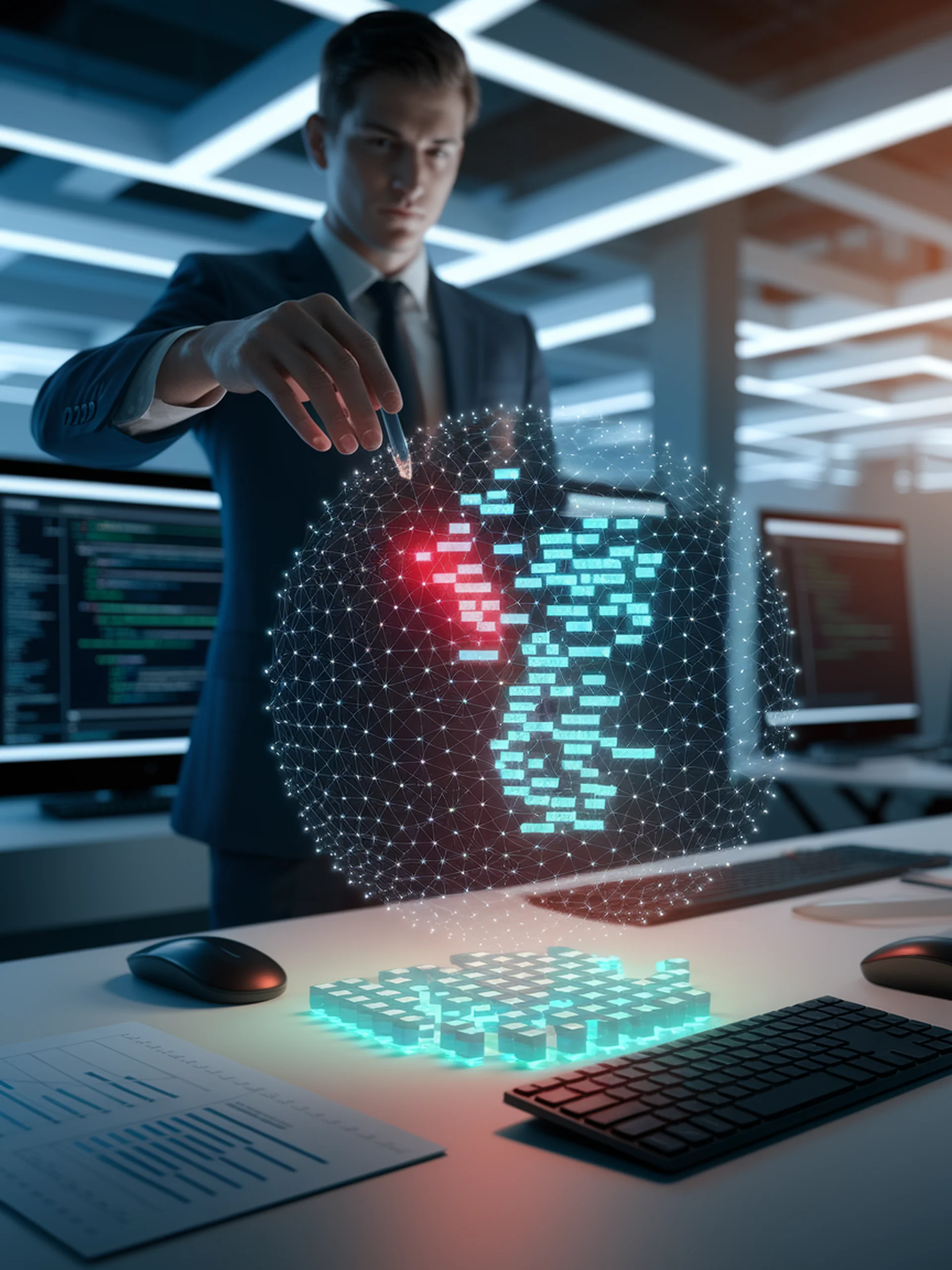
Balanced Data Forgetting in LLMs
Selective unlearning without compromising model utility
UPCORE introduces a novel approach for selectively removing data from trained language models while minimizing performance degradation on remaining data.
- Creates utility-preserving coresets that balance effective data removal with maintained model performance
- Addresses legal and user privacy requirements for data deletion from trained models
- Demonstrates superior unlearning efficiency compared to existing methods
- Provides a practical framework for responsible AI deployment in regulated environments
This research is critical for security teams who must comply with privacy regulations while preserving model functionality, enabling organizations to honor removal requests without rebuilding models from scratch.
UPCORE: Utility-Preserving Coreset Selection for Balanced Unlearning