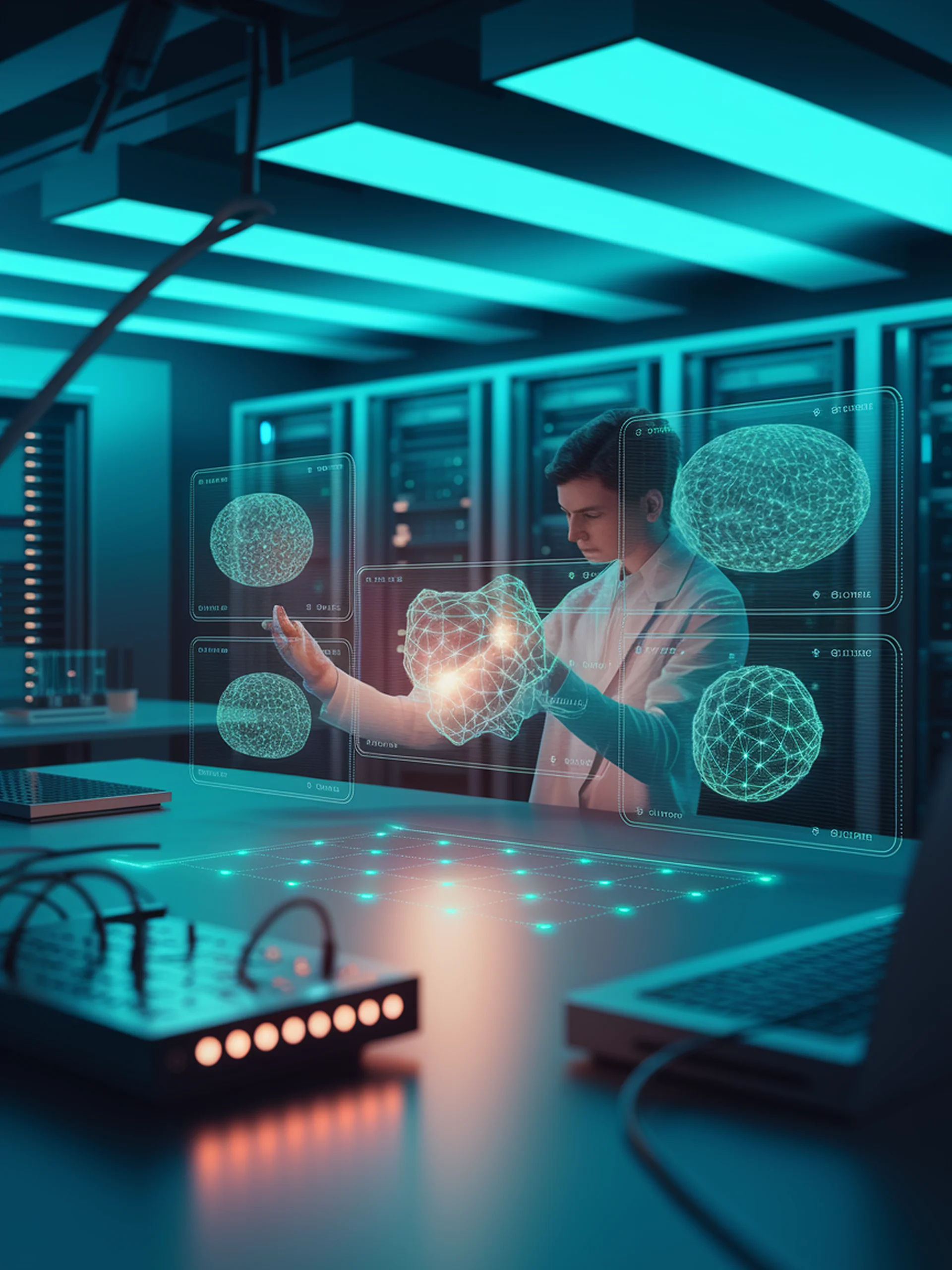
Selective Knowledge Erasure in LLMs
Advancing Security Through Model Merging Techniques
This research introduces a novel approach to selectively remove sensitive information from large language models while preserving overall model utility.
- Leverages TIES-Merging to combine specialized models into a balanced unlearned model
- Addresses both over-forgetting and under-forgetting challenges in LLM unlearning
- Achieved competitive results, ranking 2nd among 26 teams in the SemEval-2025 unlearning task
- Demonstrates practical applications for enhancing data privacy and security compliance
This innovation provides organizations with techniques to mitigate privacy risks in deployed AI systems while maintaining performance, potentially helping meet regulatory requirements for managing sensitive information in AI models.
ZJUKLAB at SemEval-2025 Task 4: Unlearning via Model Merging