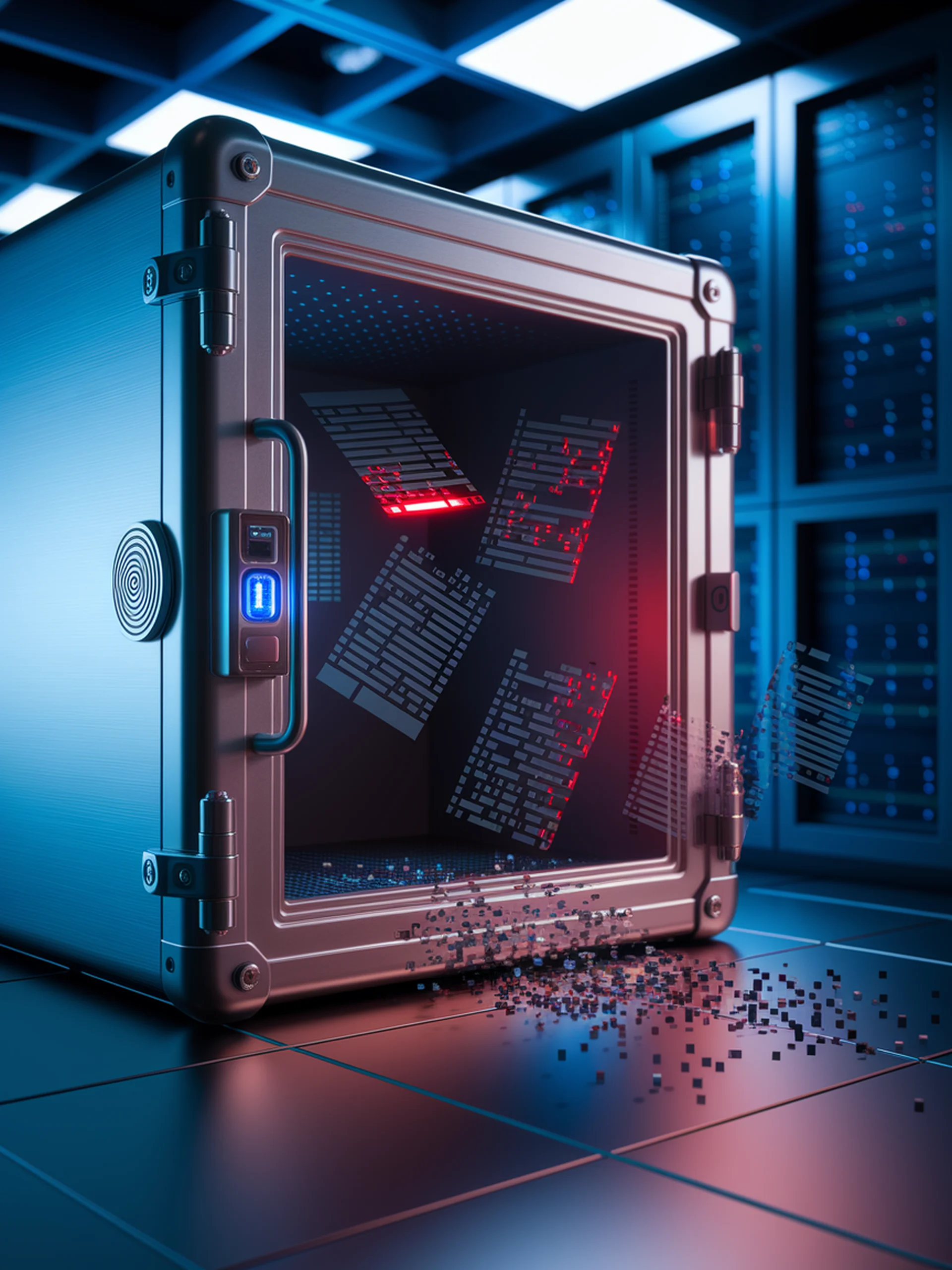
Erasing Sensitive Data from LLMs
A Systematic Approach to Making AI Forget
SemEval-2025 Task 4 introduces standardized challenges for unlearning sensitive content from Large Language Models (LLMs), addressing critical security and privacy concerns.
- Three Targeted Subtasks: Unlearning long-form creative content, synthetic biographies containing PII, and real documents from training datasets
- Security-Focused Design: Specifically targets removal of personally identifiable information including names, SSNs, phone numbers and addresses
- Comprehensive Evaluation: Provides structured benchmarks to measure unlearning effectiveness across different content types
- Practical Applications: Enables safer AI deployment by giving organizations techniques to remove sensitive information post-training
This research is crucial for security professionals as it offers systematic methods to protect privacy while maintaining model utility, addressing growing concerns about data exposure in AI systems.
SemEval-2025 Task 4: Unlearning sensitive content from Large Language Models