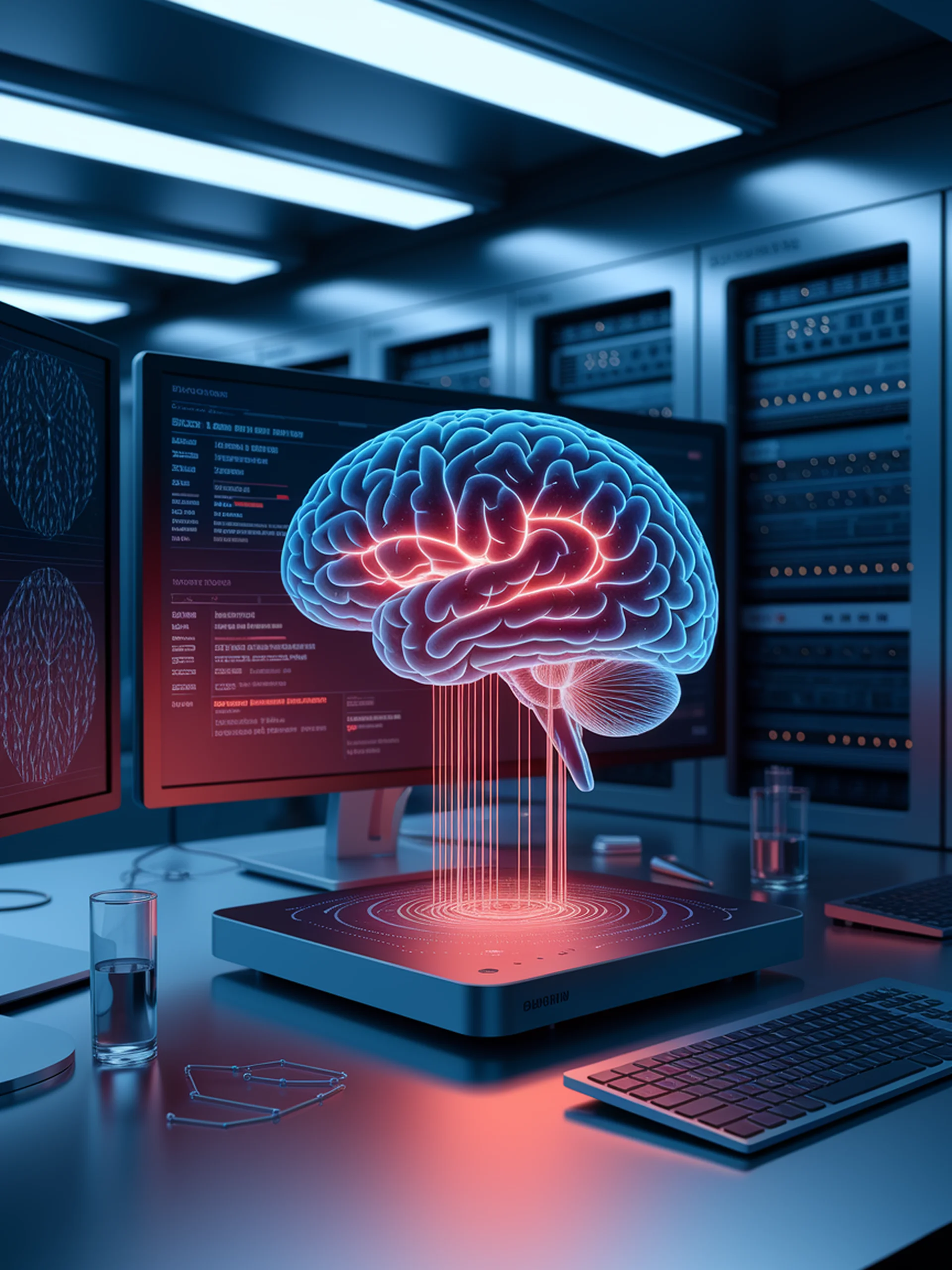
Selective Memory Wiping for AI
Precision-targeted unlearning keeps LLMs both safe and smart
This research introduces a novel two-stage methodology for selectively removing sensitive information from large language models without compromising their overall capabilities.
- Combines causal mediation analysis with layer-specific optimization
- Enables targeted removal of specific data associations
- Maintains model performance on general tasks
- Addresses critical privacy and security concerns for public AI deployment
This advancement matters for security professionals as it provides a practical solution to one of the major barriers to safe AI deployment - the ability to selectively "forget" sensitive data without degrading model utility.