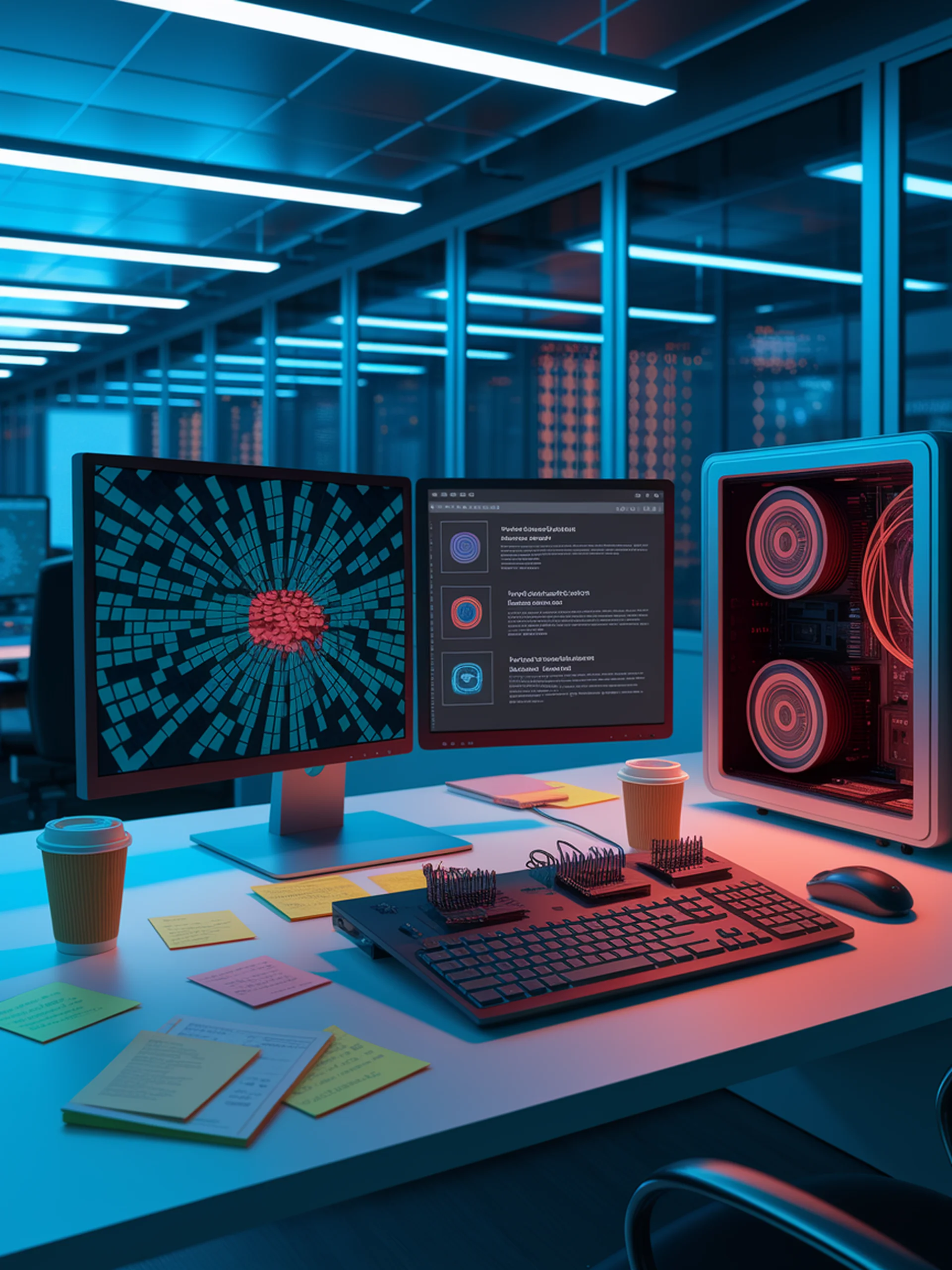
Exposing Vulnerabilities in AI Recommender Systems
How Memory Perturbation Attacks Undermine Agent-Based Recommenders
This research reveals critical security vulnerabilities in language model-based agent recommender systems by intentionally manipulating their memory components.
- Introduces novel techniques to attack agent memory through strategic perturbations that significantly degrade recommendation quality
- Demonstrates how compromised memory can lead to biased recommendations and loss of personalization
- Establishes a framework for evaluating and enhancing robustness of AI agent systems
This work is crucial for organizations deploying AI recommenders as it highlights the necessity of security measures to protect memory mechanisms, ensuring recommendation integrity and user trust in increasingly autonomous systems.
Get the Agents Drunk: Memory Perturbations in Autonomous Agent-based Recommender Systems