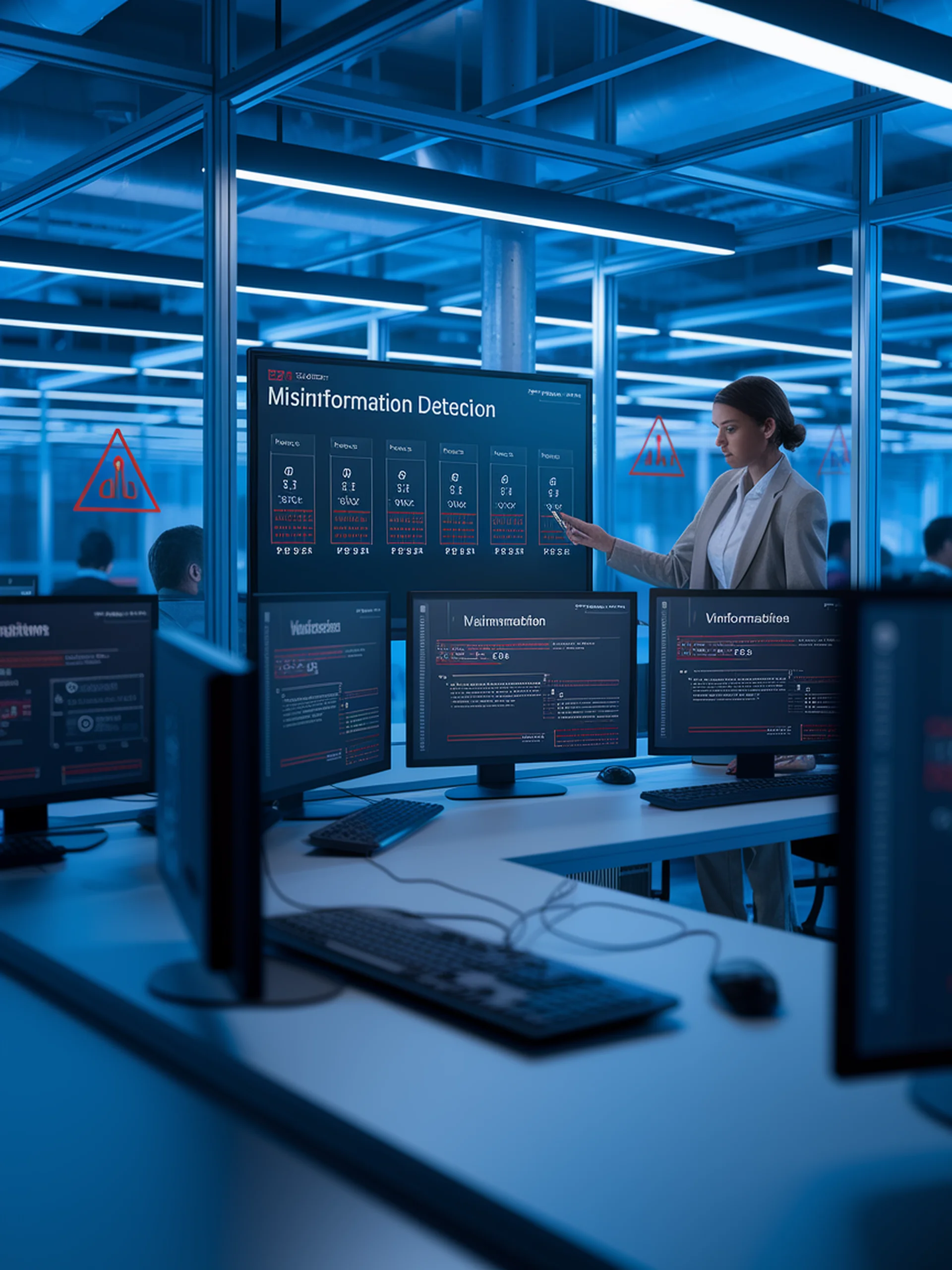
LLMs vs. Misinformation: The Adversarial Factuality Challenge
How open-source LLMs respond to deliberately deceptive prompts
This study evaluates how eight open-source large language models respond to prompts containing deliberate misinformation presented with varying levels of confidence.
- Models struggle most with strongly confident misinformation, highlighting vulnerability to authoritative-sounding falsehoods
- Performance varies significantly across models, with newer architectures showing greater resilience
- When misinformation is presented with limited confidence, models demonstrate improved ability to identify and reject false assertions
- Findings reveal critical security implications for LLM deployment in high-stakes domains
This research provides essential insights for organizations implementing LLMs in environments where misinformation poses security threats, offering a benchmark for model selection and security protocols.